Weakly Supervised Estimation of Shadow Confidence Maps in Fetal Ultrasound Imaging.
arXiv: Computer Vision and Pattern Recognition(2019)
摘要
Detecting acoustic shadows in ultrasound images is important in many clinical and engineering applications. Real-time feedback of acoustic shadows can guide sonographers to a standardized diagnostic viewing plane with minimal artifacts and can provide additional information for other automatic image analysis algorithms. However, automatically detecting shadow regions using learning-based algorithms is challenging because pixel-wise ground truth annotation of acoustic shadows is subjective and time consuming. In this paper, we propose a weakly supervised method for automatic confidence estimation of acoustic shadow regions. Our method is able to generate a dense shadow-focused confidence map. In our method, a shadow-seg module is built to learn general shadow features for shadow segmentation, based on global image-level annotations as well as a small number of coarse pixel-wise shadow annotations. A transfer function is introduced to extend the obtained binary shadow segmentation to a reference confidence map. In addition, a confidence estimation network is proposed to learn the mapping between input images and the reference confidence maps. This network is able to predict shadow confidence maps directly from input images during inference. We use evaluation metrics such as DICE, inter-class correlation, and so on, to verify the effectiveness of our method. Our method is more consistent than human annotation and outperforms the state-of-the-art quantitatively in shadow segmentation and qualitatively in confidence estimation of shadow regions. Furthermore, we demonstrate the applicability of our method by integrating shadow confidence maps into tasks such as ultrasound image classification, multi-view image fusion, and automated biometric measurements.
更多查看译文
关键词
Acoustics,Image segmentation,Estimation,Image analysis,Task analysis,Biomedical imaging
AI 理解论文
溯源树
样例
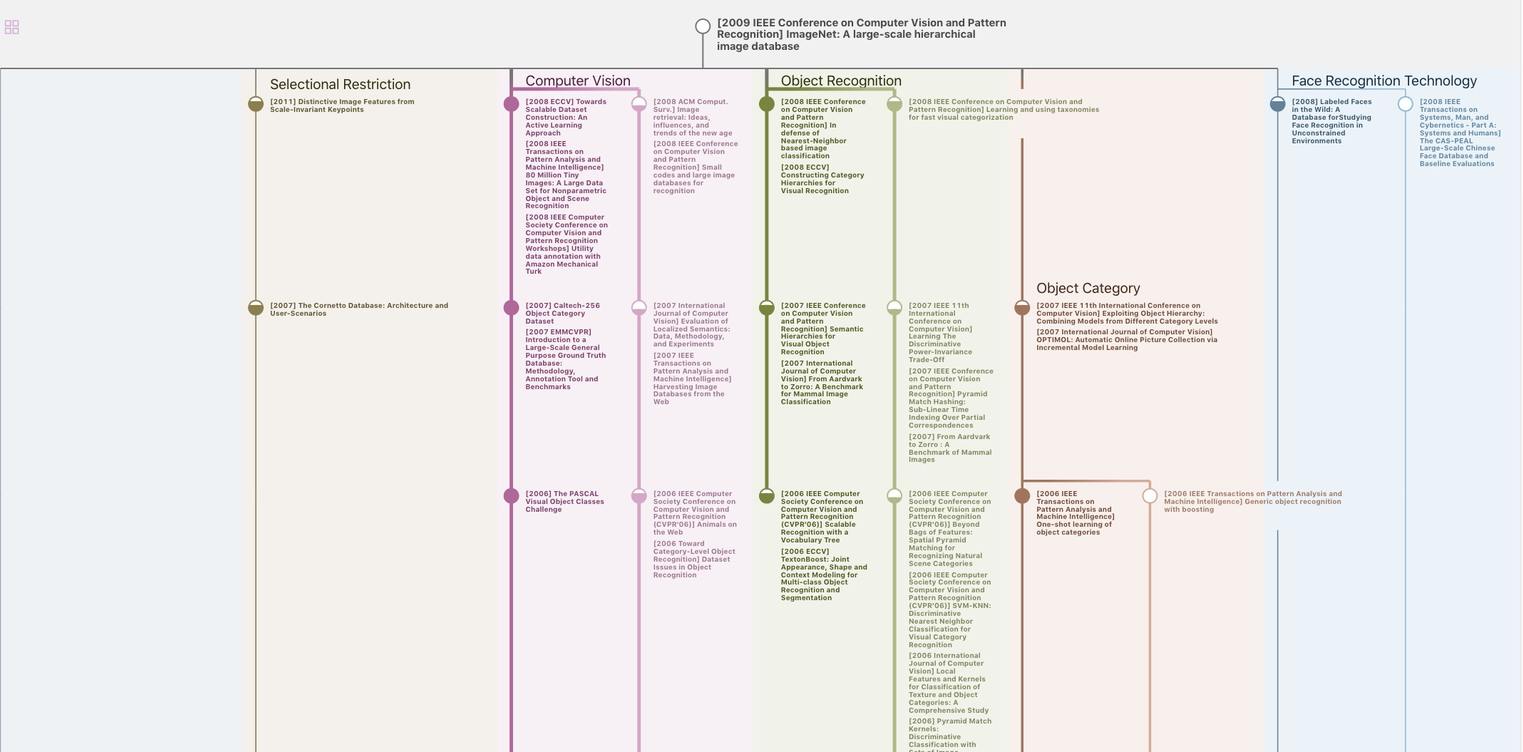
生成溯源树,研究论文发展脉络
Chat Paper
正在生成论文摘要