Relative Attribute Based Unmixing
IGARSS 2018 - 2018 IEEE INTERNATIONAL GEOSCIENCE AND REMOTE SENSING SYMPOSIUM(2018)
摘要
The abundance of a mixed pixel of certain class can be understood as to get the relative score referring to the pure representative of this class, while not be classified with two absolute and discrete value as "1 or 0". This is in accordance with the Relative Attribute Learning (RAL) problem in computer vision. In RAL, the concept of "relative attribute" is used to describe the belonging level of an object to certain class with a score which is achieved from a learn-to-rank problem using rankSVM framework. To utilize information between data samples and even of mixed pixels, Relative Attribute based Unmixing (RAU) is proposed first time by using relative attribute to describe the abundance of mixed pixel as relative purity of certain class and learn the abundance with rankSVM. The mixed data sample are used to construct training comparisons set in rankSVM with archetypes generated by the reported Kernel Archetypal Analysis (KAA) unmixing method. In addition, spectral variability is also addressed by constructing comparisons set with synonyms spectrum achieved from KAA. Experiments on both synthetic and real hyperspectral mixed image have demonstrated the potential value of proposed method for mixed pixel analysis.
更多查看译文
关键词
Hyperspectral image, spectral unmixing, NMF, spectral variability, relative attribute
AI 理解论文
溯源树
样例
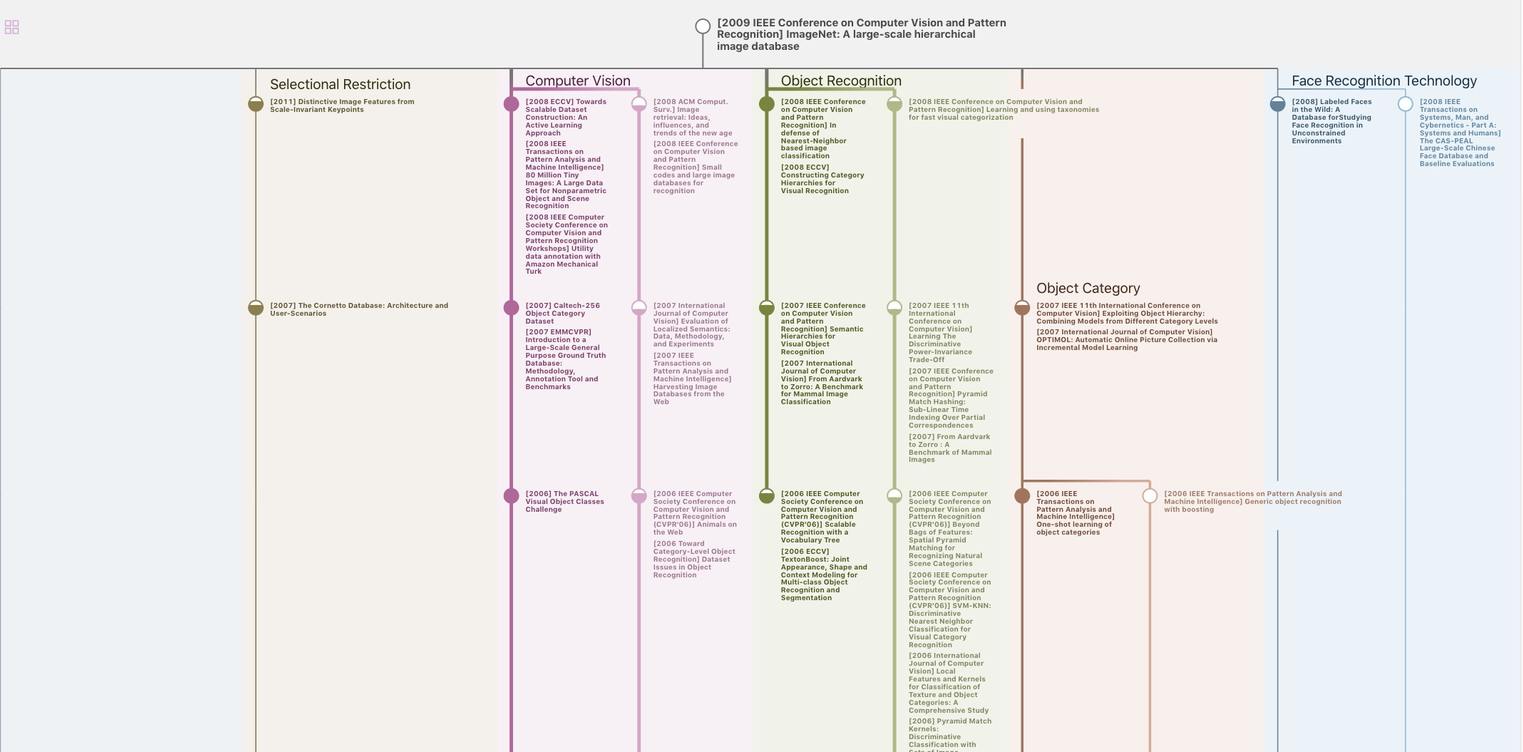
生成溯源树,研究论文发展脉络
Chat Paper
正在生成论文摘要