A Data-Driven Method To Dissect The Dynamics Of The Causal Influence In Complex Dynamical Systems
2018 IEEE WORKSHOP ON COMPLEXITY IN ENGINEERING (COMPENG 2018)(2018)
摘要
In several natural and physical systems, reconstructing the graph underlying the interactions is fundamental to understand the interplay between units and the mechanisms at the base of their collective behavior. This is the case of coupled networked dynamical systems where due to several environmental and physical factors such as obstacles, sensors limited range, or components failure, the interaction network might vary in time and space. Currently, such dynamics cannot be fully captured by most existing tools nor there exists any available tools to capture these changes in real time. Here, we present a novel method to infer changes in the causal influence of units of a coupled dynamical system. The approach builds on network and information theories to propose a metric evaluating the influence as time evolves of any node on others. The method is validated on self-propelled particles where particles influence status is subject to vary over time. Our proposed method is expected to enrich the toolbox for reconstructing directed interactions in quasi-real time with few data.
更多查看译文
关键词
Causality, data-driven, dynamical systems, network, interactions, transfer entropy
AI 理解论文
溯源树
样例
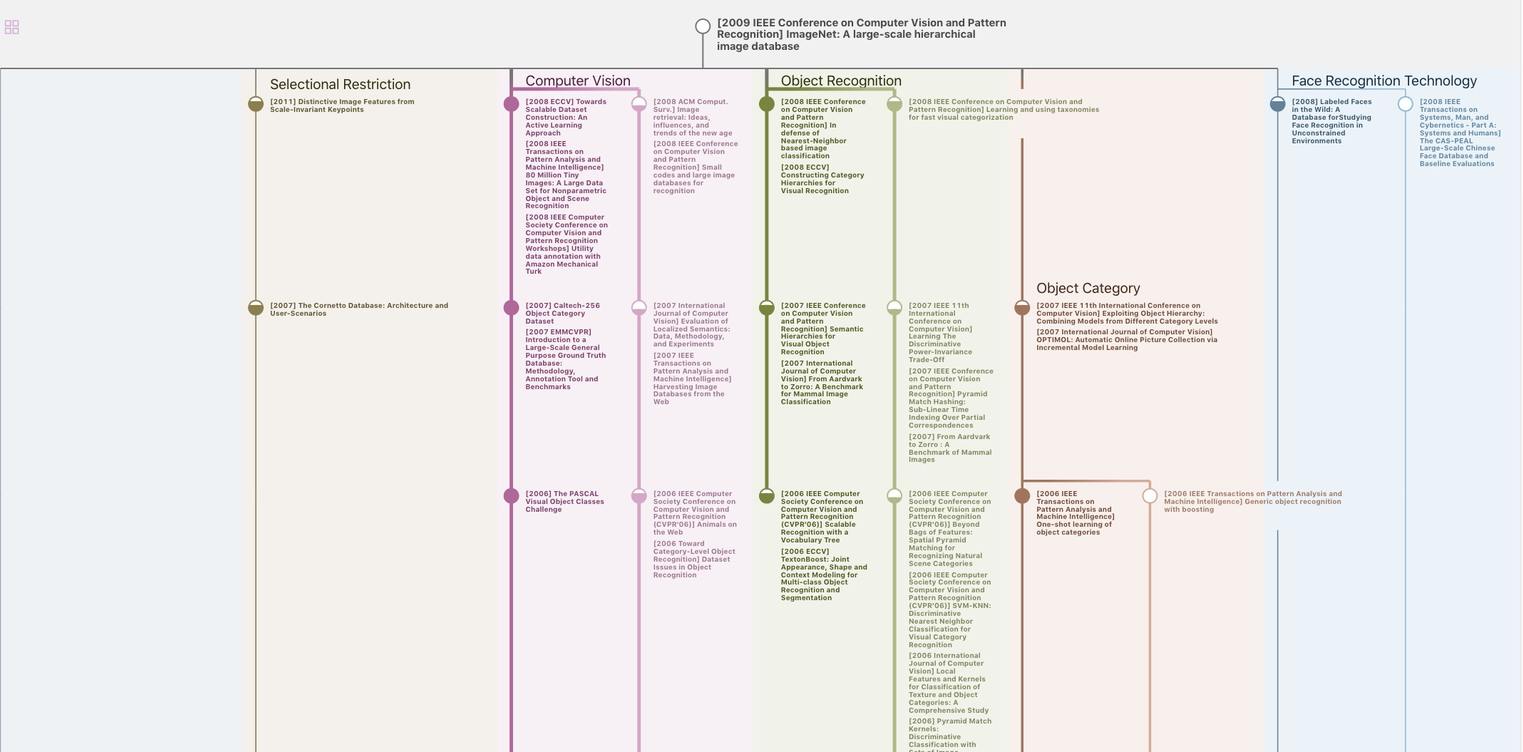
生成溯源树,研究论文发展脉络
Chat Paper
正在生成论文摘要