FineGAN: Unsupervised Hierarchical Disentanglement for Fine-Grained Object Generation and Discovery
2019 IEEE/CVF CONFERENCE ON COMPUTER VISION AND PATTERN RECOGNITION (CVPR 2019)(2018)
摘要
We propose FineGAN, a novel unsupervised GAN framework, which disentangles the background, object shape, and object appearance to hierarchically generate images of fine-grained object categories. To disentangle the factors without supervision, our key idea is to use information theory to associate each factor to a latent code, and to condition the relationships between the codes in a specific way to induce the desired hierarchy. Through extensive experiments, we show that FineGAN achieves the desired disentanglement to generate realistic and diverse images belonging to fine-grained classes of birds, dogs, and cars. Using FineGAN's automatically learned features, we also cluster real images as a first attempt at solving the novel problem of unsupervised fine-grained object category discovery. Our code/models/demo can be found at https://github.com/kkanshul/finegan
更多查看译文
关键词
Recognition: Detection,Categorization,Retrieval,Image and Video Synthesis
AI 理解论文
溯源树
样例
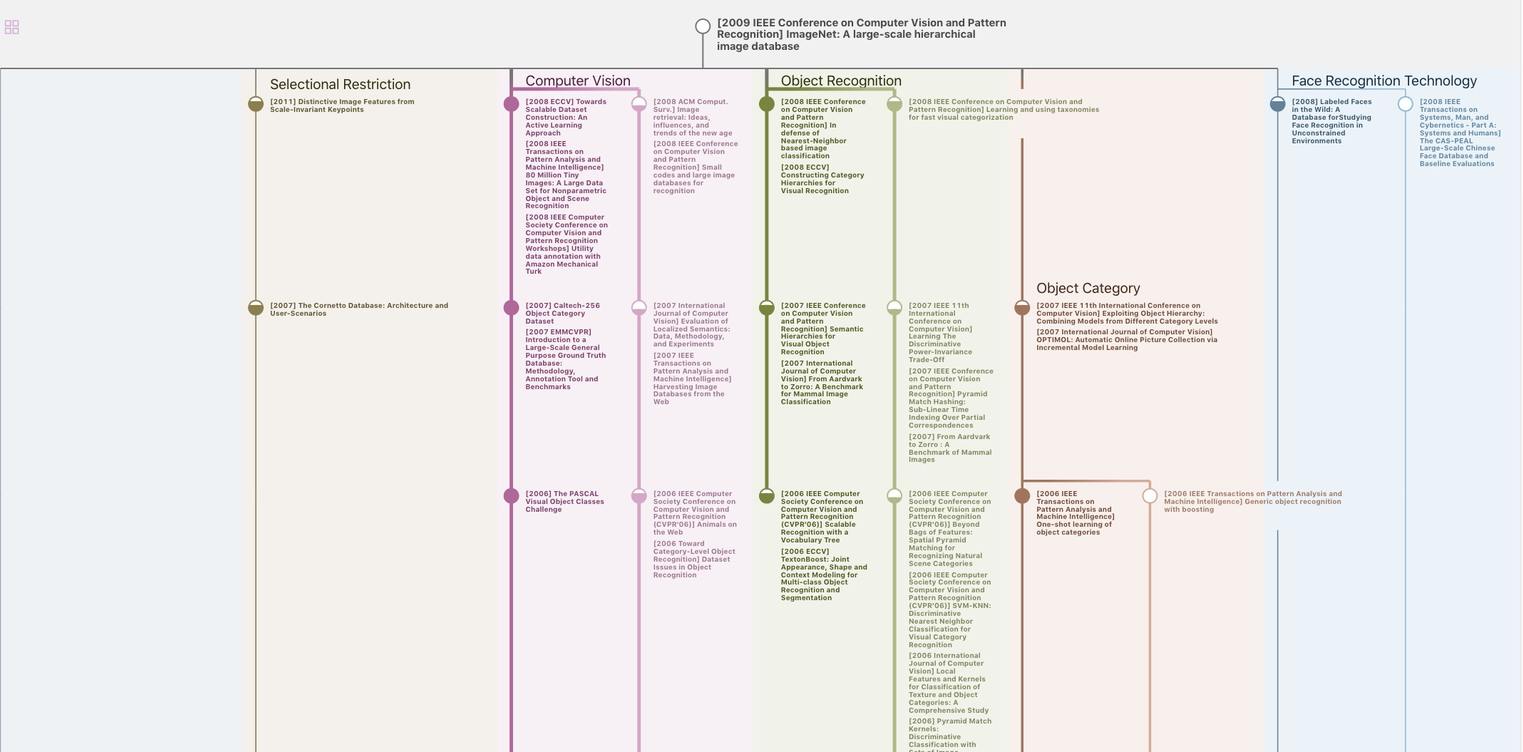
生成溯源树,研究论文发展脉络
Chat Paper
正在生成论文摘要