Using DFT methodology for more reliable predictive models: Design of inhibitors of Golgi α-Mannosidase II
Journal of Molecular Graphics and Modelling(2016)
摘要
Human Golgi α-mannosidase II (GMII), a zinc ion co-factor dependent glycoside hydrolase (E.C.3.2.1.114), is a pharmaceutical target for the design of inhibitors with anti-cancer activity. The discovery of an effective inhibitor is complicated by the fact that all known potent inhibitors of GMII are involved in unwanted co-inhibition with lysosomal α-mannosidase (LMan, E.C.3.2.1.24), a relative to GMII. Routine empirical QSAR models for both GMII and LMan did not work with a required accuracy. Therefore, we have developed a fast computational protocol to build predictive models combining interaction energy descriptors from an empirical docking scoring function (Glide-Schrödinger), Linear Interaction Energy (LIE) method, and quantum mechanical density functional theory (QM-DFT) calculations. The QSAR models were built and validated with a library of structurally diverse GMII and LMan inhibitors and non-active compounds. A critical role of QM-DFT descriptors for the more accurate prediction abilities of the models is demonstrated. The predictive ability of the models was significantly improved when going from the empirical docking scoring function to mixed empirical-QM-DFT QSAR models (Q2=0.78–0.86 when cross-validation procedures were carried out; and R2=0.81–0.83 for a testing set). The average error for the predicted ΔGbind decreased to 0.8–1.1kcalmol−1. Also, 76–80% of non-active compounds were successfully filtered out from GMII and LMan inhibitors. The QSAR models with the fragmented QM-DFT descriptors may find a useful application in structure-based drug design where pure empirical and force field methods reached their limits and where quantum mechanics effects are critical for ligand-receptor interactions. The optimized models will apply in lead optimization processes for GMII drug developments.
更多查看译文
关键词
Structure-based drug design,Linear interaction energy,Density functional theory,Mannosidase,Cancer,Selective inhibition
AI 理解论文
溯源树
样例
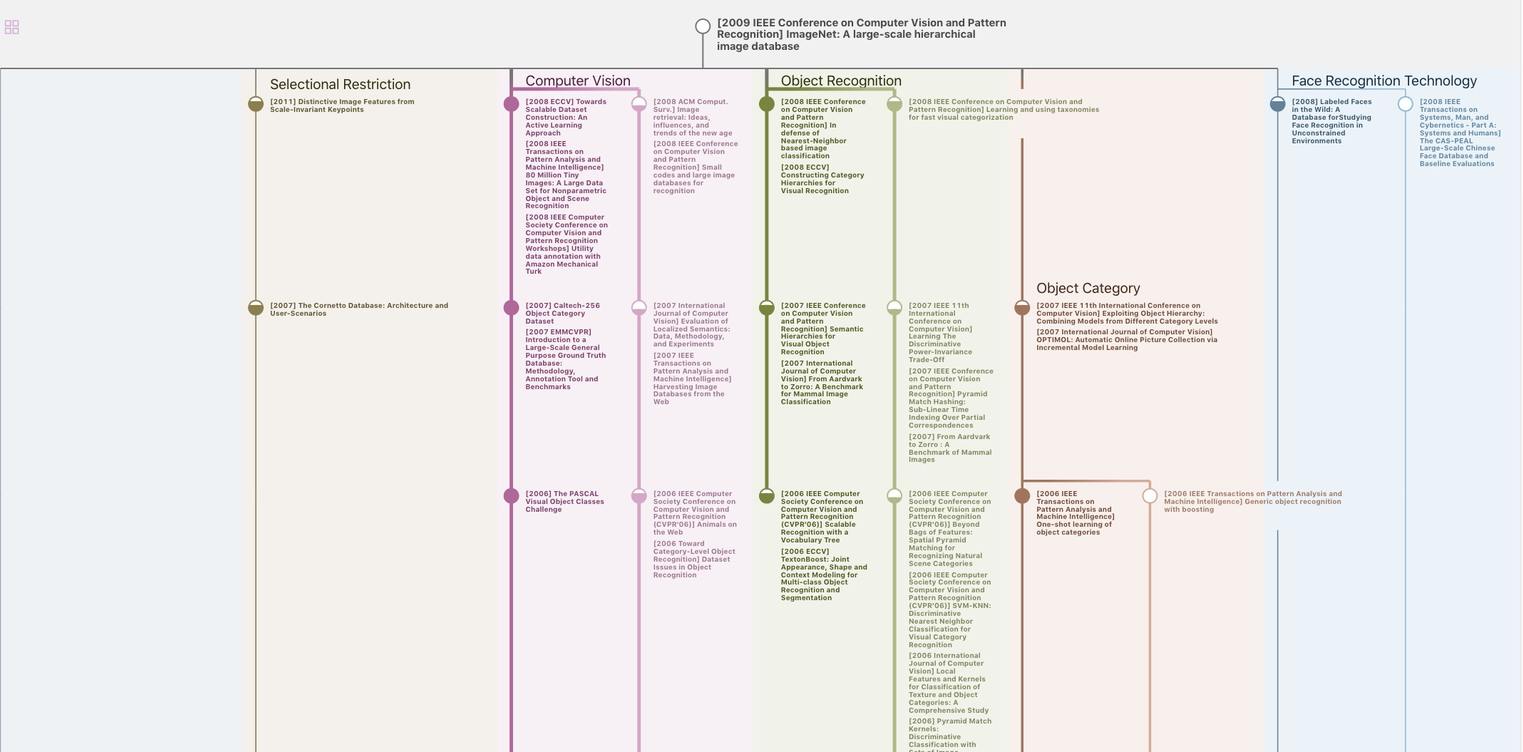
生成溯源树,研究论文发展脉络
Chat Paper
正在生成论文摘要