Multi-Attribute Missing Data Reconstruction Based On Adaptive Weighted Nuclear Norm Minimization In Iot
IEEE ACCESS(2018)
摘要
In the Internet of Things, data sets gathered from sensor nodes are missing a significant fraction of data, owing to noise, collision, unreliable links, and unexpected damage. This phenomenon is more serious in some scenarios, which limits the applications of sensor data. It is therefore necessary to develop methods to reconstruct these lost data with high accuracy. In this paper, a new multi-attribute missing data reconstruction method based on adaptive weighted nuclear norm minimization is developed, and a K-means clustering analysis is embedded into this forecasting process to improve the prediction accuracy. First, to ensure that sensors in one group have similar patterns of measurement, we use a traditional machine learning algorithm, called K-means clustering algorithm to separate sensors into different groups. Second, considering the correlations among multiple attributes of sensor data and its joint low-rank characteristics, we propose an algorithm based on the matrix rank-minimization method of automatic weighted nuclear norm minimization, which adaptively assigns different weights to each singular value simultaneously. Moreover, we use the alternating direction method of multipliers to obtain its optimal solution. Finally, we evaluate the proposed method by using a real sensor data set from the Intel Berkeley Research Laboratory with two missing patterns, namely, random missing pattern and consecutive missing pattern. The simulation results prove that our algorithm performs well even with a small number of samples, and it can propagate through a structure to fill in large missing regions.
更多查看译文
关键词
Data reconstruction, Internet of Things (IoT), K-means, multi-attribute, tensor completion, weighted nuclear norm minimization (WNNM)
AI 理解论文
溯源树
样例
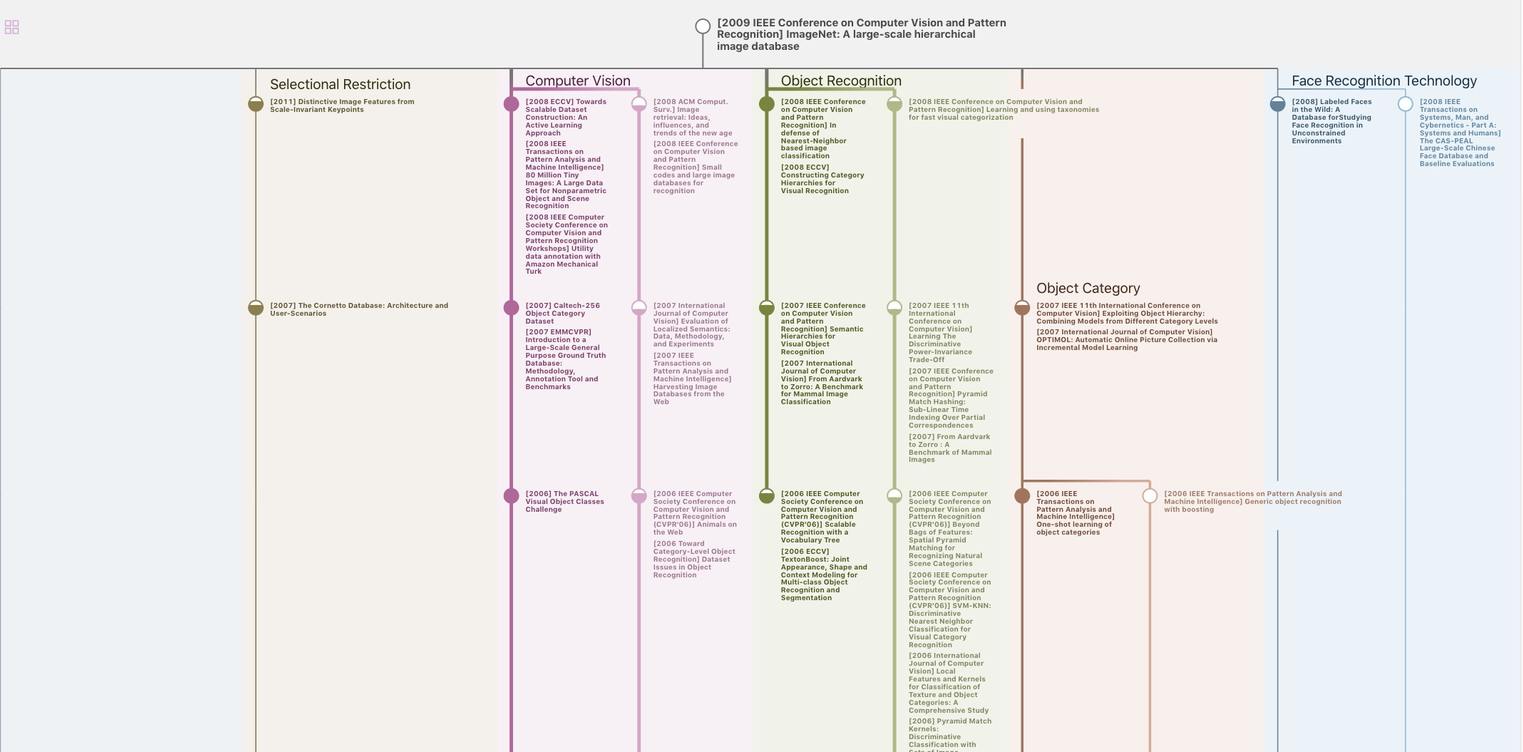
生成溯源树,研究论文发展脉络
Chat Paper
正在生成论文摘要