Hydranets: Specialized Dynamic Architectures For Efficient Inference
2018 IEEE/CVF CONFERENCE ON COMPUTER VISION AND PATTERN RECOGNITION (CVPR)(2018)
摘要
There is growing interest in improving the design of deep network architectures to be both accurate and low cost. This paper explores semantic specialization as a mechanism for improving the computational efficiency (accuracy per -unit-cost) of inference in the context of image classification. Specifically, we propose a network architecture template called HydraNet, which enables state-of-the-art architectures for image classification to be transformed into dynamic architectures which exploit conditional execution for efficient inference. HydraNets are wide networks containing distinct components specialized to compute features for visually similar classes, but they retain efficiency by dynamically selecting only a small number of components to evaluate for any one input image. This design is made possible by a soft gating mechanism that encourages component specialization during training and accurately performs component selection during inference. We evaluate the HydraNet approach on both the CIFAR-100 and ImageNet classification tasks. On CIFAR, applying the HydraNet template to the ResNet and DenseNet family of models reduces inference cost by 2-4x while retaining the accuracy of the baseline architectures. On ImageNet, applying the HydraNet template improves accuracy up to 2.5% when compared to an efficient baseline architecture with similar inference cost.
更多查看译文
关键词
deep network architectures,image classification,network architecture template,soft gating mechanism,component selection,CIFAR-100,HydraNet template,ImageNet classification,deep neural networks
AI 理解论文
溯源树
样例
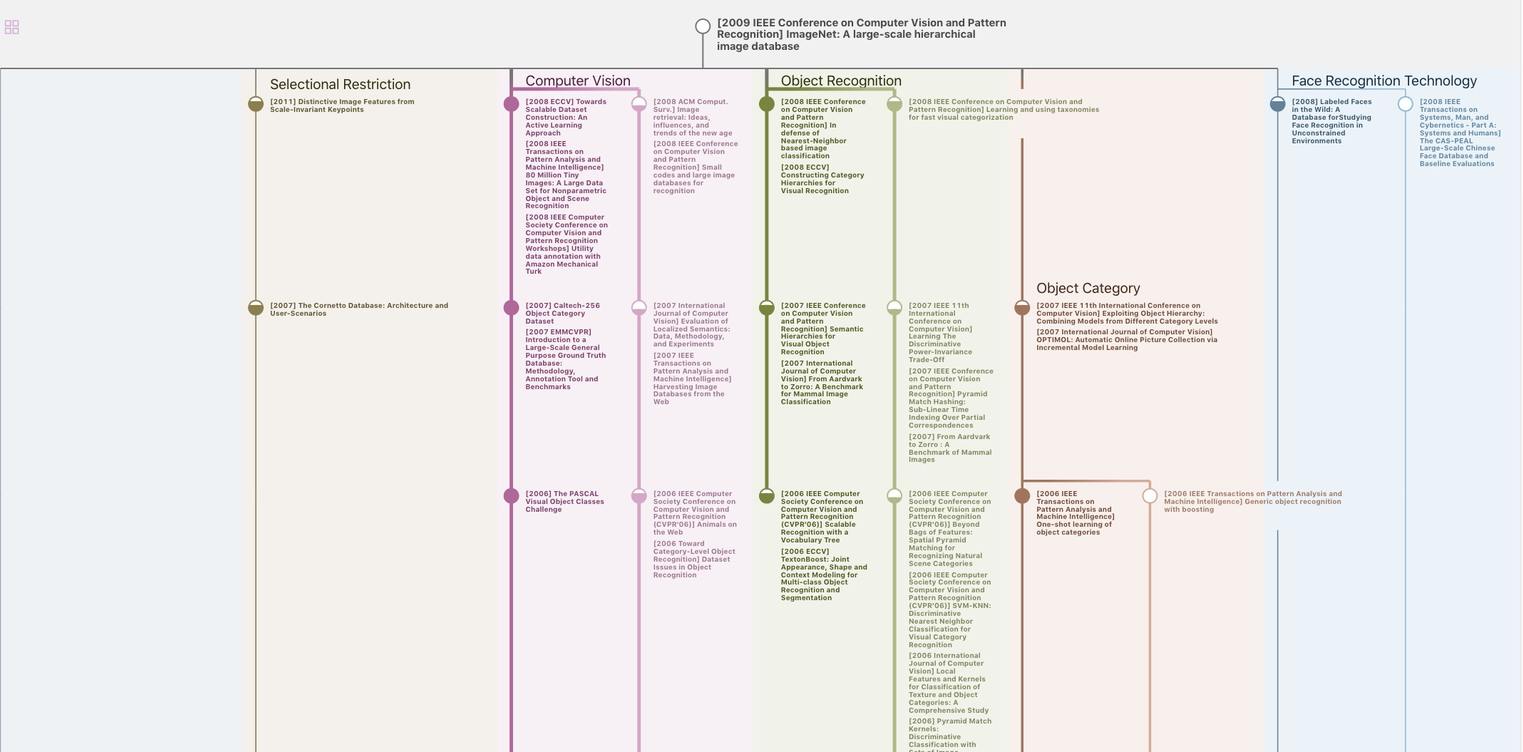
生成溯源树,研究论文发展脉络
Chat Paper
正在生成论文摘要