Moving object detection via segmentation and saliency constrained RPCA.
Neurocomputing(2019)
摘要
Robust Principal Component Analysis (RPCA) is inherently a promising approach for detecting the moving objects in video frames: Under certain conditions, the low-rank and sparse components in RPCA can identify the background and moving objects, respectively. However, it is arguable that RPCA cannot work well without the following two assumptions: (1) The background is static or quasi-static and (2) the moving of the foreground objects is not slow. These two assumptions, unfortunately, are often invalid in reality. To overcome the drawbacks of RPCA, we propose in this work a novel method termed Segmentation and Saliency constrained RPCA (SSC-RPCA), the solution of which is regularized by both segmentation and saliency constraints. In general, SSC-RPCA can effectively overcome the two aforementioned flaws: The segmentation constraint gives SSC-RPCA the ability to cope with the dynamic background, while the saliency constraint makes SSC-RPCA be able to detect the objects moving slowly. Experiments on 14 videos of CDCNET 2014 show the superior performance of SSC-RPCA.
更多查看译文
关键词
Moving object detection,Slow motion,Dynamic background,Video segmentation,Saliency constraint,RPCA
AI 理解论文
溯源树
样例
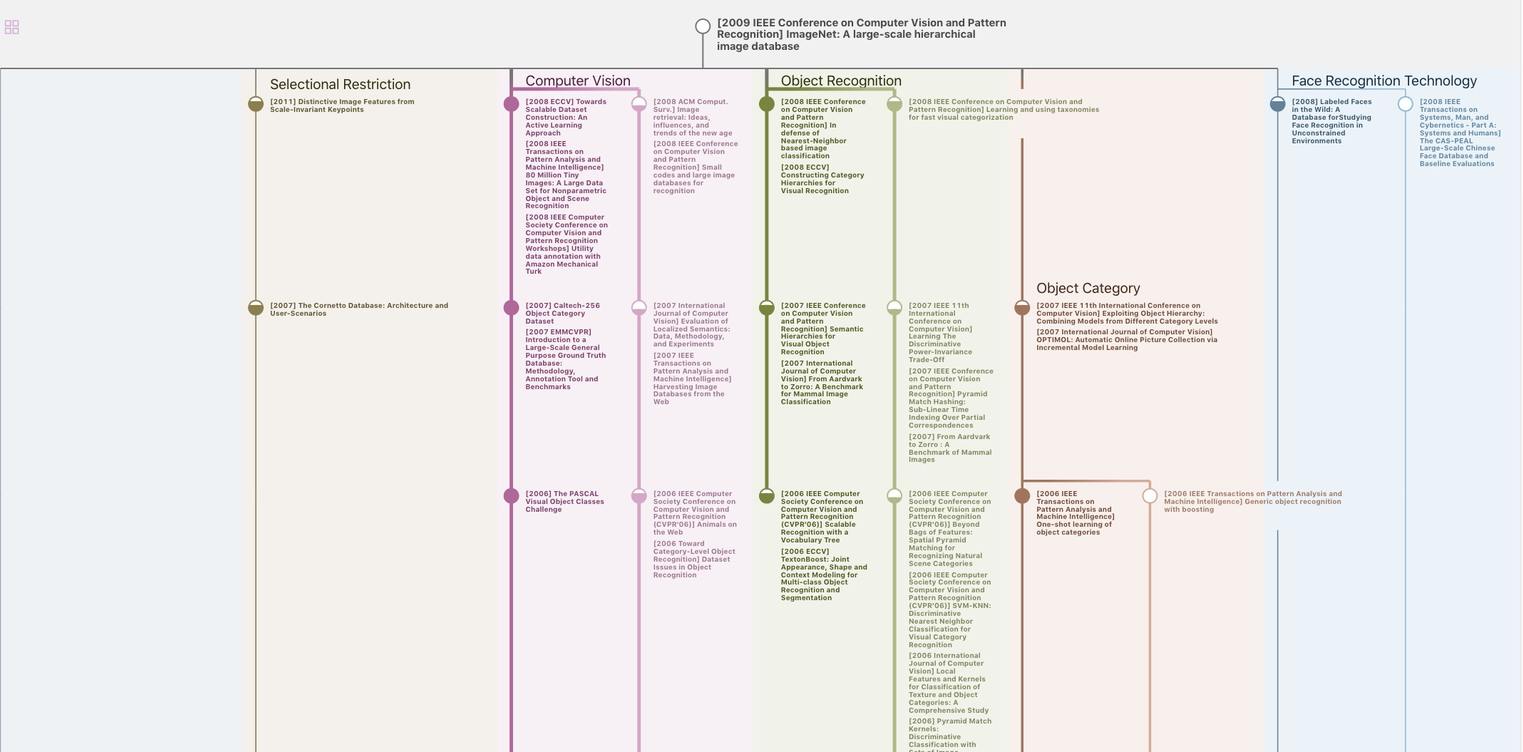
生成溯源树,研究论文发展脉络
Chat Paper
正在生成论文摘要