Distinguishing outcomes from indicators via Bayesian modeling.
PSYCHOLOGICAL METHODS(2017)
摘要
A conceptual distinction is drawn between indicators, which serve to define latent variables, and outcomes, which do not. However, commonly used frequentist and Bayesian estimation procedures do not honor this distinction. They allow the outcomes to influence the latent variables and the measurement model parameters for the indicators, rendering the latent variables subject to interpretational confounding. Modified Bayesian procedures that preclude this are advanced, along with procedures for conducting diagnostic model-data fit analyses. These are studied in a simulation, where they outperform existing strategies, and illustrated with an example. Translational Abstract Latent variables are commonly used to acknowledge and model the presence of measurement error, and are often used to predict outcome variables of interest. A problem arises in the common use of such latent variable models, wherein the outcome variables a researcher is interested in predicting exert unwanted influence on the definition of the latent variables. This study proposes and studies new methods to preclude this unwanted influence, including methods to detect when such an unwanted influence may be present. Through analyses of simulated and real data, the new methods are shown to outperform existing approaches in the situations studied here. The new methods can be implemented in freely available software, and it is argued that researchers should consider using them in situations when they wish to prevent the outcomes from influencing the latent variables that predict them.
更多查看译文
关键词
latent variables models,structural equation modeling,Bayesian methods,cut models,Gibbs sampling
AI 理解论文
溯源树
样例
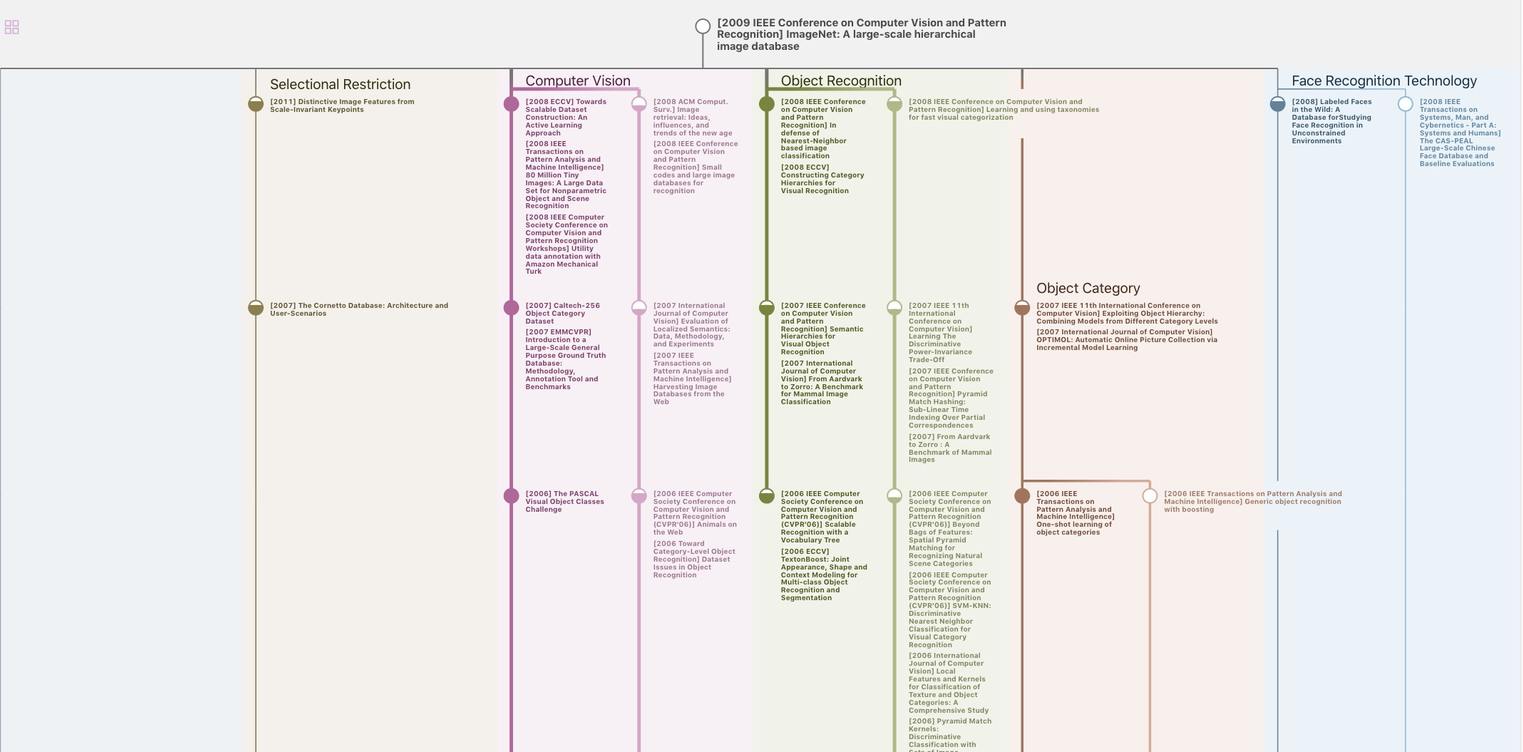
生成溯源树,研究论文发展脉络
Chat Paper
正在生成论文摘要