Optimal stratification in outcome prediction using baseline information.
BIOMETRIKA(2016)
摘要
A common practice in predictive medicine is to use current study data to construct a stratification procedure, which groups subjects according to baseline information and forms stratum-specific prevention or intervention strategies. A desirable stratification scheme would not only have small intra-stratum variation but also have a clinically meaningful discriminatory capability. We show how to obtain optimal stratification rules with such desirable properties from fitting a set of regression models relating the outcome to baseline covariates and creating scoring systems for predicting potential outcomes. We propose that all available optimal stratifications be evaluated with an independent dataset to select a final stratification. Lastly, we obtain inferential results for this selected stratification scheme with a holdout dataset. When only one study of moderate size is available, we combine the first two steps via crossvalidation. Extensive simulation studies are used to compare the proposed stratification strategy with alternatives. We illustrate the new proposal using an AIDS clinical trial for binary outcomes and a cardiovascular clinical study for censored event time outcomes.
更多查看译文
关键词
Cox regression model,Crossvalidation,Dynamic programming,Prediction score,Stratified medicine
AI 理解论文
溯源树
样例
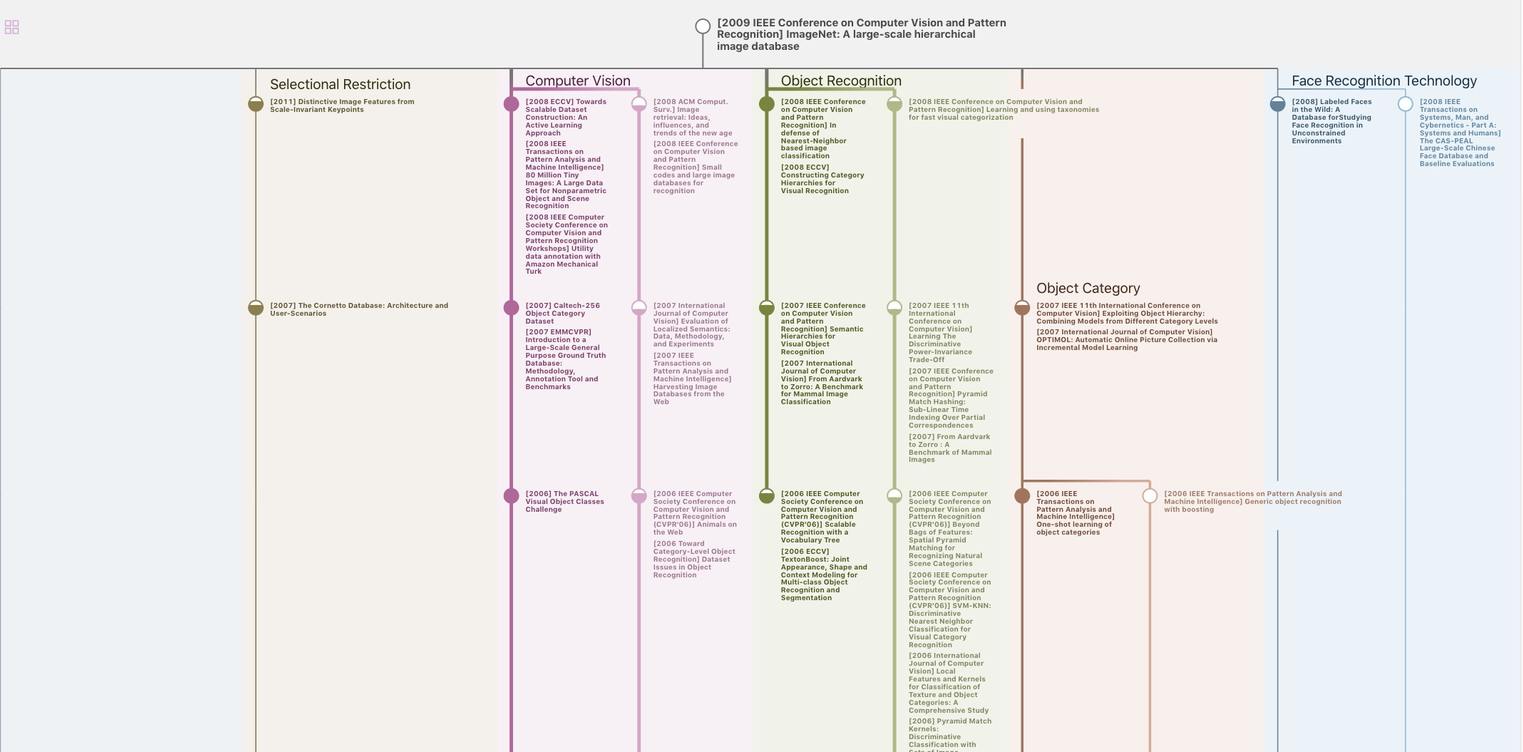
生成溯源树,研究论文发展脉络
Chat Paper
正在生成论文摘要