An online learning approach for robust motion tracking in liver ultrasound sequence
Lecture Notes in Computer Science (including subseries Lecture Notes in Artificial Intelligence and Lecture Notes in Bioinformatics)(2018)
摘要
Suffering from respiratory motion and drift, radiotherapy requires real-time and accuracy motion tracking to minimize damage to critical structures and optimize dosage delivery to target. In this paper, we propose a robust tracker to minimize tracking error and enhance the quality of radiotherapy based on two-dimensional ultrasound sequences. We firstly develop a scale adaptive kernel correlation filter to compensate deformation. Then the filter with an improved update rule is utilized to predict target position. Moreover, displacement and appearance constrains are elaborately devised to restrict unreasonable positions. Finally, a weighted displacement is calculated to further improve the robustness. Proposed method has been evaluated on 53 targets, yielding 1.13 ± 1.07 mm mean and 2.31 mm 95%ile tracking error. Extensive experiments are performed between proposed and state-of-the-art algorithms, and results show our algorithm is more competitive. Favorable agreement between automatically and manually tracked displacements proves proposed algorithm has potential for target motion tracking in abdominal radiotherapy.
更多查看译文
关键词
Target tracking, Kernel correlation filter, Scale adaptation, Displacement and appearance constrain, Radiotherapy
AI 理解论文
溯源树
样例
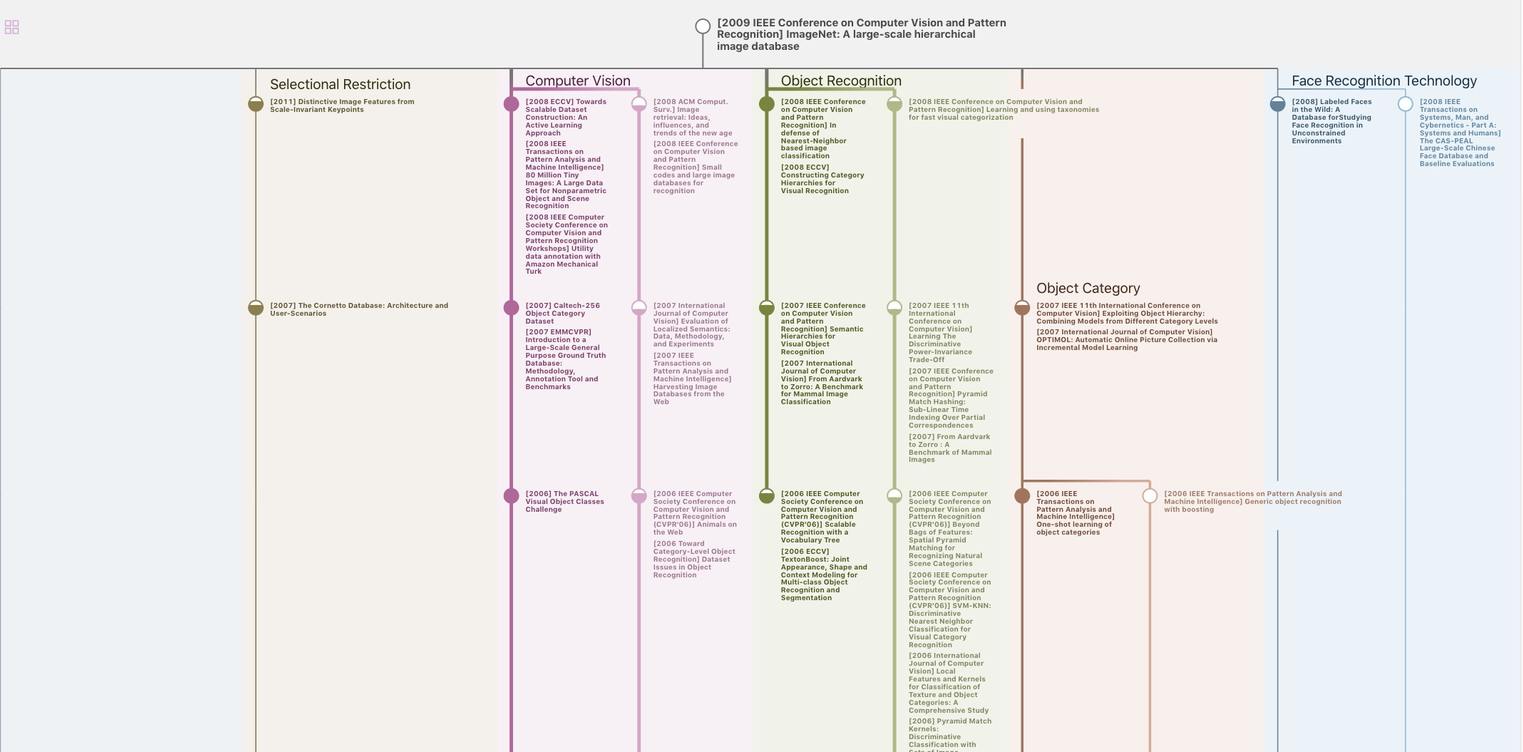
生成溯源树,研究论文发展脉络
Chat Paper
正在生成论文摘要