Making Convolutional Networks Recurrent For Visual Sequence Learning
2018 IEEE/CVF CONFERENCE ON COMPUTER VISION AND PATTERN RECOGNITION (CVPR)(2018)
摘要
Recurrent neural networks (RNNs) have emerged as a powerful model for a broad range of machine learning problems that involve sequential data. While an abundance of work exists to understand and improve RNNs in the context of language and audio signals such as language modeling and speech recognition, relatively little attention has been paid to analyze or modify RNNs for visual sequences, which by nature have distinct properties. In this paper, we aim to bridge this gap and present the first large-scale exploration of RNNs for visual sequence learning. In particular, with the intention of leveraging the strong generalization capacity of pre-trained convolutional neural networks (CNNs), we propose a novel and effective approach, Pre-RNN, to make pre-trained CNNs recurrent by transforming convolutional layers or fully connected layers into recurrent layers. We conduct extensive evaluations on three representative visual sequence learning tasks: sequential face alignment, dynamic hand gesture recognition, and action recognition. Our experiments reveal that PreRNN consistently outperforms the traditional RNNs and achieves stateof- the-art results on the three applications, suggesting that PreRNN is more suitable for visual sequence learning.
更多查看译文
关键词
recurrent neural networks,machine learning problems,speech recognition,pre-trained convolutional neural networks,CNNs,visual sequence learning tasks,audio signals,PreRNN,sequential face alignment,dynamic hand gesture recognition,action recognition
AI 理解论文
溯源树
样例
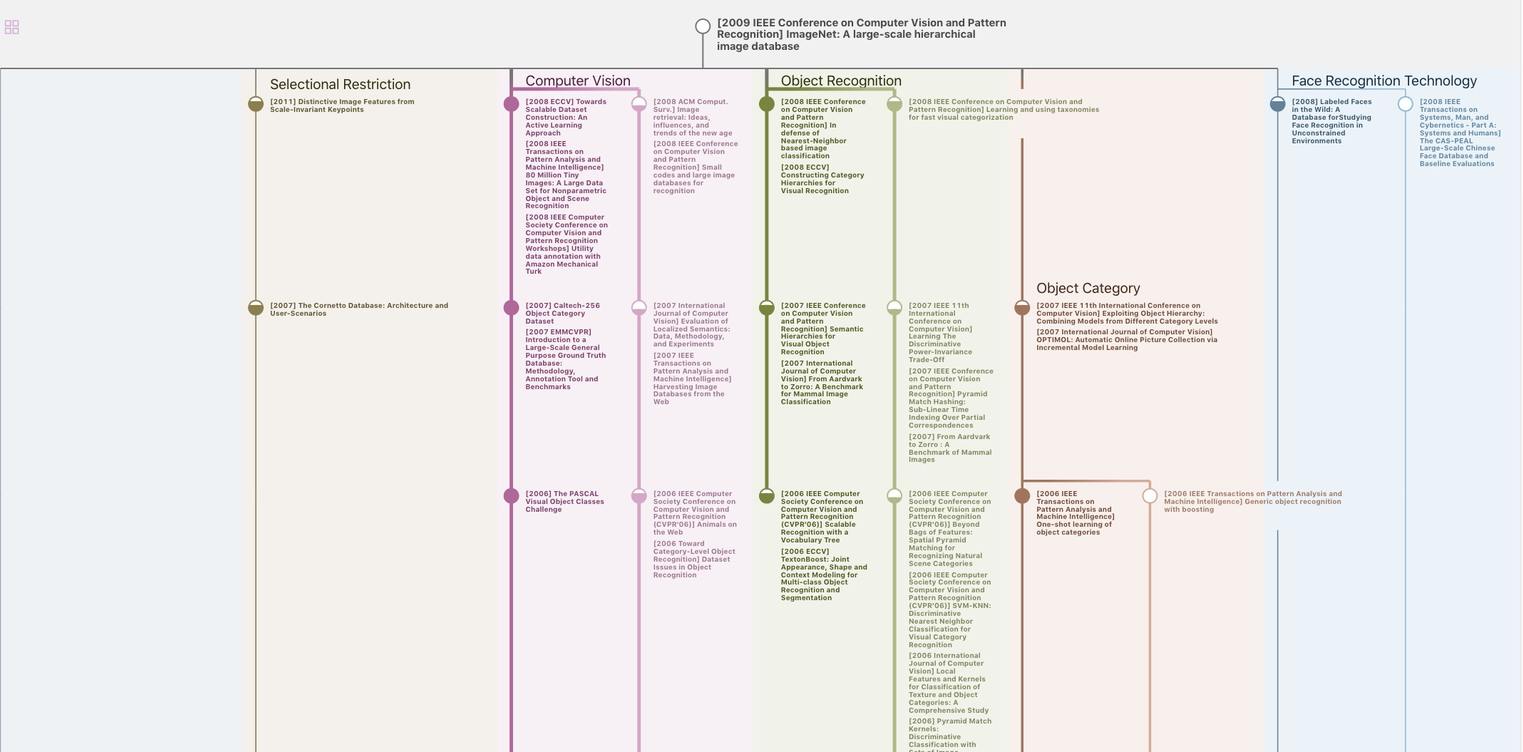
生成溯源树,研究论文发展脉络
Chat Paper
正在生成论文摘要