Dotanet: Two-Stream Match-Recurrent Neural Networks For Predicting Social Game Result
2018 IEEE FOURTH INTERNATIONAL CONFERENCE ON MULTIMEDIA BIG DATA (BIGMM)(2018)
摘要
As one of the most popular social online games of the electronic, DOTA2 (Defense of the Ancients 2) has attracted numerous players all over the world. How to automatically predict the winner of two teams before battle becomes an interesting and practical topic. Since Deep Learning has achieved great success in various classification and prediction tasks of artificial intelligence area, this work exploits Recurrent Neural Network (RNN) to train a winner predictor of DOTA2 game with two battle teams by leveraging the information of massive team rankings and post-match data of teams. Specifically, to model the sequence of previous match data well, we propose an effective Two-stream Match-Recurrent Neural Networks equipped with a new Dota Loss layer (Called DotaNet). In DotaNet, the input features of a battle team pair (i.e., Team A and Team B) are collected and quantified from their available team rankings and post-match data, followed by two sub-RNNs (e.g., GRU or LSTM). Second, the two hidden states of the team pairs from two sub-RNN are fed into a new Dota Loss layer, which outputs the winning probability of Team A vs Team B. In experiments, we collect a new DOTA2 Game Prediction (DGP) dataset from the available team rankings and post-match data, and evaluate the prediction performance of the proposed method compared with the traditional deep learning methods.
更多查看译文
关键词
Deep Neural Networks, Recurrent Neural Network, Long Short Term Memory, Game Result Prediction
AI 理解论文
溯源树
样例
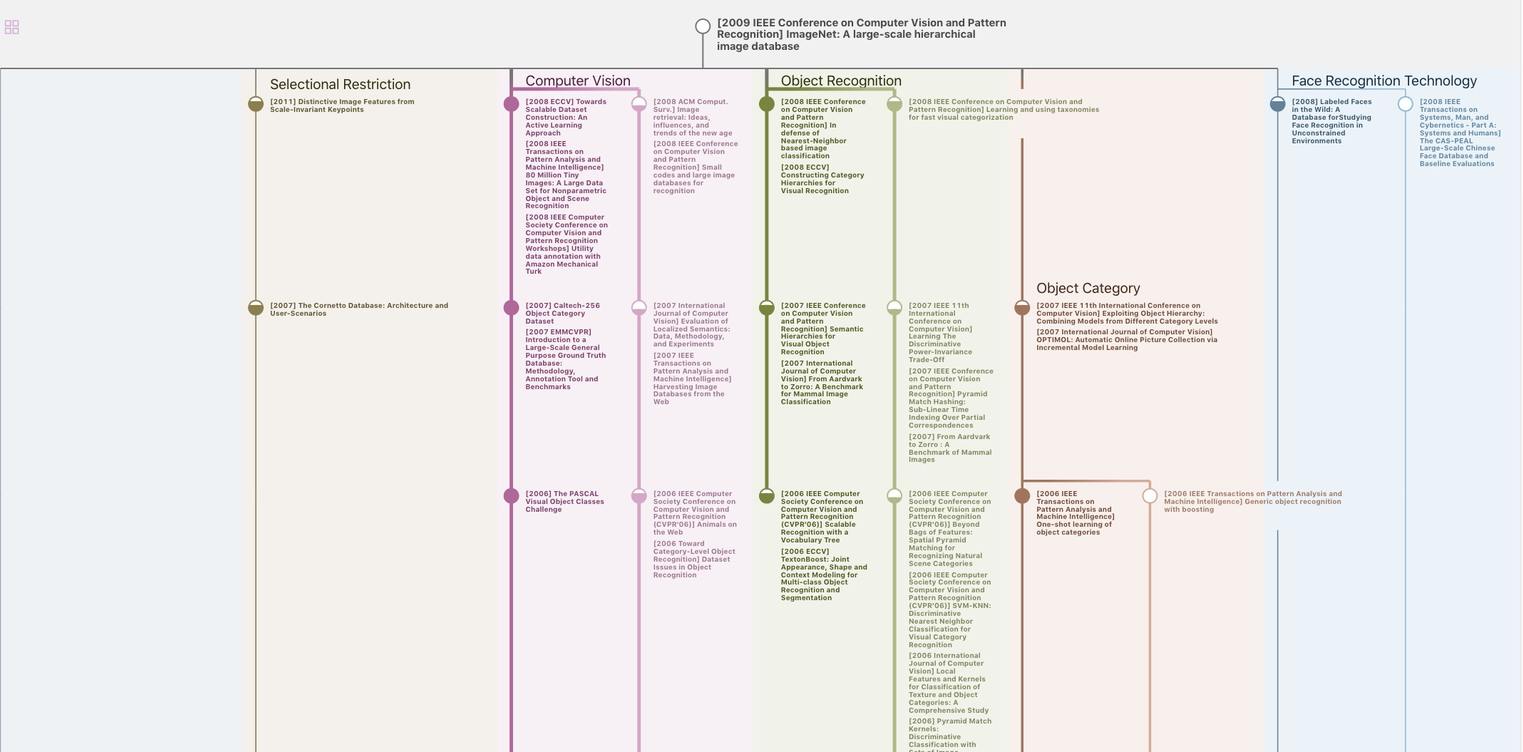
生成溯源树,研究论文发展脉络
Chat Paper
正在生成论文摘要