Trust Your Model: Light Field Depth Estimation With Inline Occlusion Handling
2018 IEEE/CVF CONFERENCE ON COMPUTER VISION AND PATTERN RECOGNITION (CVPR)(2018)
摘要
We address the problem of depth estimation from light field images. Our main contribution is a new way to handle occlusions which improves general accuracy and quality of object borders. In contrast to all prior work we work with a model which directly incorporates both depth and occlusion, using a local optimization scheme based on the PatchMatch algorithm. The key benefit of this joint approach is that we utilize all available data, and not erroneously discard valuable information in pre-processing steps. We see the benefit of our approach not only at improved object boundaries, but also at smooth surface reconstruction, where we outperform even methods which focus on good surface regularization. We have evaluated our method on a public light field dataset, where we achieve state-of-the-art results in nine out of twelve error metrics, with a close tie for the remaining three.
更多查看译文
关键词
smooth surface reconstruction,light field depth estimation,inline occlusion handling,light-field images,PatchMatch algorithm,object boundaries,surface regularization,object quality,optimization scheme
AI 理解论文
溯源树
样例
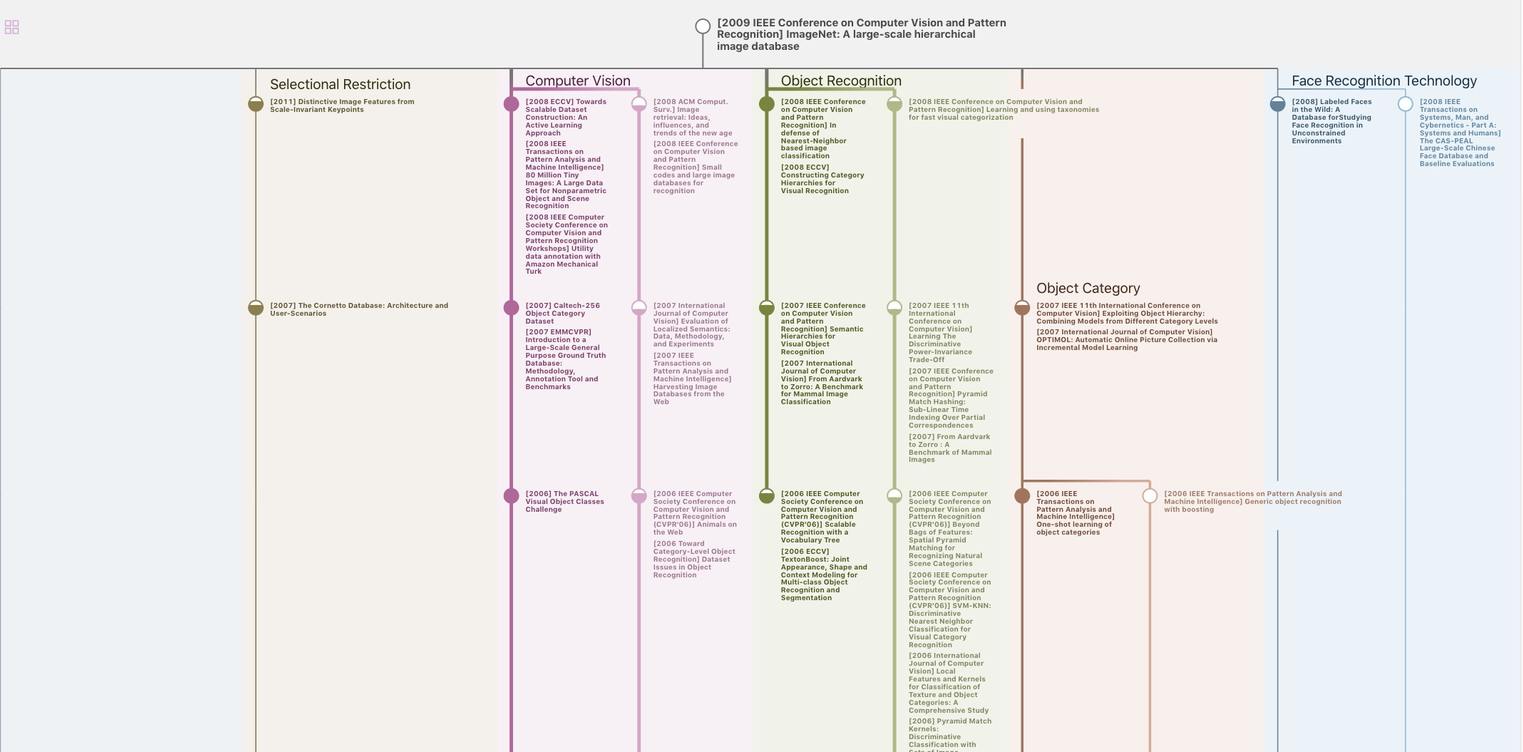
生成溯源树,研究论文发展脉络
Chat Paper
正在生成论文摘要