SurfConv: Bridging 3D and 2D Convolution for RGBD Images
2018 IEEE/CVF CONFERENCE ON COMPUTER VISION AND PATTERN RECOGNITION (CVPR)(2018)
摘要
We tackle the problem of using 3D information in convolutional neural networks for down-stream recognition tasks. Using depth as an additional channel alongside the RGB input has the scale variance problem present in image convolution based approaches. On the other hand, 3D convolution wastes a large amount of memory on mostly unoccupied 3D space, which consists of only the surface visible to the sensor. Instead, we propose SurfConv, which "slides" compact 2D filters along the visible 3D surface. SurfConv is formulated as a simple depth-aware multi-scale 2D convolution, through a new Data-Driven Depth Discretization (D4) scheme. We demonstrate the effectiveness of our method on indoor and outdoor 3D semantic segmentation datasets. Our method achieves state-of-the-art performance with less than 30% parameters used by the 3D convolution-based approaches.
更多查看译文
关键词
convolutional neural networks,RGB input,scale variance problem,image convolution based approaches,SurfConv,Data-Driven Depth Discretization scheme,3D convolution based approaches,depth-aware multiscale 2D convolution,3D semantic segmentation datasets,2D filters
AI 理解论文
溯源树
样例
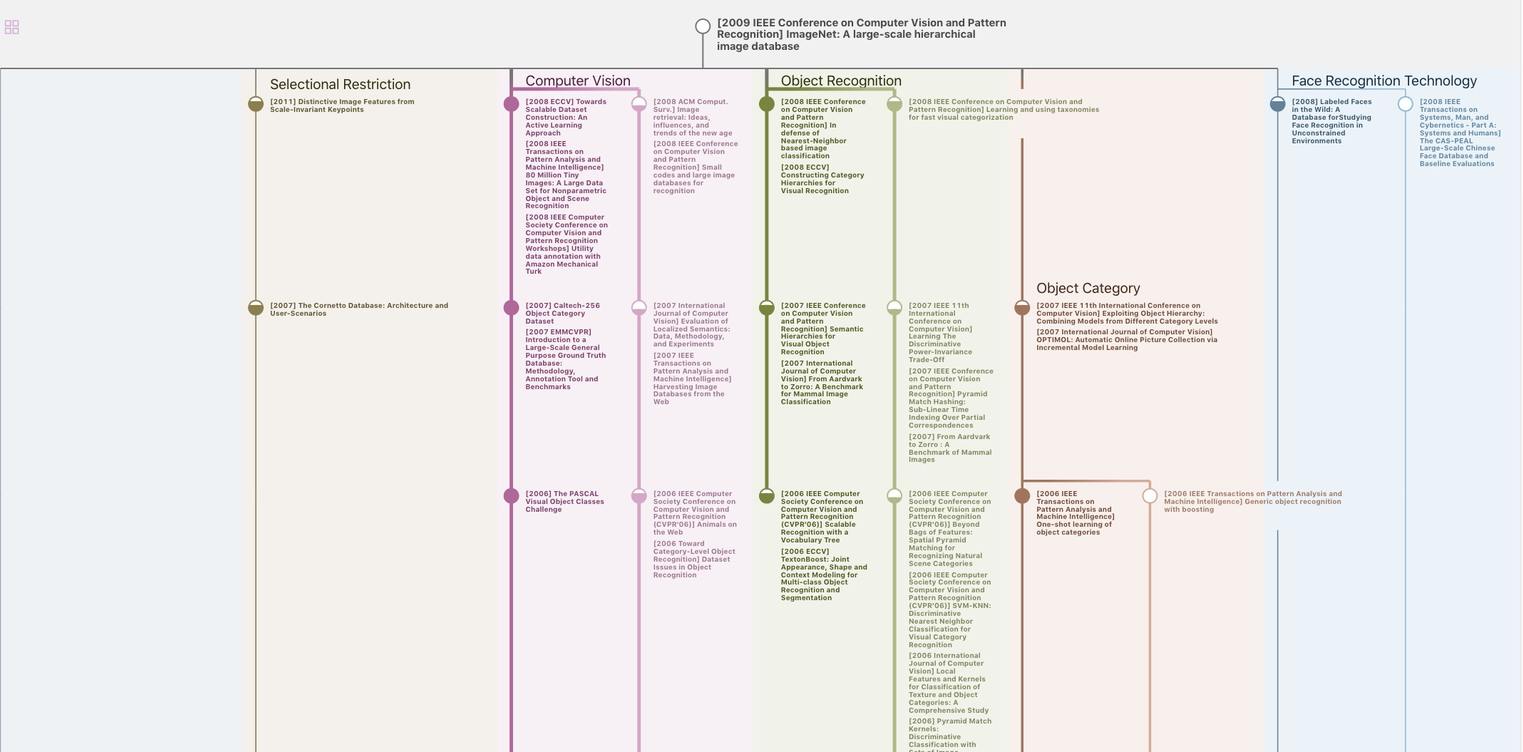
生成溯源树,研究论文发展脉络
Chat Paper
正在生成论文摘要