TPM: A Temporal Personalized Model for Spatial Item Recommendation.
ACM TIST(2018)
摘要
With the rapid development of location-based social networks (LBSNs), spatial item recommendation has become an important way of helping users discover interesting locations to increase their engagement with location-based services. The availability of spatial, temporal, and social information in LBSNs offers an unprecedented opportunity to enhance the spatial item recommendation. Many previous works studied spatial and social influences on spatial item recommendation in LBSNs. Due to the strong correlations between a user’s check-in time and the corresponding check-in location, which include the sequential influence and temporal cyclic effect, it is essential for spatial item recommender system to exploit the temporal effect to improve the recommendation accuracy. Leveraging temporal information in spatial item recommendation is, however, very challenging, considering (1) when integrating sequential influences, users’ check-in data in LBSNs has a low sampling rate in both space and time, which renders existing location prediction techniques on GPS trajectories ineffective, and the prediction space is extremely large, with millions of distinct locations as the next prediction target, which impedes the application of classical Markov chain models; (2) there are various temporal cyclic patterns (i.e., daily, weekly, and monthly) in LBSNs, but existing work is limited to one specific pattern; and (3) there is no existing framework that unifies users’ personal interests, temporal cyclic patterns, and the sequential influence of recently visited locations in a principled manner.
In light of the above challenges, we propose a Temporal Personalized Model (TPM), which introduces a novel latent variable topic-region to model and fuse sequential influence, cyclic patterns with personal interests in the latent and exponential space. The advantages of modeling the temporal effect at the topic-region level include a significantly reduced prediction space, an effective alleviation of data sparsity, and a direct expression of the semantic meaning of users’ spatial activities. Moreover, we introduce two methods to model the effect of various cyclic patterns. The first method is a time indexing scheme that encodes the effect of various cyclic patterns into a binary code. However, the indexing scheme faces the data sparsity problem in each time slice. To deal with this data sparsity problem, the second method slices the time according to each cyclic pattern separately and explores these patterns in a joint additive model.
Furthermore, we design an asymmetric Locality Sensitive Hashing (ALSH) technique to speed up the online top-k recommendation process by extending the traditional LSH. We evaluate the performance of TPM on two real datasets and one large-scale synthetic dataset. The performance of TPM in recommending cold-start items is also evaluated. The results demonstrate a significant improvement in TPM’s ability to recommend spatial items, in terms of both effectiveness and efficiency, compared with the state-of-the-art methods.
更多查看译文
关键词
POI, location-based service, online learning, spatial-temporal modeling
AI 理解论文
溯源树
样例
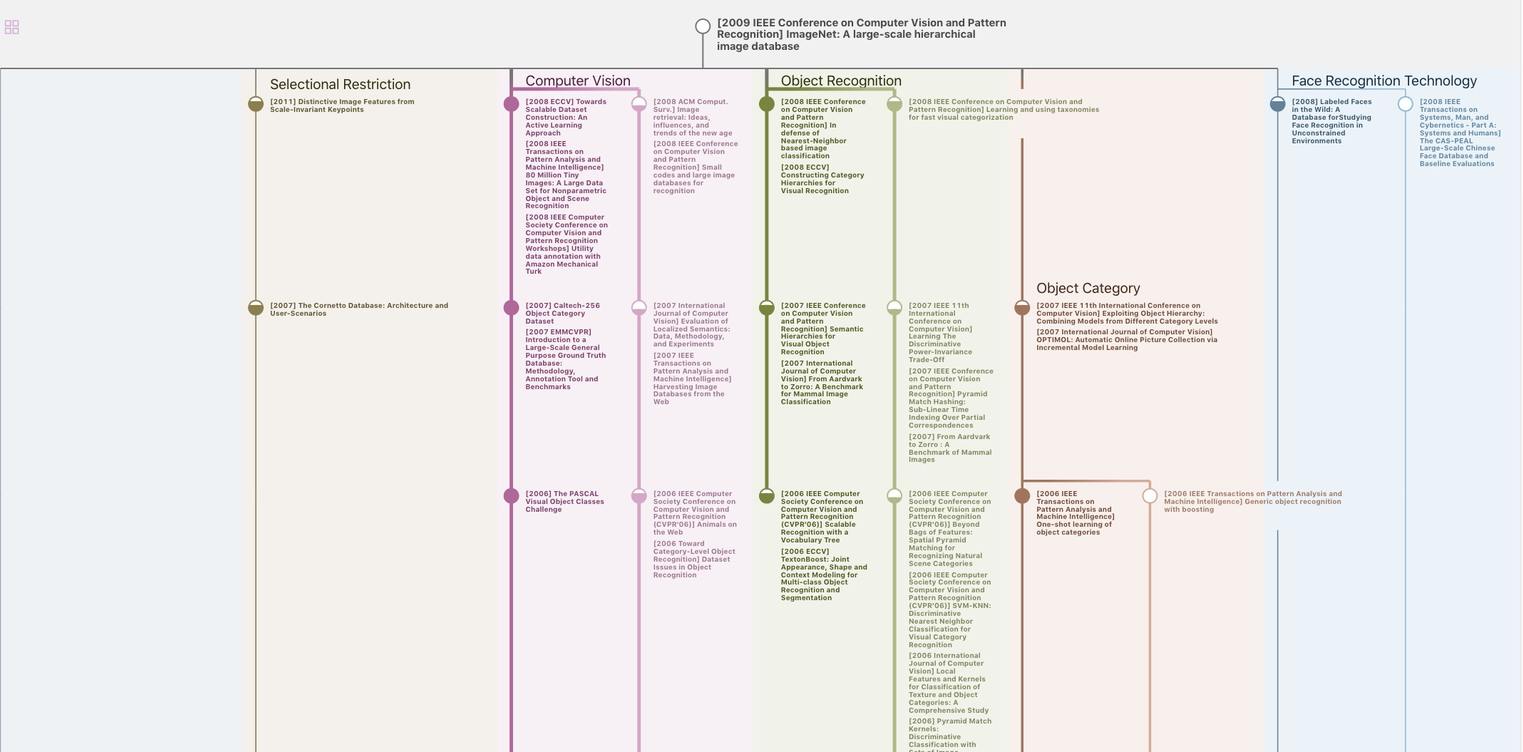
生成溯源树,研究论文发展脉络
Chat Paper
正在生成论文摘要