A Low Power, High Throughput, Fully Event-Based Stereo System
2018 IEEE/CVF CONFERENCE ON COMPUTER VISION AND PATTERN RECOGNITION (CVPR)(2018)
摘要
We introduce a stereo correspondence system implemented fully on event-based digital hardware, using a fully graph-based non von-Neumann computation model, where no frames, arrays, or any other such data-structures are used. This is the first time that an end-to-end stereo pipeline from image acquisition and rectification, multi-scale spatio-temporal stereo correspondence, winner-take-all, to disparity regularization is implemented fully on event-based hardware. Using a cluster of TrueNorth neurosynaptic processors, we demonstrate their ability to process bilateral event-based inputs streamed live by Dynamic Vision Sensors (DVS), at up to 2,000 disparity maps per second, producing high fidelity disparities which are in turn used to reconstruct, at low power the depth of events produced from rapidly changing scenes. Experiments on real-world sequences demonstrate the ability of the system to take full advantage of the asynchronous and sparse nature of DVS sensors for low power depth reconstruction, in environments where conventionalframe-based cameras connected to synchronous processors would be inefficient for rapidly moving objects. System evaluation on event-based sequences demonstrates a similar to 200 x improvement in terms of power per pixel per disparity map compared to the closest state-of-the-art, and maximum latencies of up to lI ms from spike injection to disparity map ejection.
更多查看译文
关键词
stereo correspondence system,event-based digital hardware,nonvon-Neumann computation model,disparity maps,disparity map ejection,event-based sequences,system evaluation,low power depth reconstruction,DVS sensors,rapidly changing scenes,high fidelity disparities,Dynamic Vision Sensors,bilateral event-based inputs,TrueNorth neurosynaptic processors,event-based hardware,disparity regularization,multiscale spatiotemporal stereo correspondence,rectification,image acquisition,end-to-end stereo pipeline,data-structures
AI 理解论文
溯源树
样例
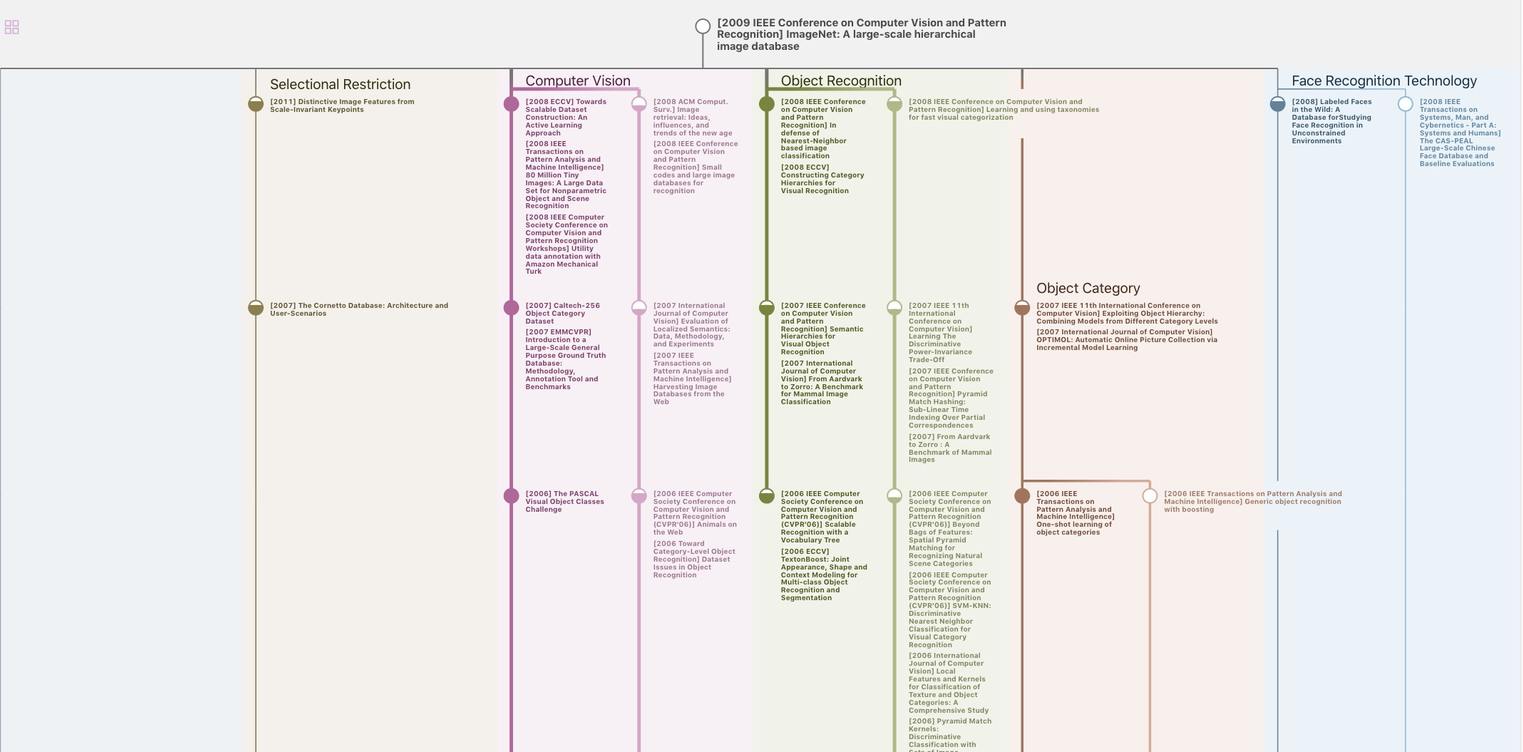
生成溯源树,研究论文发展脉络
Chat Paper
正在生成论文摘要