Large-scale hierarchical k-means for heterogeneous many-core supercomputers.
SC(2018)
摘要
This paper presents a novel design and implementation of k-means clustering algorithm targeting the Sunway TaihuLight supercomputer. We introduce a multi-level parallel partition approach that not only partitions by dataflow and centroid, but also by dimension. Our multi-level (nkd) approach unlocks the potential of the hierarchical parallelism in the SW26010 heterogeneous many-core processor and the system architecture of the supercomputer. Our design is able to process large-scale clustering problems with up to 196,608 dimensions and over 160,000 targeting centroids, while maintaining high performance and high scalability, significantly improving the capability of k-means over previous approaches. The evaluation shows our implementation achieves performance of less than 18 seconds per iteration for a large-scale clustering case with 196,608 data dimensions and 2,000 centroids by applying 4,096 nodes (1,064,496 cores) in parallel, making k-means a more feasible solution for complex scenarios.
更多查看译文
关键词
Supercomputers,Clustering algorithms,Parallel processing,Graphics processing units,Scalability,Hardware,Multicore processing
AI 理解论文
溯源树
样例
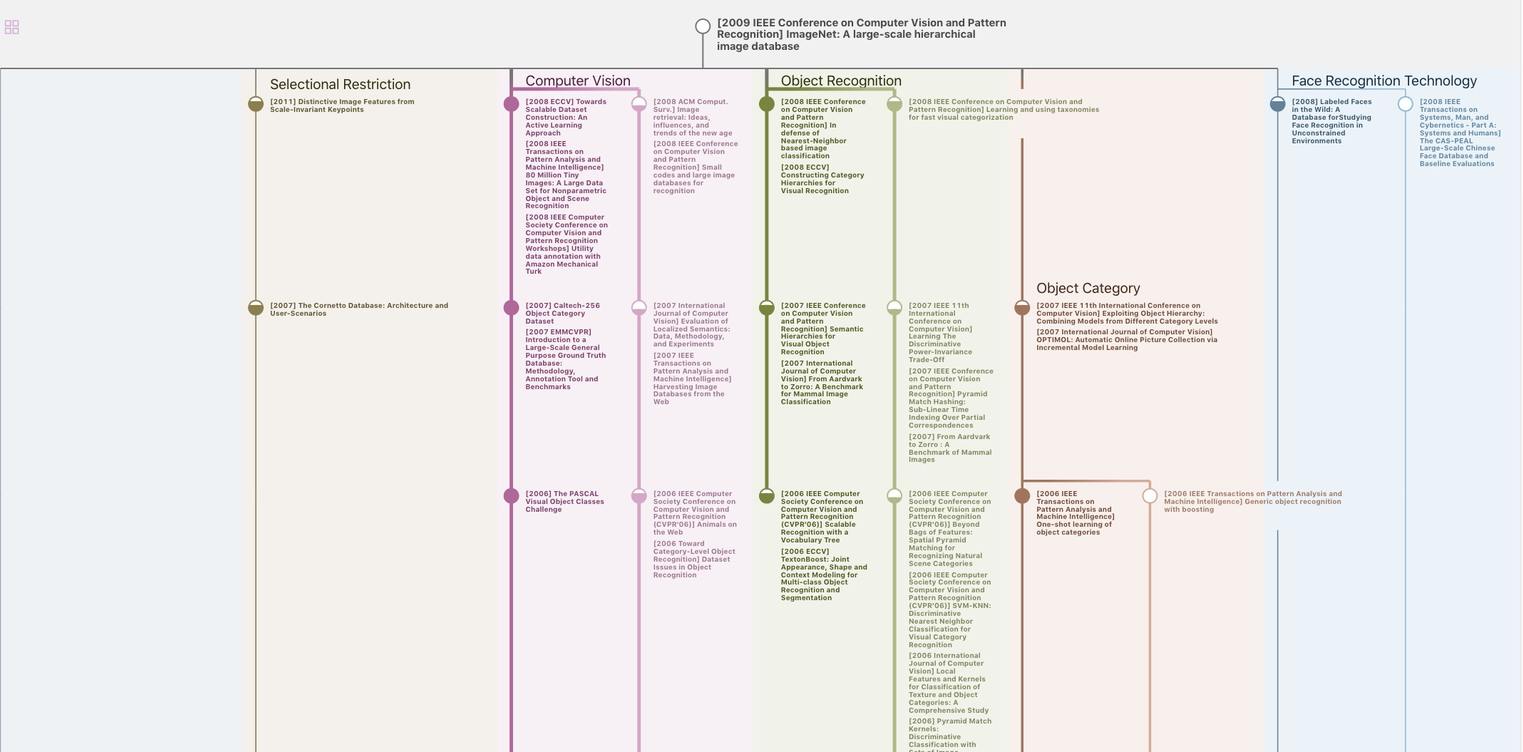
生成溯源树,研究论文发展脉络
Chat Paper
正在生成论文摘要