Pfnet: A Novel Part Fusion Network For Fine-Grained Image Categorization
2018 IEEE FOURTH INTERNATIONAL CONFERENCE ON MULTIMEDIA BIG DATA (BIGMM)(2018)
摘要
Previous work in fine-grained image categorization focuses on integrating multiple deep CNN models or complicated attention mechanism, resulting in increasing cumbersome networks, and requiring expensive expert guidance. In this work, a part fusion network (PFNet) is proposed to fuse image parts for classification, which consists of a Fast R-CNN head to extract part features and a two-level classification network to encode part-level and image-level features simultaneously. Features from These two levels are trained with focal loss and cross-entropy loss respectively. By utilizing the focal loss, PFNet can learn more from hard examples of parts and thus generate more discriminative image features.Furthermore, it does not require extra part annotations and can be trained end to end. Experiments are performed on three challenging datasets in fine-grained image categorization task, namely, CUB-200-2011, Stanford Cars and FGVC-Aircraft, and results show that the PFNnet proposed in this work achieves promising results comparing with the state of the art. In datasets Stanford Cars and FGVC-Aircraft, our PFNet outperforms the best results marginally reported in previous work, and achieves comparable accuracy in datasets CUB-200-2011.
更多查看译文
关键词
Fine-grained categorization, CNN, image classification, PFNet
AI 理解论文
溯源树
样例
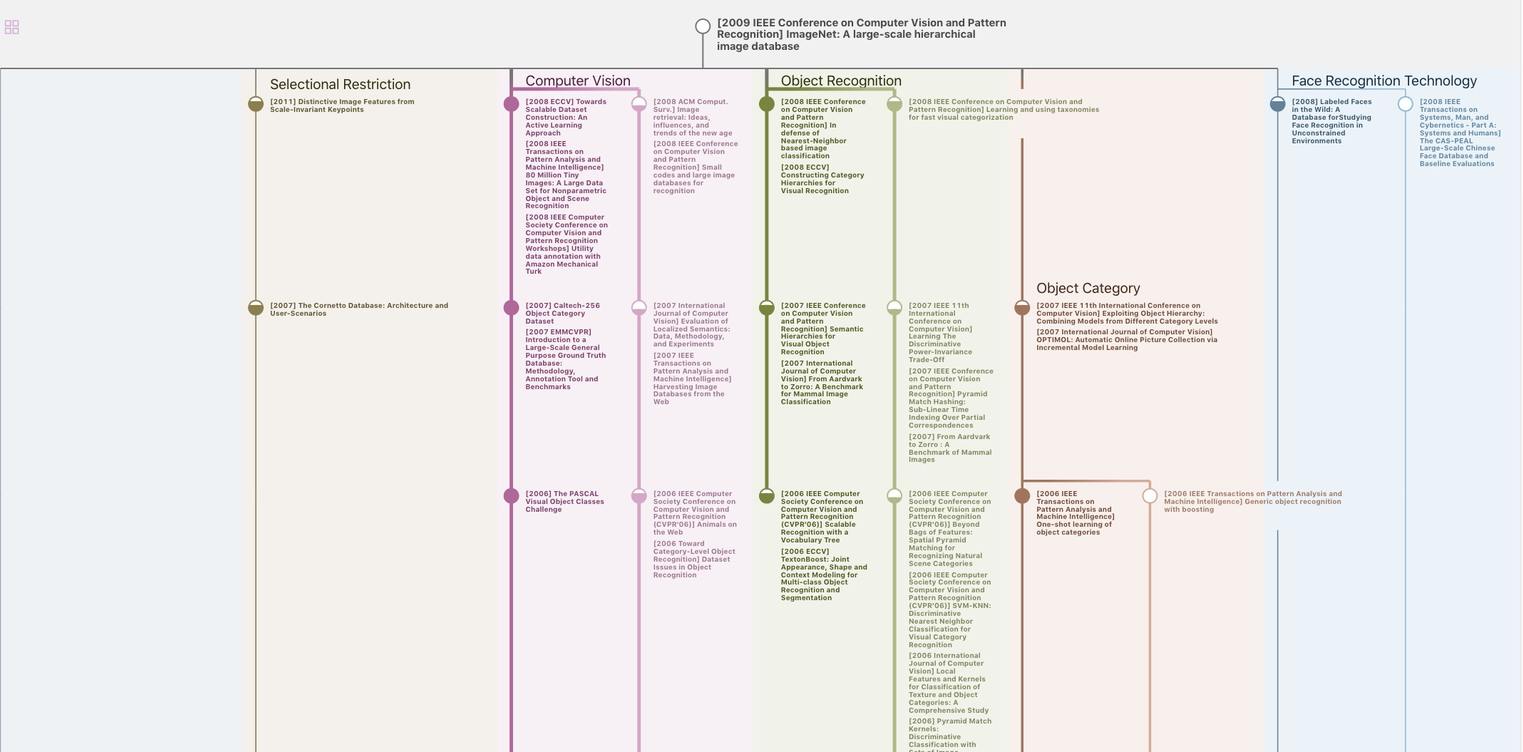
生成溯源树,研究论文发展脉络
Chat Paper
正在生成论文摘要