A mixed-effects, spatially varying coefficients model with application to multi-resolution functional magnetic resonance imaging data.
STATISTICAL METHODS IN MEDICAL RESEARCH(2019)
摘要
Spatial resolution plays an important role in functional magnetic resonance imaging studies as the signal-to-noise ratio increases linearly with voxel volume. In scientific studies, where functional magnetic resonance imaging is widely used, the standard spatial resolution typically used is relatively low which ensures a relatively high signal-to-noise ratio. However, for pre-surgical functional magnetic resonance imaging analysis, where spatial accuracy is paramount, high-resolution functional magnetic resonance imaging may play an important role with its greater spatial resolution. High spatial resolution comes at the cost of a smaller signal-to-noise ratio. This begs the question as to whether we can leverage the higher signal-to-noise ratio of a standard functional magnetic resonance imaging study with the greater spatial accuracy of a high-resolution functional magnetic resonance imaging study in a pre-operative patient. To answer this question, we propose to regress the statistic image from a high resolution scan onto the statistic image obtained from a standard resolution scan using a mixed-effects model with spatially varying coefficients. We evaluate our model via simulation studies and we compare its performance with a recently proposed model that operates at a single spatial resolution. We apply and compare the two models on data from a patient awaiting tumor resection. Both simulation study results and the real data analysis demonstrate that our newly proposed model indeed leverages the larger signal-to-noise ratio of the standard spatial resolution scan while maintaining the advantages of the high spatial resolution scan.
更多查看译文
关键词
Spatially varying coefficients,Bayesian model,functional magnetic resonance imaging,multiresolution
AI 理解论文
溯源树
样例
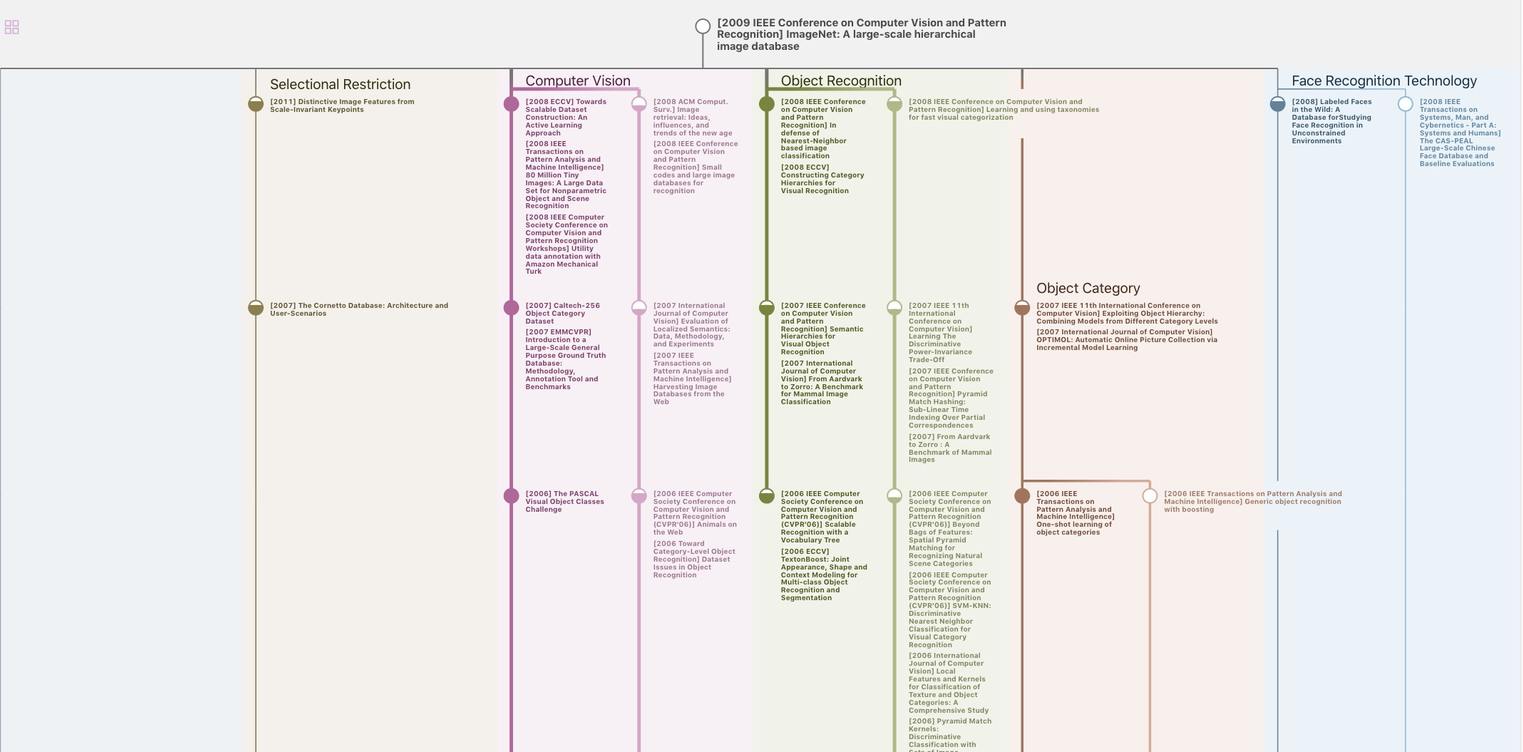
生成溯源树,研究论文发展脉络
Chat Paper
正在生成论文摘要