Learning to Compose Topic-Aware Mixture of Experts for Zero-Shot Video Captioning
AAAI'19/IAAI'19/EAAI'19: Proceedings of the Thirty-Third AAAI Conference on Artificial Intelligence and Thirty-First Innovative Applications of Artificial Intelligence Conference and Ninth AAAI Symposium on Educational Advances in Artificial Intelligence(2018)
摘要
Although promising results have been achieved in video captioning, existing models are limited to the fixed inventory of activities in the training corpus, and do not generalize to open vocabulary scenarios. Here we introduce a novel task, zero-shot video captioning, that aims at describing out-of-domain videos of unseen activities. Videos of different activities usually require different captioning strategies in many aspects, i.e. word selection, semantic construction, and style expression etc, which poses a great challenge to depict novel activities without paired training data. But meanwhile, similar activities share some of those aspects in common. Therefore, We propose a principled Topic-Aware Mixture of Experts (TAMoE) model for zero-shot video captioning, which learns to compose different experts based on different topic embeddings, implicitly transferring the knowledge learned from seen activities to unseen ones. Besides, we leverage external topic-related text corpus to construct the topic embedding for each activity, which embodies the most relevant semantic vectors within the topic. Empirical results not only validate the effectiveness of our method in utilizing semantic knowledge for video captioning, but also show its strong generalization ability when describing novel activities.
更多查看译文
AI 理解论文
溯源树
样例
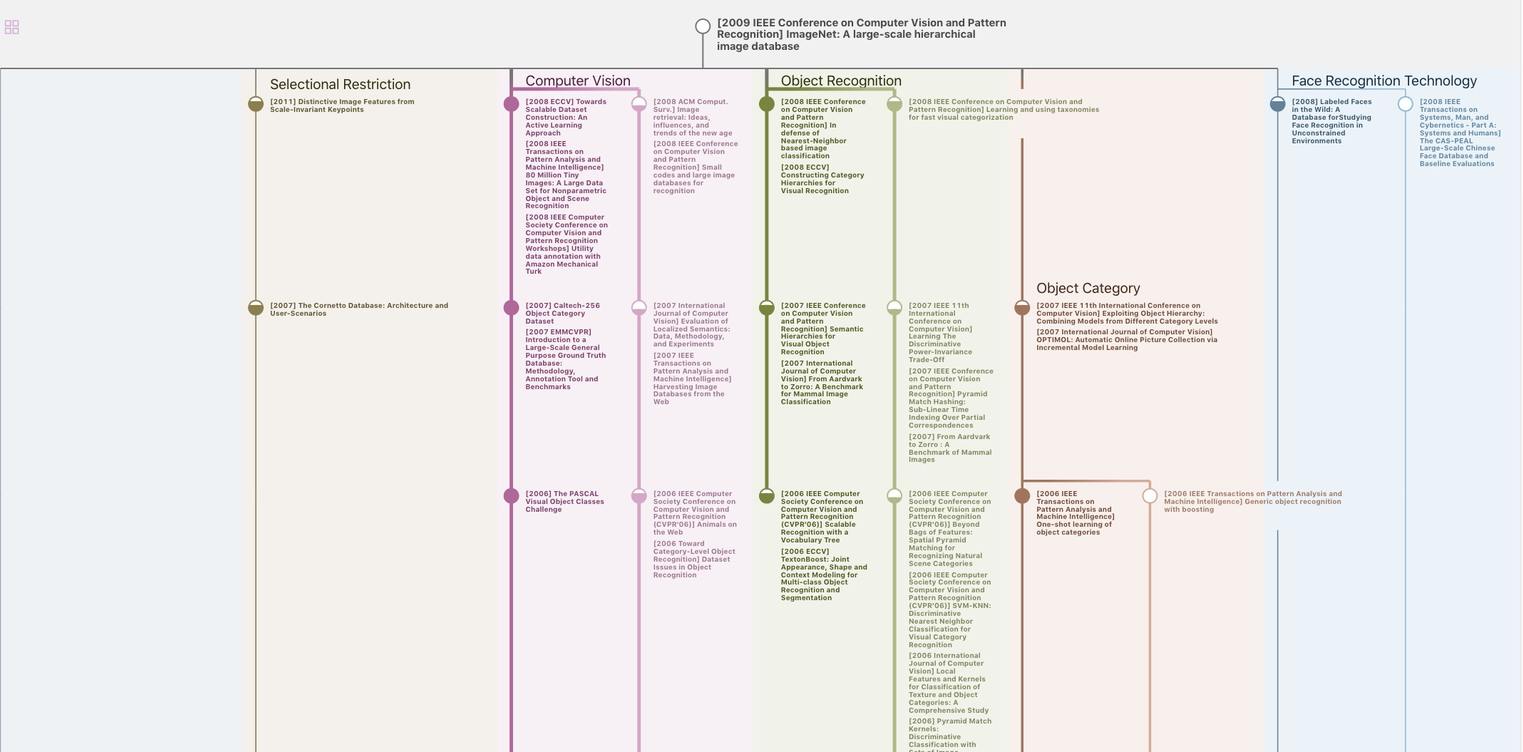
生成溯源树,研究论文发展脉络
Chat Paper
正在生成论文摘要