Multiview 3D human pose estimation using improved least-squares and LSTM networks.
Neurocomputing(2019)
摘要
In this paper we present a deep learning based method to estimate the human pose in 3D when multiple 2D views are available. Our system is composed of a cascade of specialized systems. Firstly, 2D poses are obtained using a deep neural network for the detection of skeleton keypoints in each available view. Then, the 3D coordinates of each keypoint are reconstructed with our proposed least squares optimization method, that analyzes the quality of the 2D detections to decide either to consider or reject them. Once the 3D poses are obtained for each time step, full body pose estimation is performed with a long short-term memory (LSTM) neural network, that takes advantage of the process history to refine the final pose estimation. We provide evidence of the suitability of our contributions in an extensive experimental study. Finally, we were able to prove experimentally that our method obtains competitive results when it is compared to recent representative works in the literature.
更多查看译文
关键词
3D full-body estimation,Deep learning,Long short-term memory,Least squares optimization
AI 理解论文
溯源树
样例
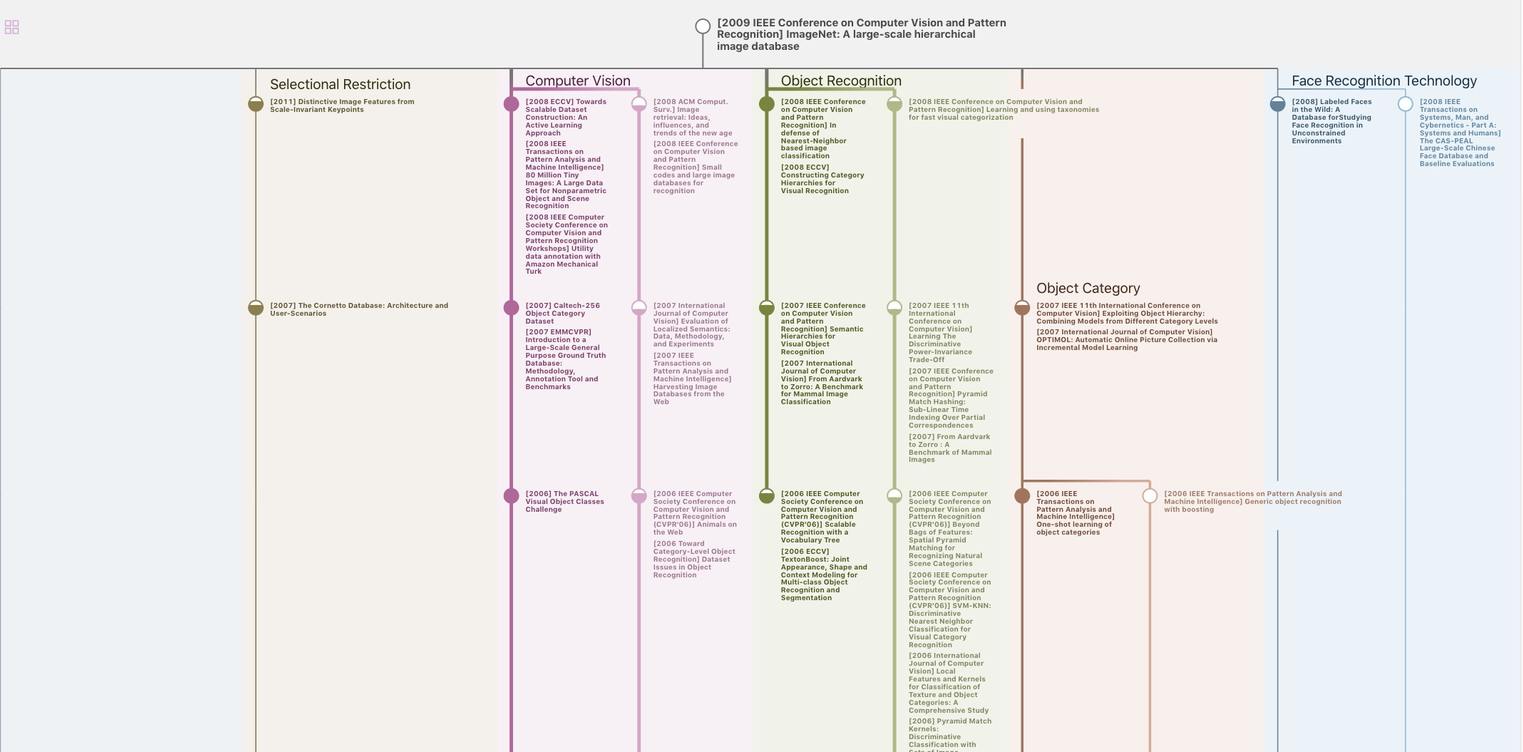
生成溯源树,研究论文发展脉络
Chat Paper
正在生成论文摘要