Total Belief Theorem And Conditional Belief Functions
INTERNATIONAL JOURNAL OF INTELLIGENT SYSTEMS(2018)
摘要
In this paper, new theoretical results for reasoning with belief functions are obtained and discussed. After a judicious decomposition of the set of focal elements of a belief function, we establish the total belief theorem (TBT). which is the direct generalization of the total probability theorem when working in the framework of belief functions. The TBT is also generalized for dealing with different frames of discernments thanks to Cartesian product space. From TBT, we can derive and define formally the expressions of conditional belief functions, which are consistent with the bounds of imprecise conditional probability. This work provides a direct establishment and solid justification of Fagin-Halpern belief conditioning formulas. The well-known Bayes' theorem of probability theory is then generalized in the framework of belief functions, and we illustrate it with an example at the end of this paper.
更多查看译文
AI 理解论文
溯源树
样例
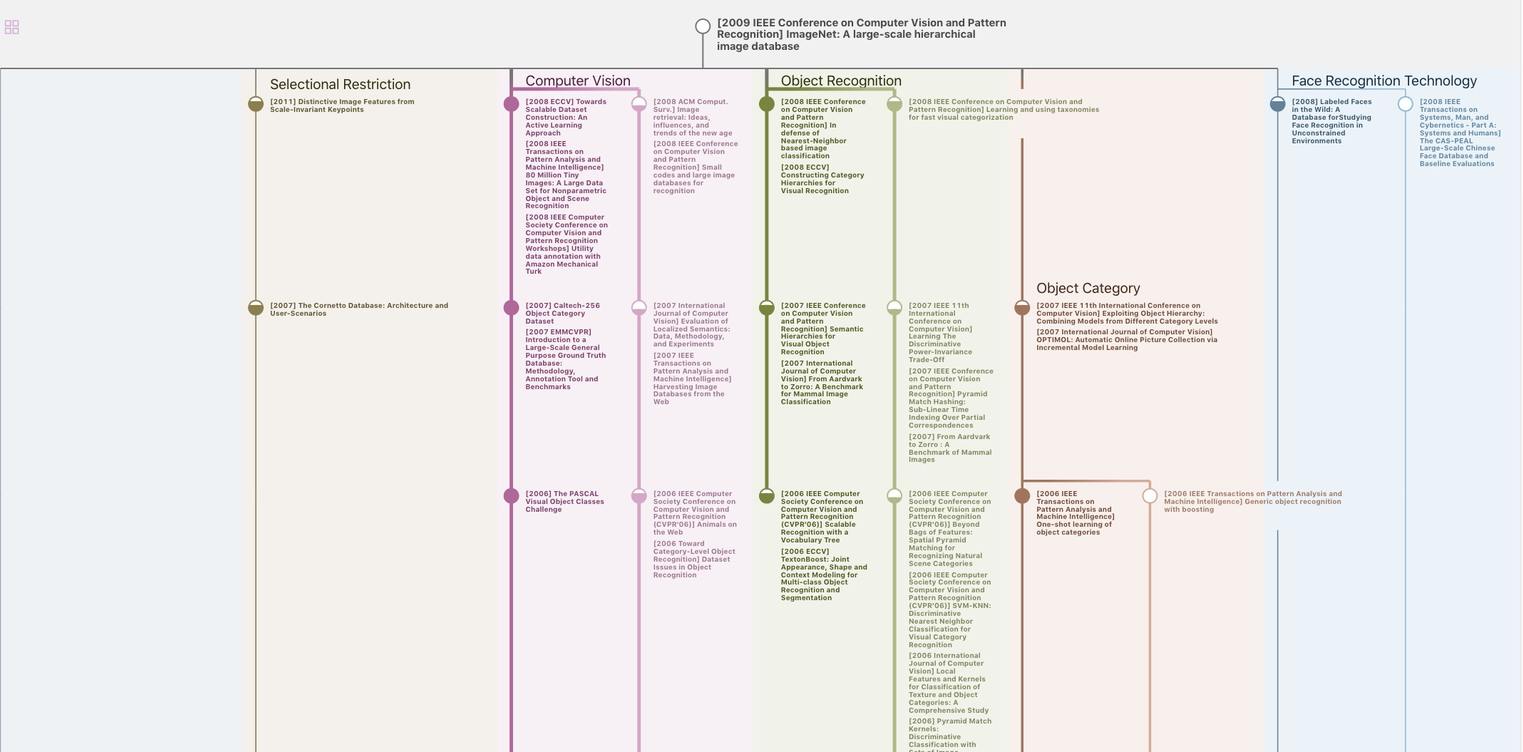
生成溯源树,研究论文发展脉络
Chat Paper
正在生成论文摘要