Characteristics of Real-World Metastatic Non-Small Cell Lung Cancer Patients Treated with Nivolumab and Pembrolizumab During the Year Following Approval.
ONCOLOGIST(2018)
摘要
Background. Evidence from cancer clinical trials can be difficult to generalize to real-world patient populations, but can be complemented by real-world evidence to optimize personalization of care. Further, real-world usage patterns of programmed cell death protein 1 (PD-1) inhibitors following approval can inform future studies of subpopulations underrepresented in clinical trials. Materials and Methods. We performed a multicenter analysis using electronic health record data collected during routine care of patients treated in community cancer care clinics in the Flatiron Health network. Real-world metastatic non-small cell lung cancer (NSCLC) patients who received nivolumab or pembrolizumab in the metastatic setting (n=1,344) were selected from a starting random sample of 55,969 NSCLC patients with two or more documented visits from January 1, 2011, through March 31, 2016. The primary study outcome measurement was demographic and treatment characteristics of the cohort. Results. Median age at PD-1 inhibitor initiation was 69 years (interquartile range 61-75). Patients were 56% male, 88% smokers, 65% nonsquamous histology, and 64% diagnosed at stage IV. Of 1,344 patients, 112 (8%) were tested for programmed death-ligand 1 expression. Overall, 50% received nivolumab or pembrolizumab in the second line, with a substantial proportion of third and later line use that began to decline in Q4 2015. Conclusion. During the year following U.S. regulatory approval of PD-1 inhibitors for treatment of NSCLC, real-world patients receiving nivolumab or pembrolizumab were older at treatment initiation and more had smoking history relative to clinical trial cohorts. Studies of outcomes in underrepresented subgroups are needed to inform real-world treatment decisions.
更多查看译文
关键词
Non-small cell lung cancer,Nivolumab,Pembrolizumab,Demography,Electronic health records
AI 理解论文
溯源树
样例
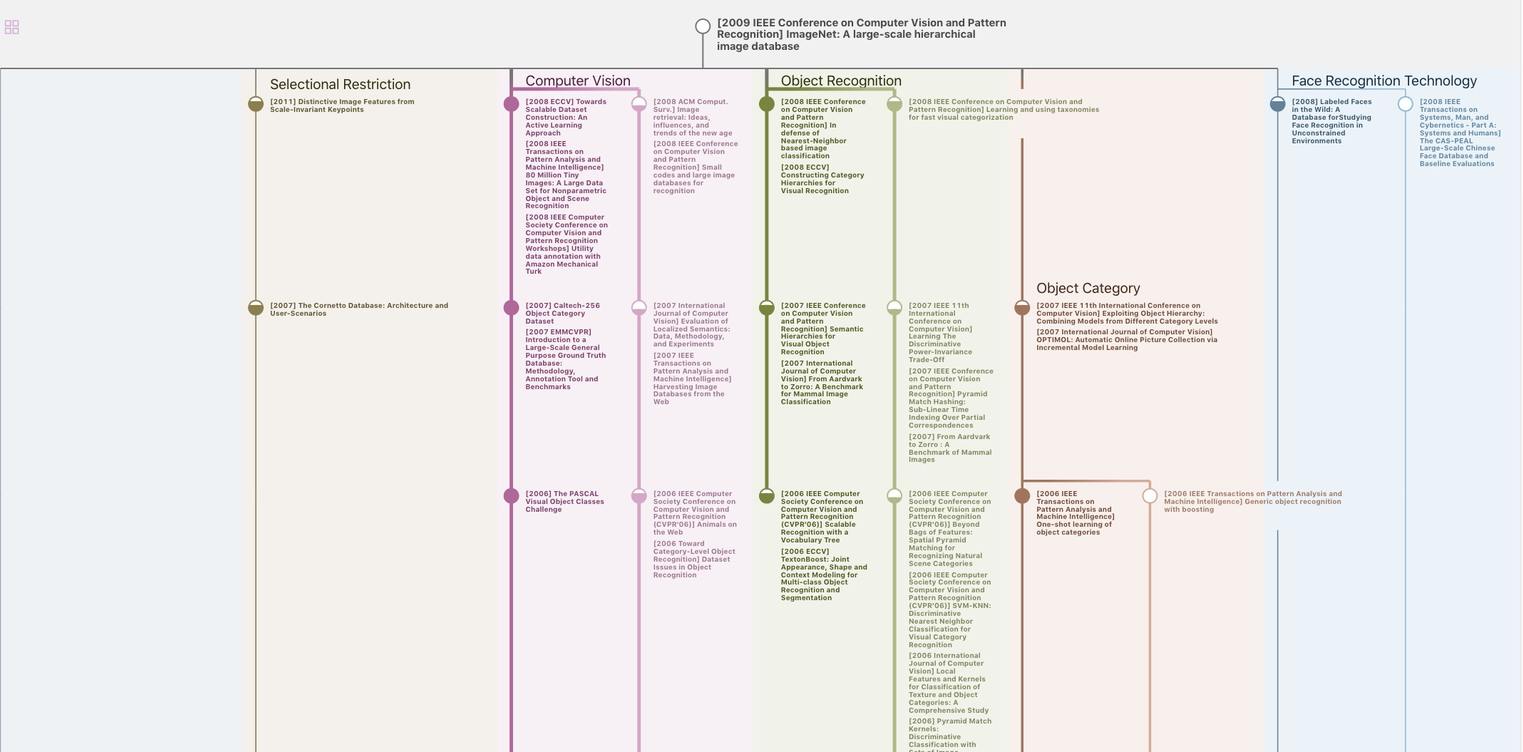
生成溯源树,研究论文发展脉络
Chat Paper
正在生成论文摘要