Joint Spoken Language Understanding And Domain Adaptive Language Modeling
INTELLIGENCE SCIENCE AND BIG DATA ENGINEERING(2018)
摘要
Spoken Language Understanding (SLU) aims to extract structured information from speech recognized texts, which suffers from inaccurate automatic speech recognition (ASR) (especially in a specific dialogue domain). Language Modeling (LM) is significant to ASR for producing natural sentences. To improve the SLU performance in a specific domain, we try two ways: (1) domain adaptive language modeling which tends to recognize in-domain utterances; (2) joint modeling of SLU and LM which distills semantic information to build semantic-aware LM, and also helps SLU by semi-supervised learning (LM is unsupervised). To unify these two approaches, we propose a multi-task model (MTM) that jointly performs two SLU tasks (slot filling and intent detection), domain-specific LM and domain-free (general) LM. In the proposed multi-task architecture, the shared-private network is utilized to automatically learn which part of general data can be shared by the specific domain or not. We attempt to further improve the SLU and ASR performance in a specific domain with a few of labeled data in the specific domain and plenty of unlabeled data in general domain. The experiments show that the proposed MTM can obtain 4.06% absolute WER (Word Error Rate) reduction in a car navigation domain, compared to a general-domain LM. For language understanding, the MTM outperforms the baseline (especially slot filling task) on the manual transcript, ASR 1-best output. By exploiting the domain-adaptive LM to rescore ASR output, our proposed model achieves further improvement in SLU (7.08% absolute F1 increase of slot filling task).
更多查看译文
关键词
Spoken language understanding, Domain adaptive, Language modeling, Semi-supervised, Multi-task
AI 理解论文
溯源树
样例
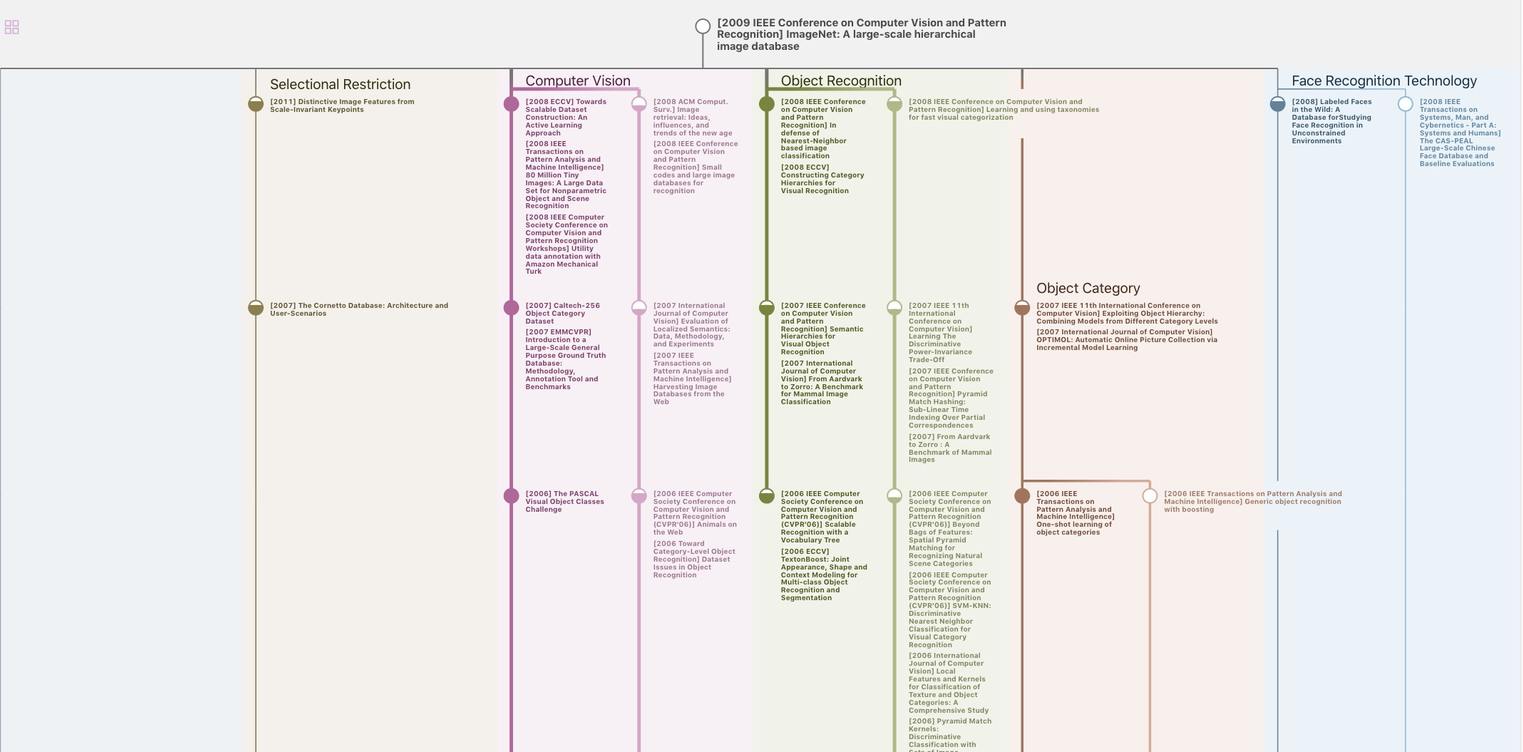
生成溯源树,研究论文发展脉络
Chat Paper
正在生成论文摘要