Dominant-Set-Based Consensus For Fuzzy C-Means Clustering Ensemble
2018 International Conference on Machine Learning and Cybernetics (ICMLC)(2018)
摘要
Conventional clustering approaches partition a set of objects into a certain (some can automatically detect the number of clusters such as DBScan) number of clusters. During the partitioning process, the clusters of objects are produced where each object is assigned to one cluster. On the other hand, the dominantset-based clustering provides a formalisation of clusters by sequentially searching for individual clusters in the set of objects. The resultant clusters do not necessarily form a partition of the set. With the popularity of clustering ensemble, graph-based consensus approaches have been proposed with promising results achieved, many of which are based on the partition of the graph. In this paper, a dominant-set-based consensus method for fuzzy-c-means-based clustering ensemble is proposed. Different from traditional graph-based consensus techniques, the graph generated by the fuzzy clusters are grouped on the basis of the extracted dominant sets. The proposed approach employs a similarity relation to denote the links between component clusters from which the final clusters of ensemble are derived with the extracted dominant set. The proposed method is tested on benchmark data sets against several alternative ensemble methods for fuzzy c-means. The results of experiment show that the proposed dominant-set-based clustering ensemble method generally achieves higher accuracy than its competitors.
更多查看译文
关键词
Dominant set,Clustering ensemble,Consensus function,Fuzzy c-means,Clustering
AI 理解论文
溯源树
样例
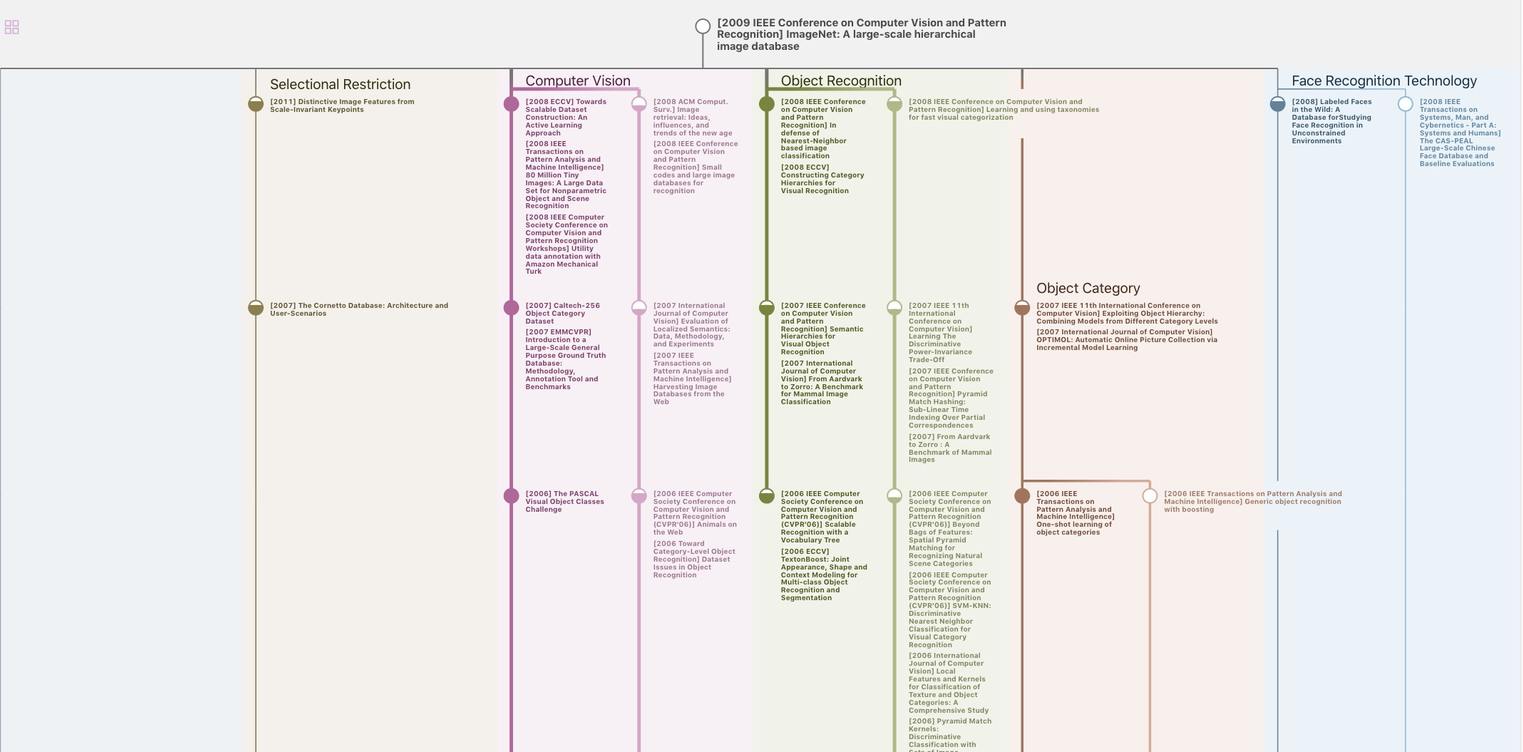
生成溯源树,研究论文发展脉络
Chat Paper
正在生成论文摘要