Improved generative adversarial networks with reconstruction loss.
Neurocomputing(2019)
摘要
We propose a simple regularization scheme to handle the problem of mode missing and unstable training in the generative adversarial networks (GAN). The key idea is to utilize the visual features learned by the discriminator. We reconstruct the real data by feeding the generator with the real data features extracted by the discriminator. A reconstruction loss is added to the GAN’s objective function to enforce the generator can reconstruct from the features of the discriminator, which helps to explicitly guide the generator towards to near the probable configurations of real data. The proposed reconstruction loss improves the performance of GAN, produces higher quality images on different dataset, and can be easily combined with other regularization loss functions such as gradient penalty to improve the performance of various GANs. We conducted experiments on the widespread adopted architecture DCGAN and the complicated ResNet architecture across different datasets, the results of which show the effectiveness and robustness of our proposed method.
更多查看译文
关键词
Generative adversarial networks (GAN),Image generation,Reconstruction loss,Deep generative model
AI 理解论文
溯源树
样例
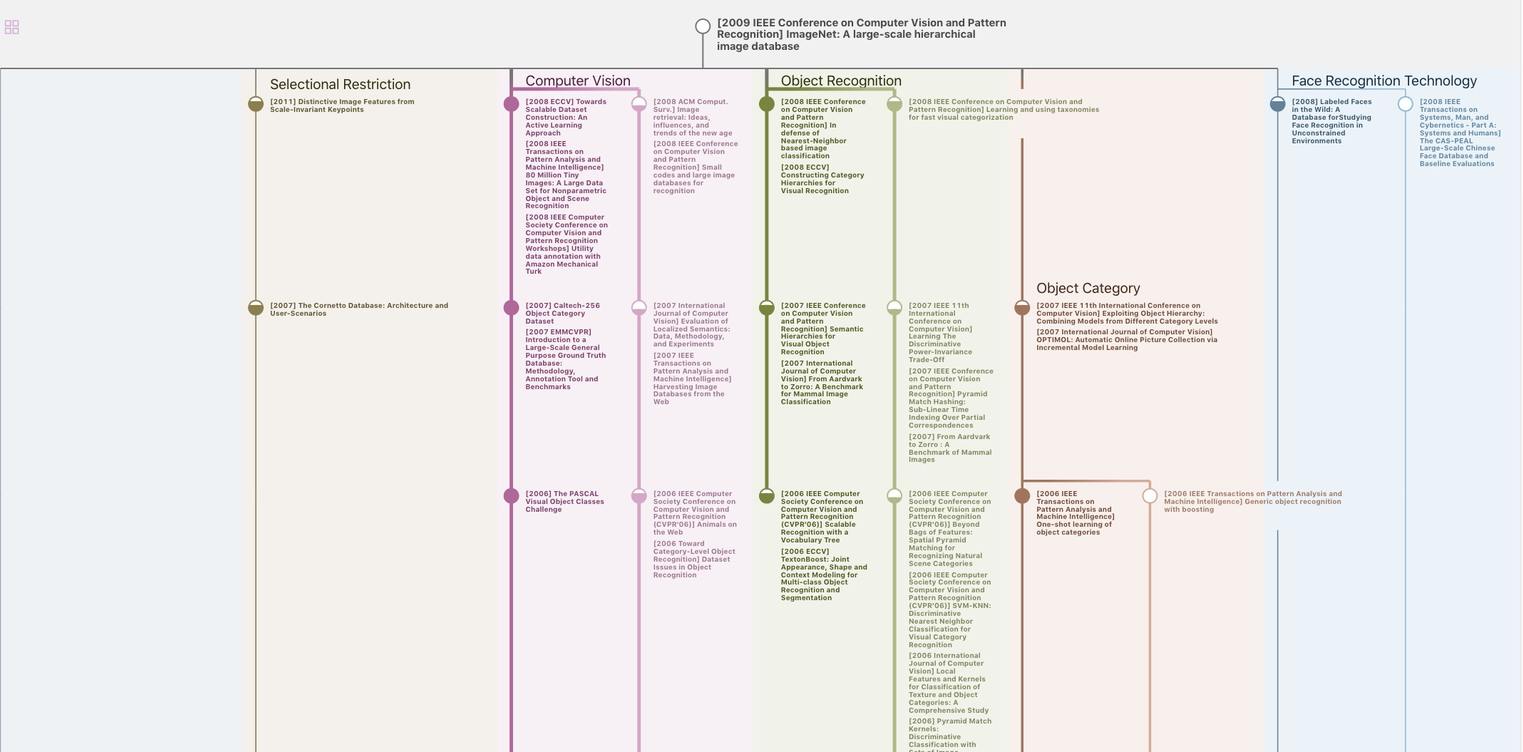
生成溯源树,研究论文发展脉络
Chat Paper
正在生成论文摘要