Applying temporal dependence to detect changes in streaming data
Appl. Intell.(2018)
摘要
Detection of changes in streaming data is an important mining task, with a wide range of real-life applications. Numerous algorithms have been proposed to efficiently detect changes in streaming data. However, the limitation of existing algorithms is that they assume that data are generated independently. In particular, temporal dependencies of data in a stream are still not thoroughly studied. Motivated by this, in this work we propose a new efficient method to detect changes in streaming data by exploring the temporal dependencies of data in the stream. As part of this, we introduce a new statistical model called the Candidate Change Point (CCP) model, with which the main idea is to compute the probabilities of finding change points in the stream. The computed probabilities are used to generate a distribution, which is, in turn, used in statistical hypothesis tests to determine the candidate changes. We use the CCP model to develop a new algorithm called Candidate Change Point Detector (CCPD), which detects change points in linear time, and is thus applicable for real-time applications. Our extensive experimental evaluation demonstrates the efficiency and the feasibility of our approach.
更多查看译文
关键词
Data streams,Change detection,Temporal dependence,Adaptive estimation
AI 理解论文
溯源树
样例
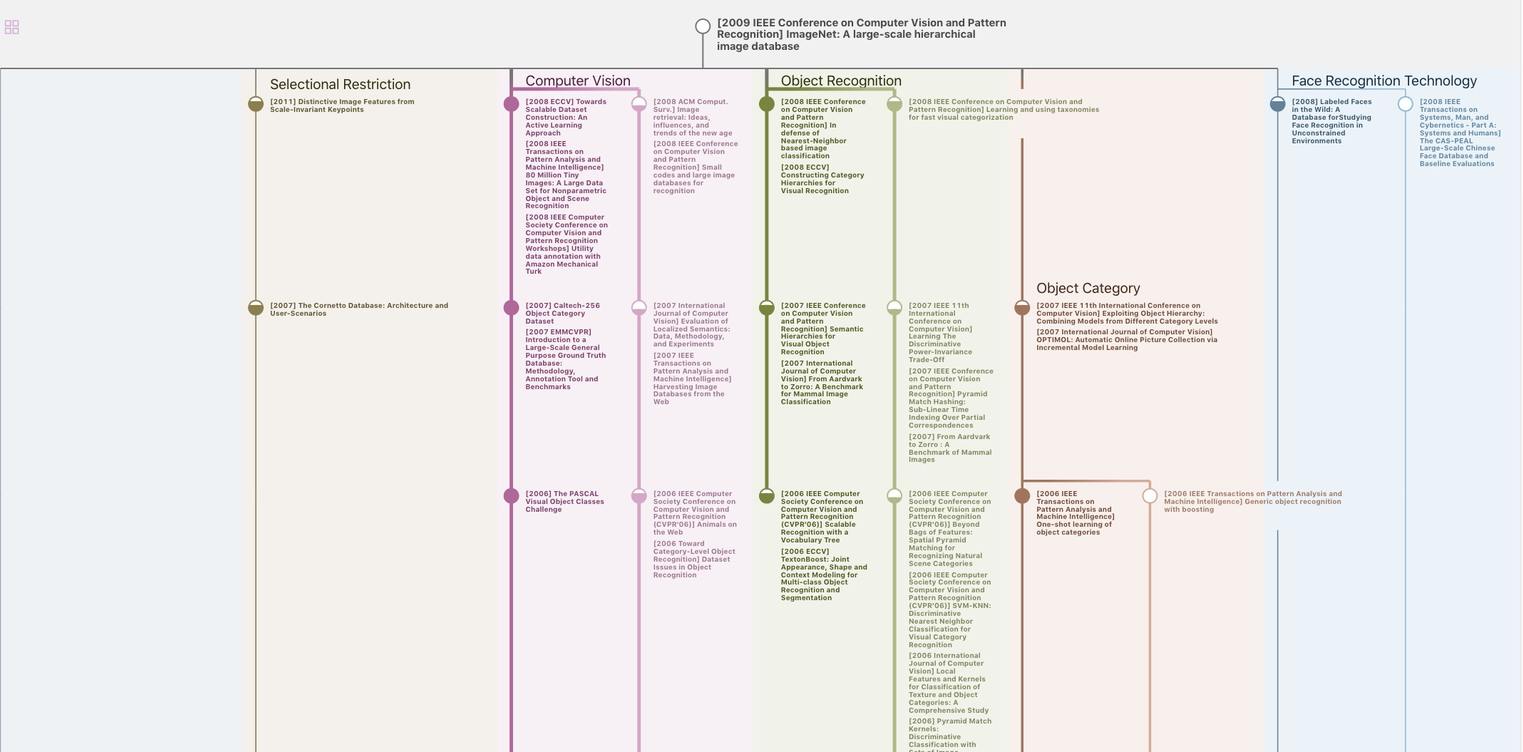
生成溯源树,研究论文发展脉络
Chat Paper
正在生成论文摘要