Feature Visualization Based Stacked Convolutional Neural Network for Human Body Detection in a Depth Image.
PRCV(2018)
摘要
Human body detection is a key technology in the fields of biometric recognition, and the detection in a depth image is rather challenging due to serious noise effects and lack of texture information. For addressing this issue, we propose the feature visualization based stacked convolutional neural network (FV-SCNN), which can be trained by a two-layer unsupervised learning. Specifically, the next CNN layer is obtained by optimizing a sparse auto-encoder (SAE) on the reconstructed visualization of the former to capture robust high-level features. Experiments on SZU Depth Pedestrian dataset verify that the proposed method can achieve favorable accuracy for body detection. The key of our method is that the CNN-based feature visualization actually pursues a data-driven processing for a depth map, and significantly alleviates the influences of noise and corruptions on body detection.
更多查看译文
关键词
Human detection, Depth image, Feature visualization Sparse auto-encoder, Convolutional neural network
AI 理解论文
溯源树
样例
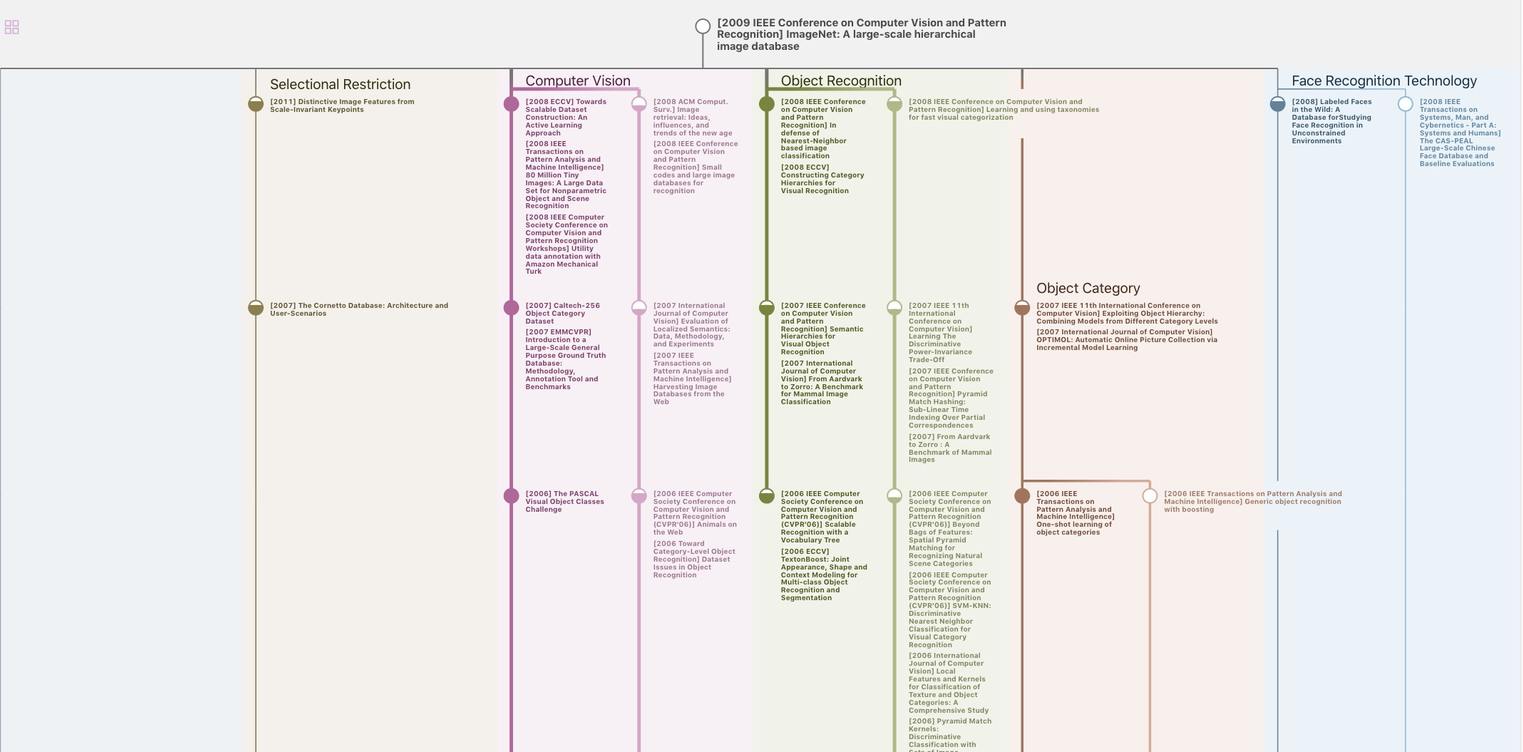
生成溯源树,研究论文发展脉络
Chat Paper
正在生成论文摘要