Robust object estimation using generative-discriminative inference for secure robotics applications
ICCAD-IEEE ACM International Conference on Computer-Aided Design(2018)
摘要
Convolutional neural networks (CNNs) are of increasing widespread use in robotics, especially for object recognition. However, such CNNs still lack several critical properties necessary for robots to properly perceive and function autonomously in uncertain, and potentially adversarial, environments. In this paper, we investigate factors for accurate, reliable, and resource-efficient object and pose recognition suitable for robotic manipulation in adversarial clutter. Our exploration is in the context of a three-stage pipeline of discriminative CNN-based recognition, generative probabilistic estimation, and robot manipulation. This pipeline proposes using a SAmpling Network Density filter, or SAND filter, to recover from potentially erroneous decisions produced by a CNN through generative probabilistic inference. We present experimental results from SAND filter perception for robotic manipulation in tabletop scenes with both benign and adversarial clutter. These experiments vary CNN model complexity for object recognition and evaluate levels of inaccuracy that can be recovered by generative pose inference. This scenario is extended to consider adversarial environmental modifications with varied lighting, occlusions, and surface modifications.
更多查看译文
关键词
Robust machine learning,Robot perception,DNN adversarial attack
AI 理解论文
溯源树
样例
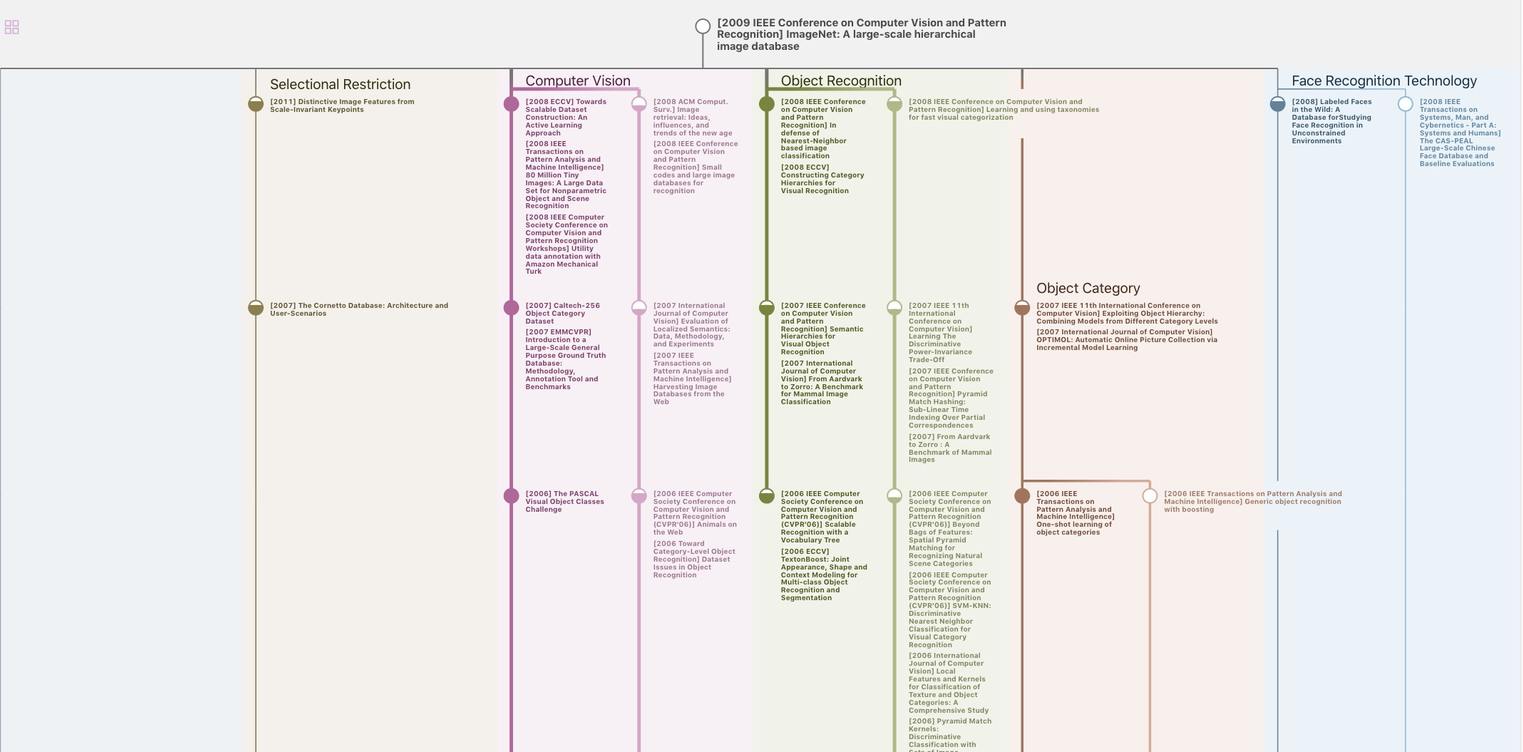
生成溯源树,研究论文发展脉络
Chat Paper
正在生成论文摘要