Near Perfect Neural Critic from Motor Cortical Activity Toward an Autonomously Updating Brain Machine Interface
2018 40th Annual International Conference of the IEEE Engineering in Medicine and Biology Society (EMBC)(2018)
摘要
We are developing an autonomously updating brain machine interface (BMI) utilizing reinforcement learning principles. One aspect of this system is a neural critic that determines reward expectations from neural activity. This critic is then used to update a BMI decoder toward an improved performance from the user's perspective. Here we demonstrate the ability of a neural critic to classify trial reward value given activity from the primary motor cortex (M1), using neural features from single/multi units (SU/MU), and local field potentials (LFPs) with prediction accuracies up to 97% correct. A nonhuman primate subject conducted a cued center out reaching task, either manually, or observationally. The cue indicated the reward value of a trial. Features such as power spectral density (PSD) of the LFPs and spike-field coherence (SFC) between SU/MU and corresponding LFPs were calculated and used as inputs to several classifiers. We conclude that hybrid features of PSD and SFC show higher classification performance than PSD or SFC alone (accuracy was 92% for manual tasks, and 97% for observational). In the future, we will employ these hybrid features toward our autonomously updating BMI.
更多查看译文
关键词
Animals,Brain-Computer Interfaces,Learning,Motor Cortex,Reinforcement, Psychology,Reward
AI 理解论文
溯源树
样例
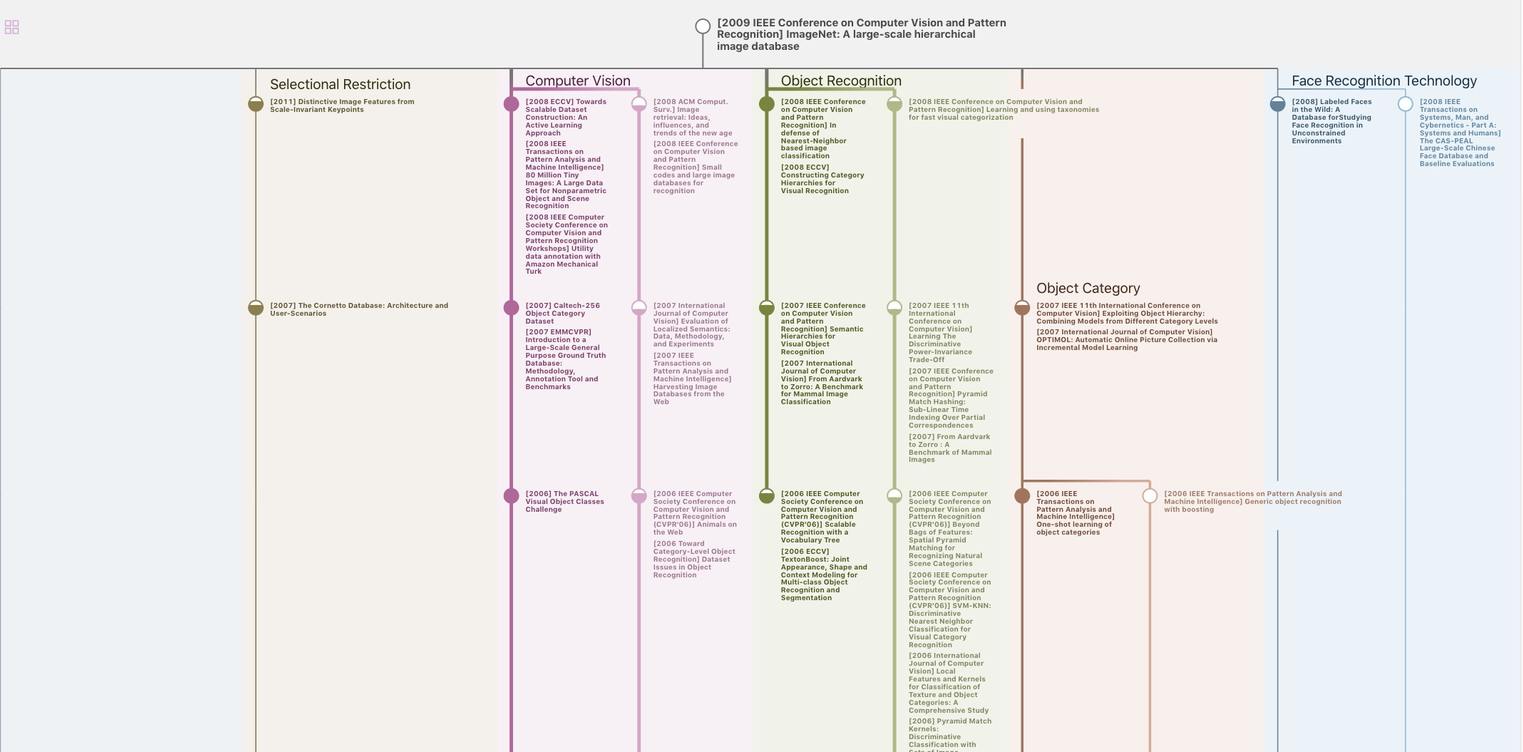
生成溯源树,研究论文发展脉络
Chat Paper
正在生成论文摘要