Attentive Long Short-Term Preference Modeling for Personalized Product Search
ACM Transactions on Information Systems (TOIS)(2018)
摘要
E-commerce users may expect different products even for the same query, due to their diverse personal preferences. It is well-known that there are two types of preferences: long-term ones and short-term ones. The former refers to user' inherent purchasing bias and evolves slowly. By contrast, the latter reflects users' purchasing inclination in a relatively short period. They both affect users' current purchasing intentions. However, few research efforts have been dedicated to jointly model them for the personalized product search. To this end, we propose a novel Attentive Long Short-Term Preference model, dubbed as ALSTP, for personalized product search. Our model adopts the neural networks approach to learn and integrate the long- and short-term user preferences with the current query for the personalized product search. In particular, two attention networks are designed to distinguish which factors in the short-term as well as long-term user preferences are more relevant to the current query. This unique design enables our model to capture users' current search intentions more accurately. Our work is the first to apply attention mechanisms to integrate both long- and short-term user preferences with the given query for the personalized search. Extensive experiments over four Amazon product datasets show that our model significantly outperforms several state-of-the-art product search methods in terms of different evaluation metrics.
更多查看译文
关键词
Personalized product search, attention mechanism, long short-term preference
AI 理解论文
溯源树
样例
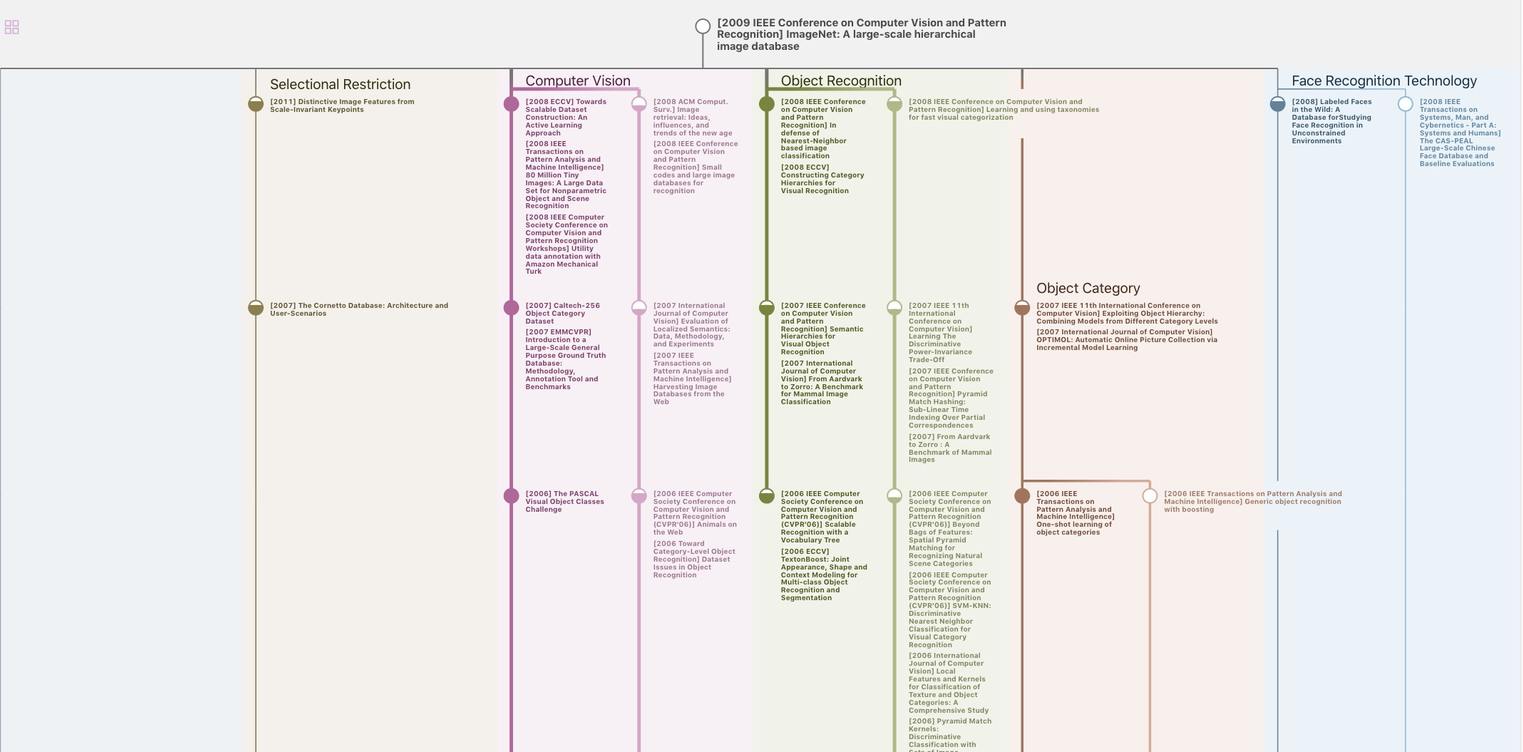
生成溯源树,研究论文发展脉络
Chat Paper
正在生成论文摘要