Weight Learning in Weighted ELM Classification Model Based on Genetic Algorithms
2018 International Conference on Machine Learning and Cybernetics (ICMLC)(2018)
摘要
In cost sensitive classification problems we often suppose to have a known cost matrix in which each element represents the cost of mistakenly classifying an object from one class into another. Weighted least square, which does not equally consider individual classes and therefore assigns a different weight to each class of samples, is a typical approach to dealing with cost sensitive classification problems. Theoretically and experimentally it is confirmed that reasonable class weights will greatly improve classification ability of a learning model. Unfortunately we only know that these weights depend generally on cost matrix but very few methods can be used to specifically determine these weights according to cost matrix. This paper proposes a weighted least square (WLS) model of random weight network and then successfully uses the model in cost sensitive classification. A genetic algorithm to determine weights of different sample classes based on a cost matrix is given. Model analysis and experimental simulations are conducted. Considering the total misclassification cost as the evaluation index, a comparative study shows that our WLS model is far superior to the existing cost sensitive ELM and cost sensitive naive Bayes models.
更多查看译文
关键词
Weighted least square method,Cost sensitive,Cost matrix,Random weight network,Genetic algorithm
AI 理解论文
溯源树
样例
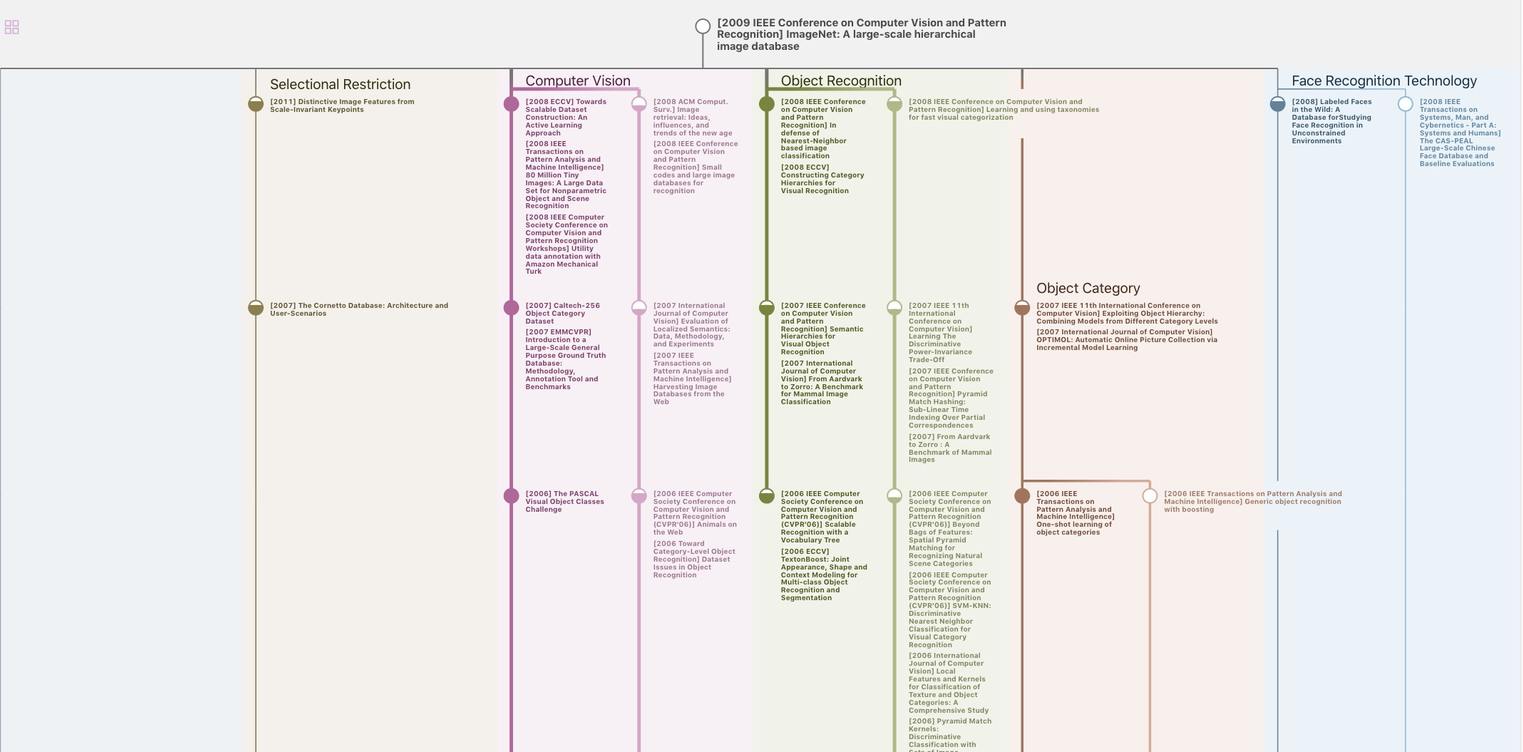
生成溯源树,研究论文发展脉络
Chat Paper
正在生成论文摘要