Heat Map Based Feature Ranker: In Depth Comparison With Popular Methods
INTELLIGENT DATA ANALYSIS(2018)
摘要
The new era of technology allows us to gather more data than ever before, complex data emerge and a lot of noise can be found among high dimensional datasets. In order to discard useless features and help build more generalized models, feature selection seeks a reduced subset of features that improve the performance of the learning algorithm. The evaluation of features and their interactions are an expensive process, hence the need for heuristics. In this work, we present Heat Map Based Feature Ranker, an algorithm to estimate feature importance purely based on its interaction with other variables. A compression mechanism reduces evaluation space up to 66% without compromising efficacy. Our experiments show that our proposal is very competitive against popular algorithms, producing stable results across different types of data. We also show how noise reduction through feature selection aids data visualization using emergent self-organizing maps.
更多查看译文
关键词
Feature selection, high-dimensional data, self-organizing maps
AI 理解论文
溯源树
样例
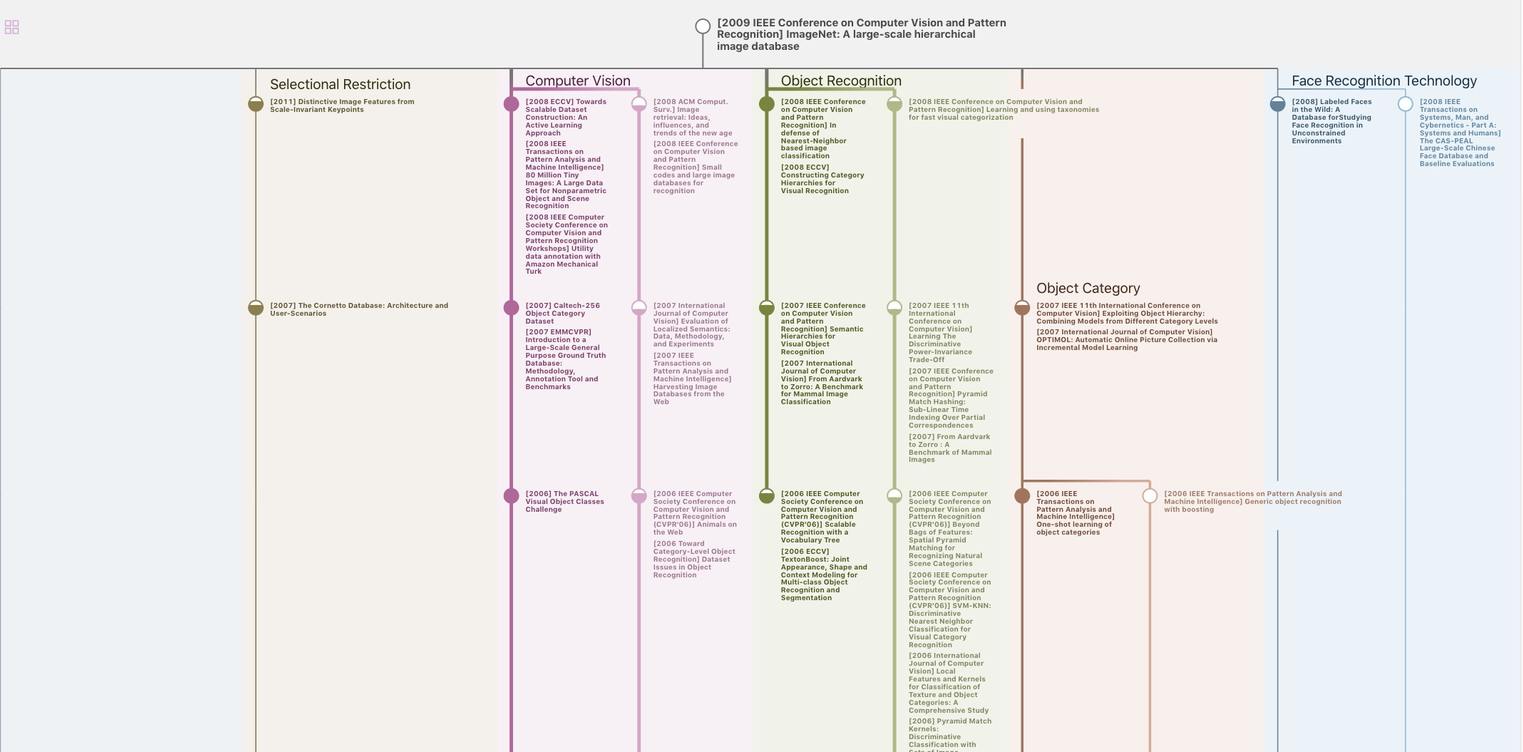
生成溯源树,研究论文发展脉络
Chat Paper
正在生成论文摘要