NU-Net: Deep Residual Wide Field of View Convolutional Neural Network for Semantic Segmentation.
IEEE Computer Society Conference on Computer Vision and Pattern Recognition Workshops(2018)
摘要
Semantic Segmentation of satellite images is one of the most challenging problems in computer vision as it requires a model capable of capturing both local and global information at each pixel. Current state of the art methods are based on Fully Convolutional Neural Networks (FCNN) with mostly two main components: an encoder which is a pretrained classification model that gradually reduces the input spatial size and a decoder that transforms the encoder's feature map into a predicted mask with the original size. We change this conventional architecture to a model that makes use of full resolution information. NU-Net is a deep FCNN that is able to capture wide field of view global information around each pixel while maintaining localized full resolution information throughout the model. We evaluate our model on the Land Cover Classification and Road Extraction tracks in the DeepGlobe competition.
更多查看译文
关键词
NU-Net,semantic segmentation,satellite images,computer vision,local information,encoder,pretrained classification model,input spatial size,predicted mask,conventional architecture,resolution information,deep FCNN,fully convolutional neural networks,land cover classification,deep residual wide field-of-view convolutional neural network,wide field-of-view global information,road extraction tracks,DeepGlobe competition
AI 理解论文
溯源树
样例
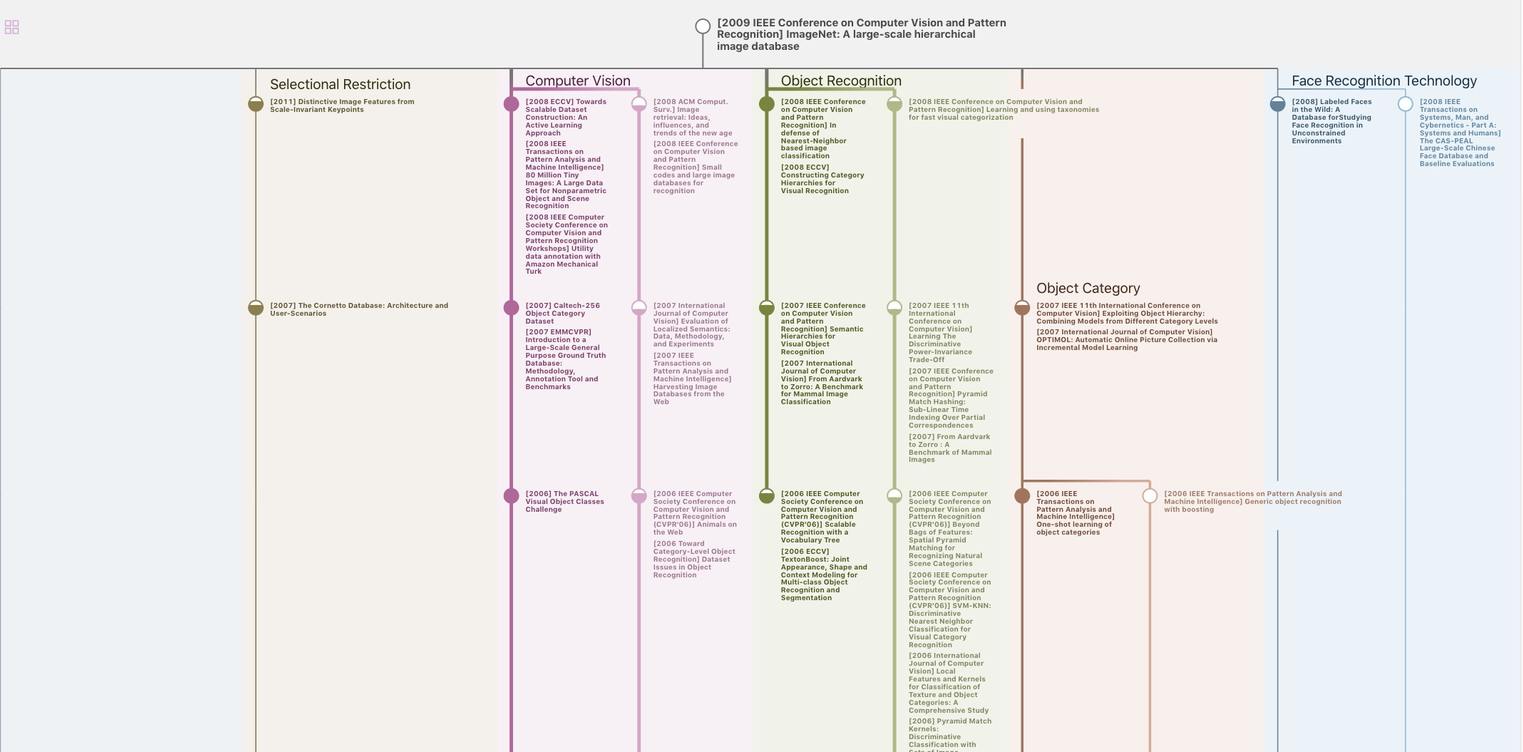
生成溯源树,研究论文发展脉络
Chat Paper
正在生成论文摘要