Visual Entailment Task for Visually-Grounded Language Learning
arXiv (Cornell University)(2019)
摘要
We introduce a new inference task - Visual Entailment (VE) - which differs from traditional Textual Entailment (TE) tasks whereby a premise is defined by an image, rather than a natural language sentence as in TE tasks. A novel dataset SNLI-VE (publicly available at https://github.com/necla-ml/SNLI-VE) is proposed for VE tasks based on the Stanford Natural Language Inference corpus and Flickr30k. We introduce a differentiable architecture called the Explainable Visual Entailment model (EVE) to tackle the VE problem. EVE and several other state-of-the-art visual question answering (VQA) based models are evaluated on the SNLI-VE dataset, facilitating grounded language understanding and providing insights on how modern VQA based models perform.
更多查看译文
关键词
visual entailment task,learning,language,visually-grounded
AI 理解论文
溯源树
样例
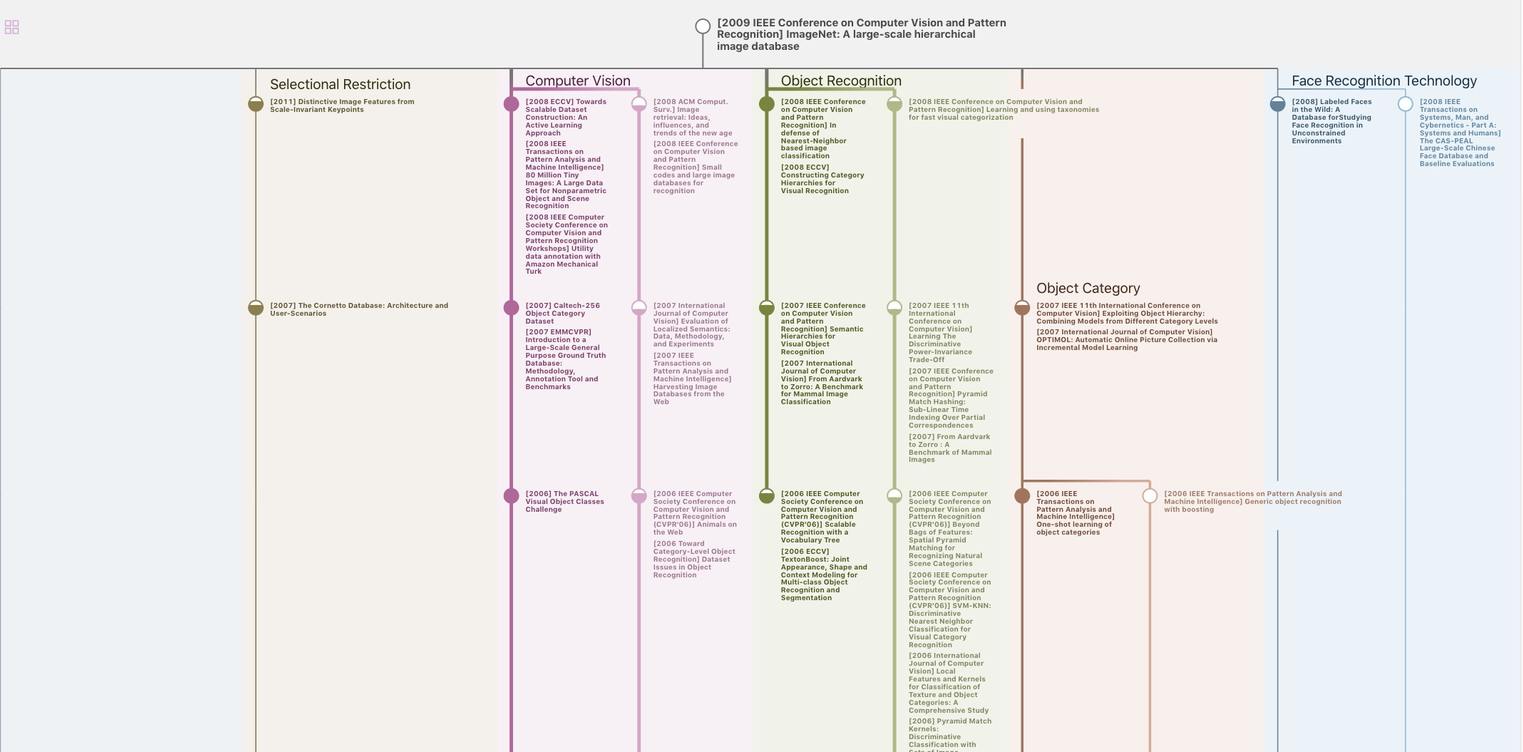
生成溯源树,研究论文发展脉络
Chat Paper
正在生成论文摘要