Built-Up Area Extraction from Landsat 8 Images Using Convolutional Neural Networks with Massive Automatically Selected Samples.
PRCV(2018)
摘要
Extraction of built-up area (e.g., roads, buildings, and other Man-made object) from remotely sensed imagery plays an important role in many urban applications. This task is normally difficult due to complex data in the form of heterogeneous appearance with large intra-class variations and lower inter-class variations. In order to extract the built-up area of 15-m resolution based on Landsat 8-OLI images, we propose the convolutional neural networks (CNN) which built in Google Drive using Colaboratory-Tensorflow. In this Framework, Google Earth Engine (GEE) provides massive remote sensing images and preprocesses the data. In this proposed CNN, for each pixel, the spectral information of the 8 bands and the spatial relationship in the 5 neighborhood are taken into account. Training this network requires lots of sample points, so we propose a method based on the ESA’s 38-m global built-up area data of 2014, Open Street Map and MOD13Q1-NDVI to achieve rapid and automatic generation of large number of sample points. We choose Beijing, Lanzhou, Chongqing, Suzhou and Guangzhou of China as the experimentation sites, which represent different landforms and different urban environments. We use the proposed CNN to extract built-up area, and compare the results with other existing building data products. Our research shows: (1) The test accuracy of the five experimental sites is higher than 89%. (2) The classification results of CNN can be very good for the details of the built-up area, and greatly reduce the classification error and leakage error. Therefore, this paper provides a reference for the classification and mapping of built-up areas in large space range.
更多查看译文
关键词
Extract built-up area, CNN, Landsat 8 image, Generate samples, Google Earth Engine, Google Colaboratory
AI 理解论文
溯源树
样例
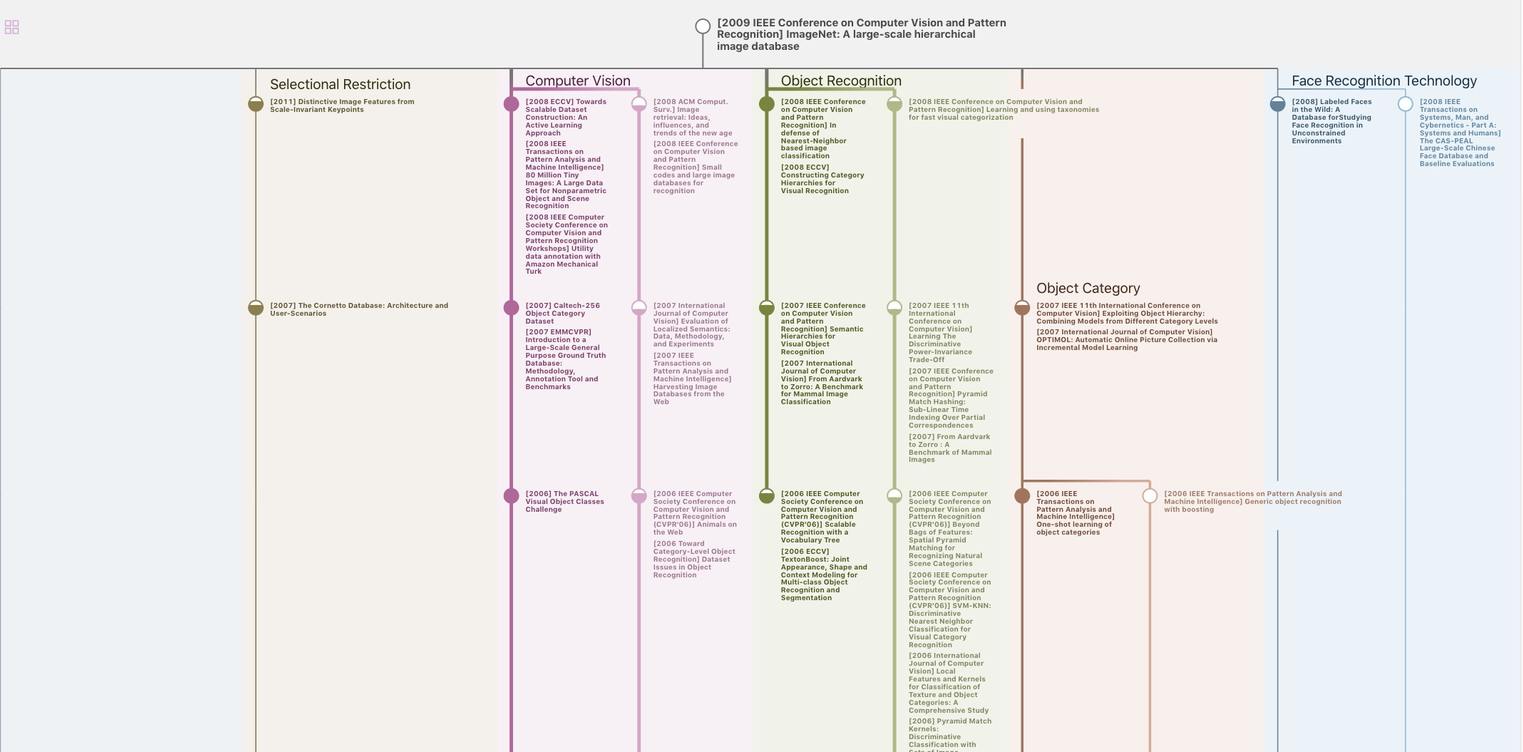
生成溯源树,研究论文发展脉络
Chat Paper
正在生成论文摘要