DSIC: Deep Learning based Self-Interference Cancellation for In-Band Full Duplex Wireless.
arXiv: Networking and Internet Architecture(2018)
摘要
In-band full duplex wireless is of utmost interest to future wireless communication and networking due to great potentials of spectrum efficiency. IBFD wireless, however, is throttled by its key challenge, namely self-interference. Therefore, effective self-interference cancellation is the key to enable IBFD wireless. This paper proposes a real-time non-linear self-interference cancellation solution based on deep learning. In this solution, a self-interference channel is modeled by a deep neural network (DNN). Synchronized self-interference channel data is first collected to train the DNN of the self-interference channel. Afterwards, the trained DNN is used to cancel the self-interference at a wireless node. This solution has been implemented on a USRP SDR testbed and evaluated in real world in multiple scenarios with various modulations in transmitting information including numbers, texts as well as images. It results in the performance of 17dB in digital cancellation, which is very close to the self-interference power and nearly cancels the self-interference at a SDR node in the testbed. The solution yields an average of 8.5% bit error rate (BER) over many scenarios and different modulation schemes.
更多查看译文
AI 理解论文
溯源树
样例
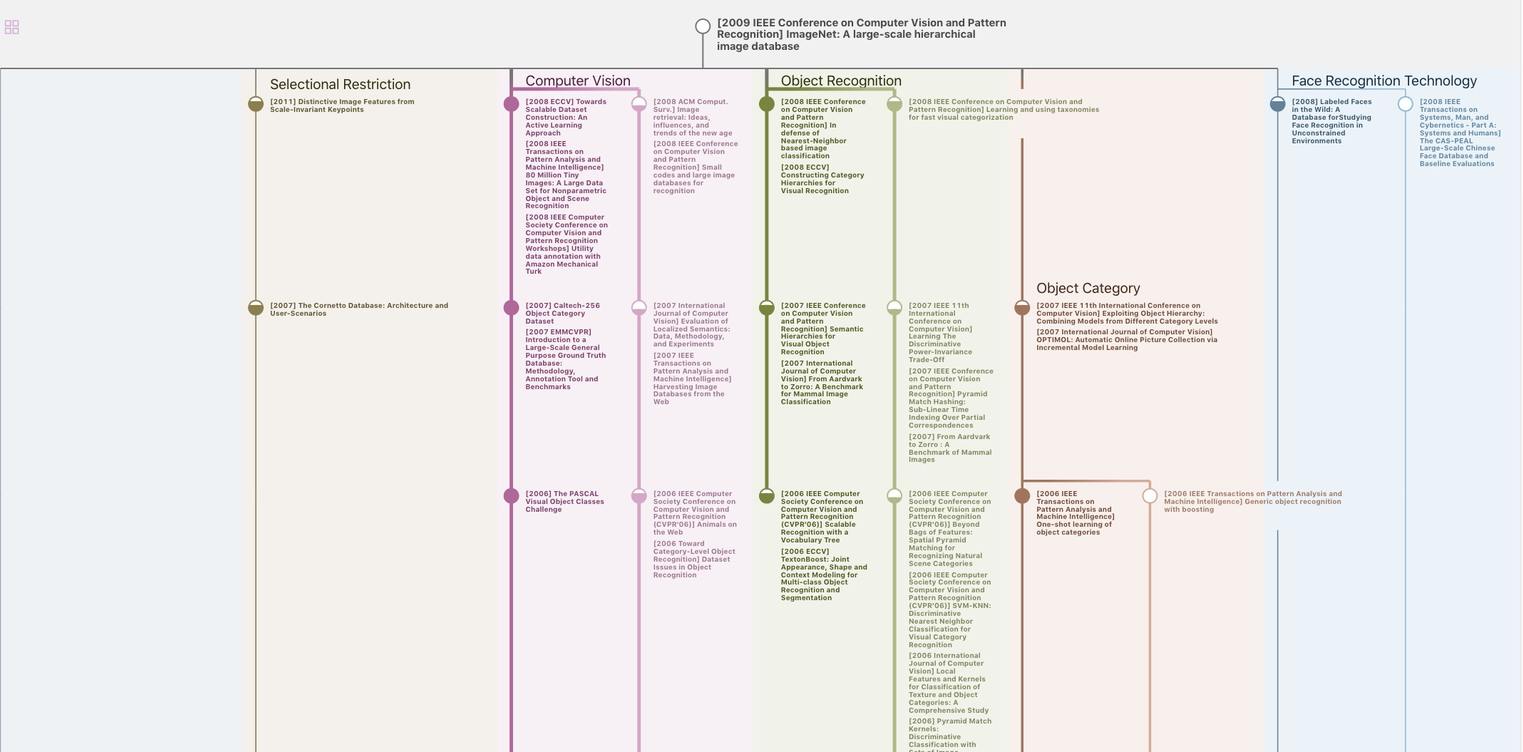
生成溯源树,研究论文发展脉络
Chat Paper
正在生成论文摘要