Conditional Face Synthesis for Data Augmentation.
PRCV(2018)
摘要
Conditional face synthesis has been an appealing yet challenging problem in computer vision. It has a wide range of applications. However, few works attempt to leverage the synthesized face images for data augmentation and improve performance of recognition model. In this paper, we propose a conditional face synthesis framework that combines a variational auto-encoder with a conditional generative adversarial network, for synthesizing face images with specific identity. Our approach has three novel aspects. First, we propose to leverage the synthesized face images to do data augmentation and train a better recognition model. Second, we adopt multi-scale discriminators to enable high-quality image generation. Third, we adopt identity-preserving loss and classification loss to ensure identity invariance of synthesized images, and use feature matching loss to stabilize the GAN training. With extensive qualitative and quantitative evaluation, we demonstrate that face images generated by our approach are realistic, discriminative and diverse. We further show that our approach can be used for data augmentation and train superior face recognition models.
更多查看译文
关键词
synthesis
AI 理解论文
溯源树
样例
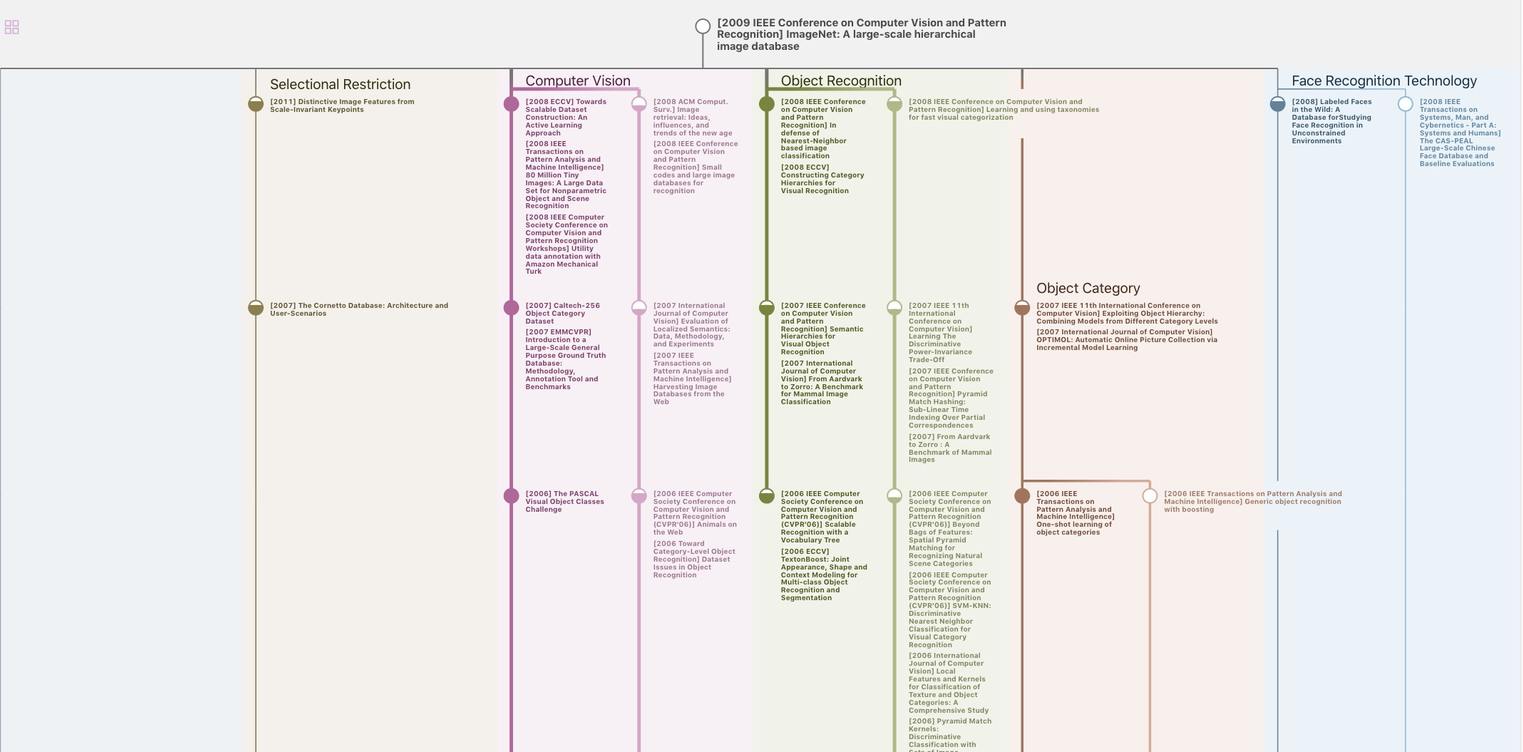
生成溯源树,研究论文发展脉络
Chat Paper
正在生成论文摘要