On The Importance Of Label Quality For Semantic Segmentation
2018 IEEE/CVF CONFERENCE ON COMPUTER VISION AND PATTERN RECOGNITION (CVPR)(2018)
摘要
Convolutional networks (ConvNets) have become the dominant approach to semantic image segmentation. Producing accurate, pixel level labels required for this task is a tedious and time consuming process; howevet; producing approximate. coarse labels could take only a fraction of the time and clbrt. We investigate the relationship between the quality of labels and the performance of ConvNets for semantic segmentation. We create a very large synthetic dataset with perfectly labeled street view scenes. From these perfect labels, we synthetically coarsen labels with different qualities and estimate human hours required for producing them. We peiform a series of experiments by training ConvNets with a varying number of training images and label qualify. We found that the performance of ConvNets mostly depends on the time spent creating the training labels. That is, a larger coarsely annotated dataset can yield the same performance as a smaller finely annotated one. Furthermore. fine tuning coarsely pre trained ConvNets with few finely-annotated labels can yield comparable or superior performance to training it with a large amount of finely annotated labels alone, at a fraction of the labeling cost. We demonstrate that our result is also valid for different network architectures, and various object classes in an urban scene.
更多查看译文
关键词
label quality,convolutional networks,semantic image segmentation,pixel-level labels,coarsely-annotated dataset,finely-annotated labels,labeling cost,ConvNets training
AI 理解论文
溯源树
样例
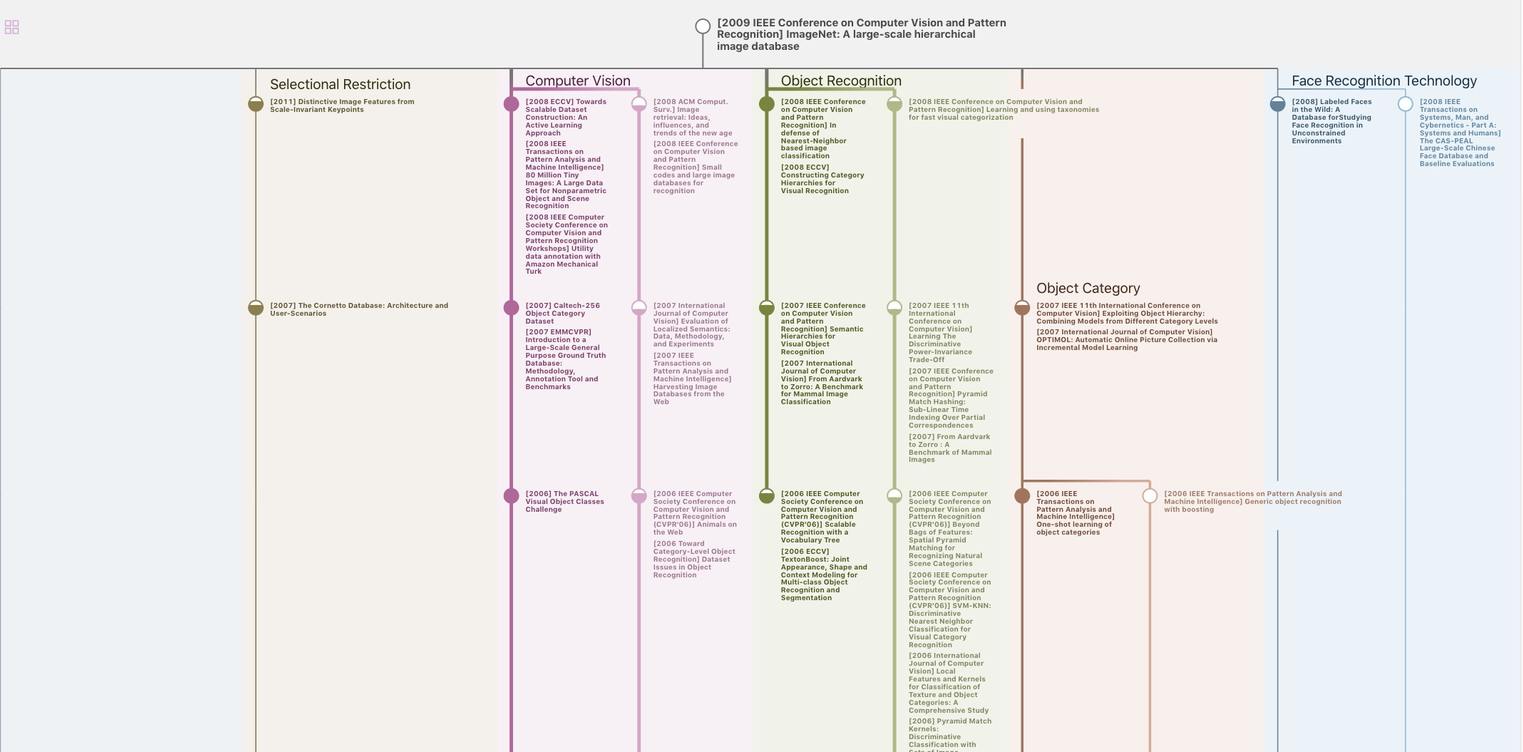
生成溯源树,研究论文发展脉络
Chat Paper
正在生成论文摘要