HSCS: Hierarchical Sparsity Based Co-saliency Detection for RGBD Images.
IEEE Transactions on Multimedia(2019)
摘要
Co-saliency detection aims to discover common and salient objects in an image group containing more than two relevant images. Moreover, depth information has been demonstrated to be effective for many computer vision tasks. In this paper, we propose a novel co-saliency detection method for RGBD images based on hierarchical sparsity reconstruction and energy function refinement. With the assistance of the intrasaliency map, the inter-image correspondence is formulated as a hierarchical sparsity reconstruction framework. The global sparsity reconstruction model with a ranking scheme focuses on capturing the global characteristics among the whole image group through a common foreground dictionary. The pairwise sparsity reconstruction model aims to explore the corresponding relationship between pairwise images through a set of pairwise dictionaries. In order to improve the intra-image smoothness and inter-image consistency, an energy function refinement model is proposed, which includes the unary data term, spatial smooth term, and holistic consistency term. Experiments on two RGBD co-saliency detection benchmarks demonstrate that the proposed method outperforms the state-of-the-art algorithms both qualitatively and quantitatively.
更多查看译文
关键词
Saliency detection,Image reconstruction,Dictionaries,Feature extraction,Task analysis,Computer vision,Computational modeling
AI 理解论文
溯源树
样例
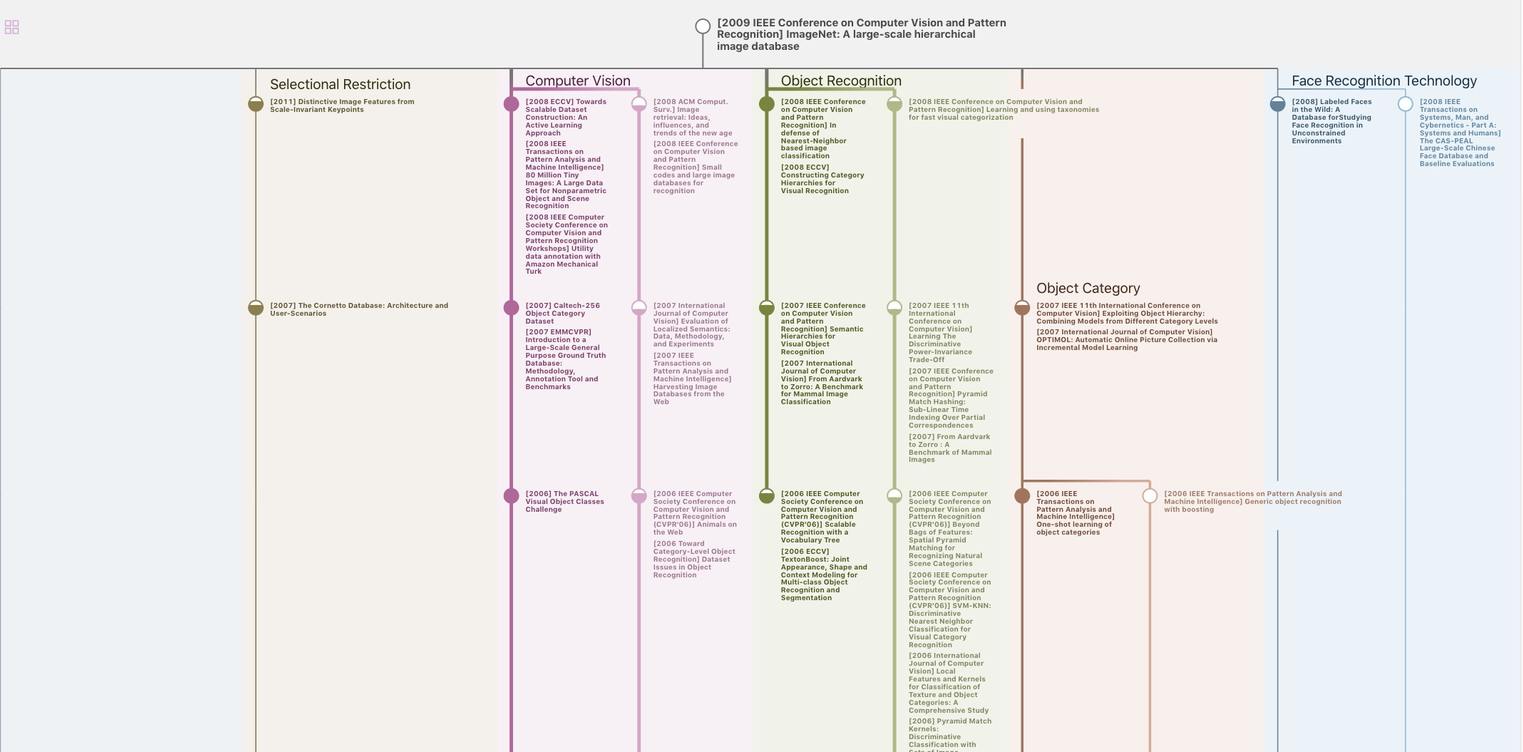
生成溯源树,研究论文发展脉络
Chat Paper
正在生成论文摘要