A Missing Data Imputation Method With Distance Function
2018 International Conference on Machine Learning and Cybernetics (ICMLC)(2018)
摘要
“Missing data” is an important research issue in big data analysis. This problem may cause data hard to analyze precisely. In recent research, several imputation-based methods have been proposed to solve the missing data issue without using domain knowledge.Among them, the missing data imputation method based on association rule mining was proposed to determine which value should be filled in the missing data. However, the generated rules may not always be suitable for filling in missing values. For example, some strong rules may fill up different missing values with the same result. We propose here an algorithm named RID (Rule-based Imputation with Distance function) to deal with this shortcoming. RID generates rules for missing data imputation by association rule mining and then uses a distance function to adjust the rule to fill in values appropriately. Experimental results show that the accuracy of RIDis approximately 3 to 5 percentage higher than those of C4.5 and kNN, and approximately 6 to 7 percentage higher than that of HMiT.
更多查看译文
关键词
Big data,Missing data,Association rules,Distance function,Imputation
AI 理解论文
溯源树
样例
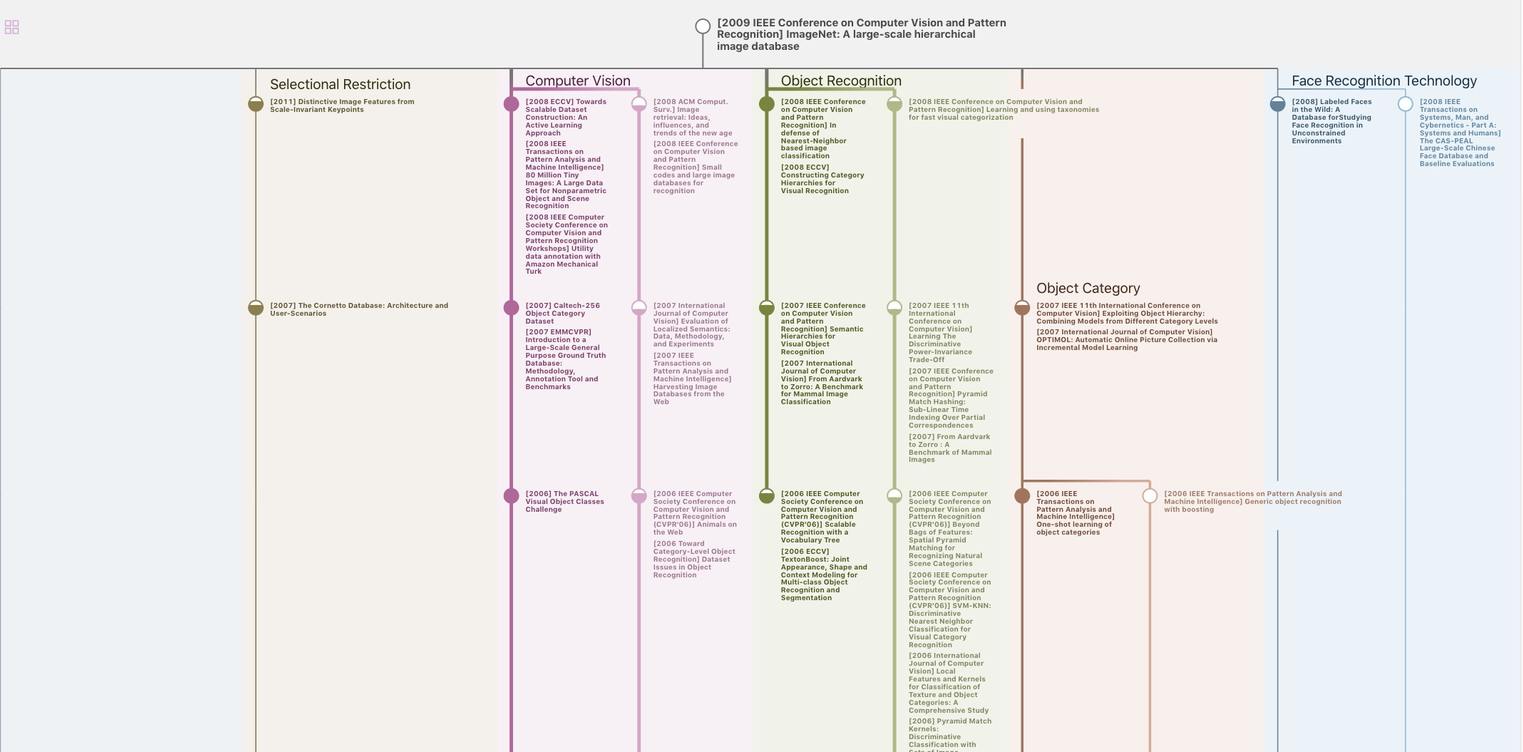
生成溯源树,研究论文发展脉络
Chat Paper
正在生成论文摘要