Breast Region Segmentation Using Convolutional Neural Network in Dynamic Contrast Enhanced MRI
EMBC(2018)
摘要
Breast density and background parenchymal enhancement (BPE) are suggested to be related to the risk of breast cancer. The first step to quantitative analysis of breast density and BPE is segmenting the breast from body. Nowadays, convolutional neural networks (CNNs) are widely used in image segmentation and work well in semantic segmentation, however, CNNs have been rarely used in breast region segmentation. In this paper, the CNN was employed to segment the breast region in transverse fat-suppressed breast dynamic contrast enhanced magnetic resonance imaging (DCE-MRI). Image normalization was initially performed. Subsequently, the dataset was divided into three sets randomly: train set, validation set and test set. The 2-D U-Net was trained by train set and the optimum model was chosen by validation set. Finally, segmentation results of test set obtained by U-Net were adjusted in the postprocessing. In this step, two largest volumes were computed to determine whether the smaller volume is the scar after mastectomy. With the limitation of small dataset, 5-fold cross-validation and data augmentation were used in this study. Final results on the test set were evaluated by volume-based and boundary-based metrics with manual segmentation results. By using this method, the mean dice similarity coefficient (DSC), dice difference coefficient (DDC), and root-mean-square distance reached 97.44%, 5.11%, and 1.25 pixels, respectively.
更多查看译文
关键词
Breast Neoplasms,Humans,Image Processing, Computer-Assisted,Magnetic Resonance Imaging,Mastectomy,Nerve Net,Neural Networks, Computer
AI 理解论文
溯源树
样例
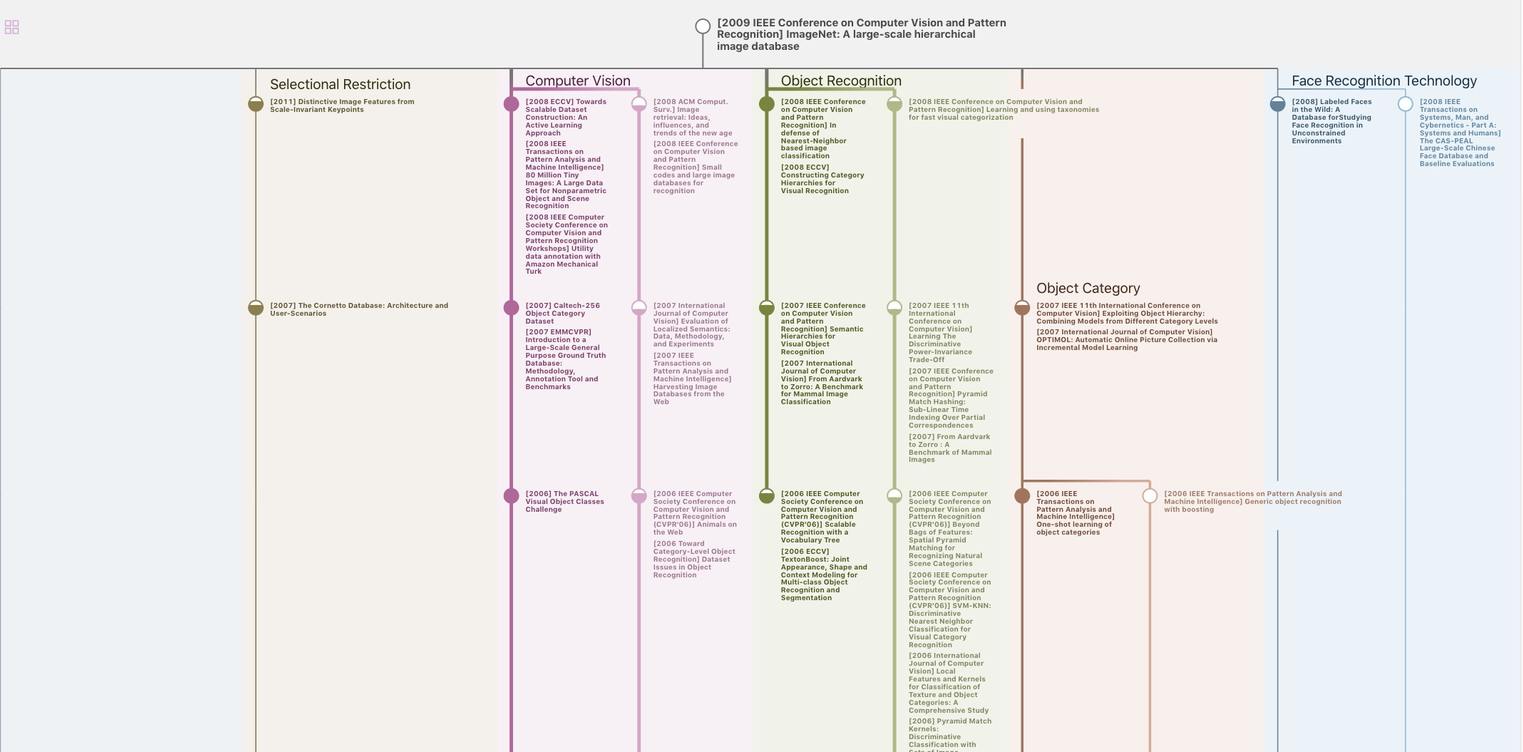
生成溯源树,研究论文发展脉络
Chat Paper
正在生成论文摘要