Feature Analysis On English Word Difficulty By Gaussian Mixture Model
2018 INTERNATIONAL CONFERENCE ON INFORMATION AND COMMUNICATION TECHNOLOGY CONVERGENCE (ICTC)(2018)
摘要
Machine Learning has significantly improved Natural Language Process (NLP) recently. In this paper, we firstly adopt the NLP approaches to extract features which represent the difficulty levels of English words. Then, Principal Component Analysis (PCA) is applied to reduce the feature dimension for visualization of data points, which intuitively justifies the selection of the features. More elaborated analysis is carried out using Gaussian Mixture Model (GMM) which clusters the data points in the reduced dimensional space. The analysis verifies that the proposed features appropriately contribute to the prediction of English word difficulty level. Finally, we demonstrate that 73.5% of classification accuracy can be achieved with Support Vector Machine (SVM) with the features we proposed.
更多查看译文
关键词
PCA, word difficulty level, difficulty features, GMM, SVM
AI 理解论文
溯源树
样例
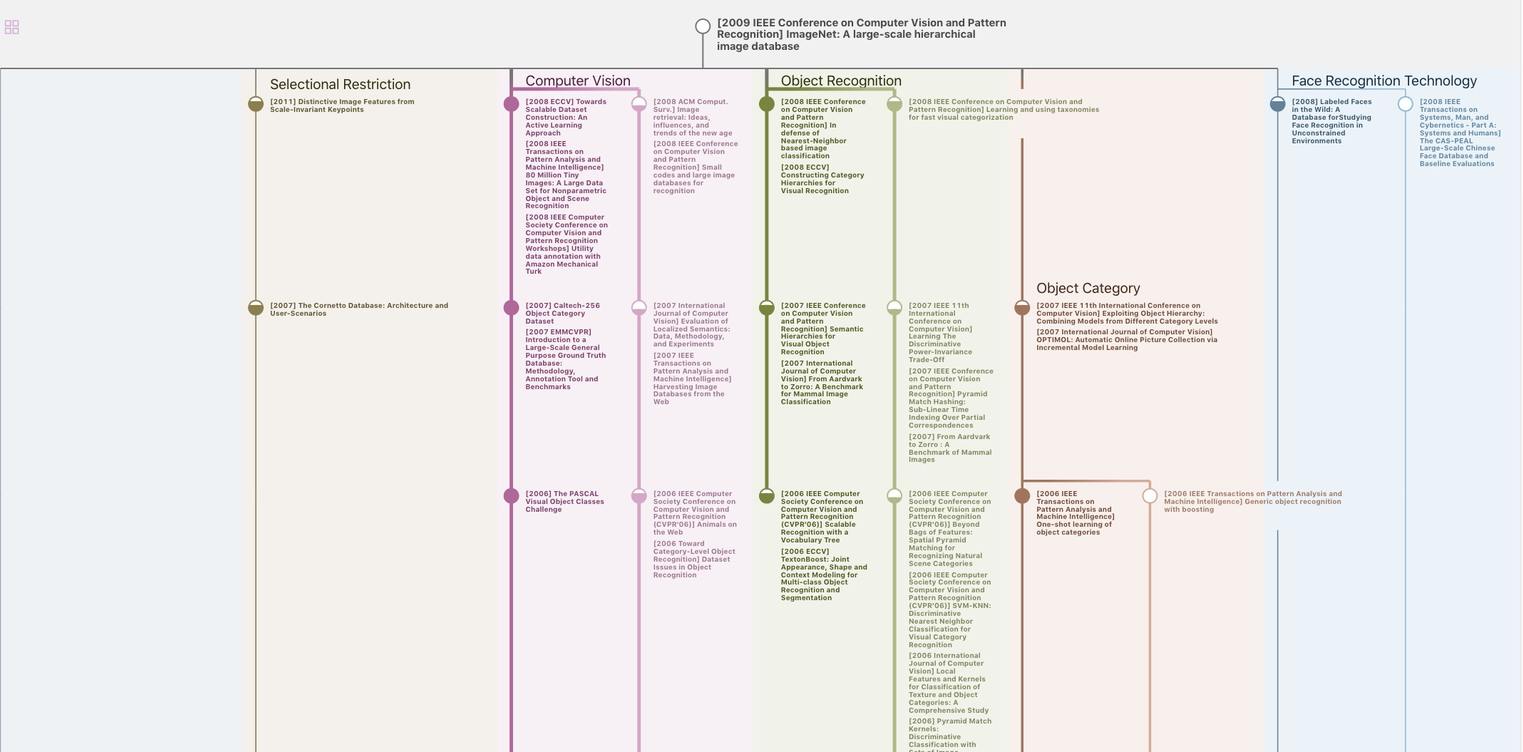
生成溯源树,研究论文发展脉络
Chat Paper
正在生成论文摘要