Deep neural network based i-vector mapping for speaker verification using short utterances.
Speech Communication(2018)
摘要
Text-independent speaker recognition using short utterances is a highly challenging task due to the large variation and content mismatch between short utterances. I-vector and probabilistic linear discriminant analysis (PLDA) based systems have become the standard in speaker verification applications, but they are less effective with short utterances. In this paper, we first compare two state-of-the-art universal background model (UBM) training methods for i-vector modeling using full-length and short utterance evaluation tasks. The two methods are Gaussian mixture model (GMM) based (denoted I-vector_GMM) and deep neural network (DNN) based (denoted as I-vector_DNN) methods. The results indicate that the I-vector_DNN system outperforms the I-vector_GMM system under various durations (from full length to 5 s). However, the performances of both systems degrade significantly as the duration of the utterances decreases. To address this issue, we propose two novel nonlinear mapping methods which train DNN models to map the i-vectors extracted from short utterances to their corresponding long-utterance i-vectors. The mapped i-vector can restore missing information and reduce the variance of the original short-utterance i-vectors. The proposed methods both model the joint representation of short and long utterance i-vectors: the first method trains an autoencoder first using concatenated short and long utterance i-vectors and then uses the pre-trained weights to initialize a supervised regression model from the short to long version; the second method jointly trains the supervised regression model with an autoencoder reconstructing the short utterance i-vector itself. Experimental results using the NIST SRE 2010 dataset show that both methods provide significant improvement and result in a 24.51% relative improvement in Equal Error Rates (EERs) from a baseline system. In order to learn a better joint representation, we further investigate the effect of a deep encoder with residual blocks, and the results indicate that the residual network can further improve the EERs of a baseline system by up to 26.47%. Moreover, in order to improve the short i-vector mapping to its long version, an additional vector, which represents the average value of phoneme posteriors across frames, is also added to the input, and results in a 28.43% improvement. When further testing the best-validated models of SRE10 on the Speaker In The Wild (SITW) dataset, the methods result in a 23.12% improvement on arbitrary-duration (1–5 s) short-utterance conditions.
更多查看译文
关键词
Speaker verification,Short utterances,I-vectors,DNNs,Nonlinear mapping,Joint modeling,Autoencoder
AI 理解论文
溯源树
样例
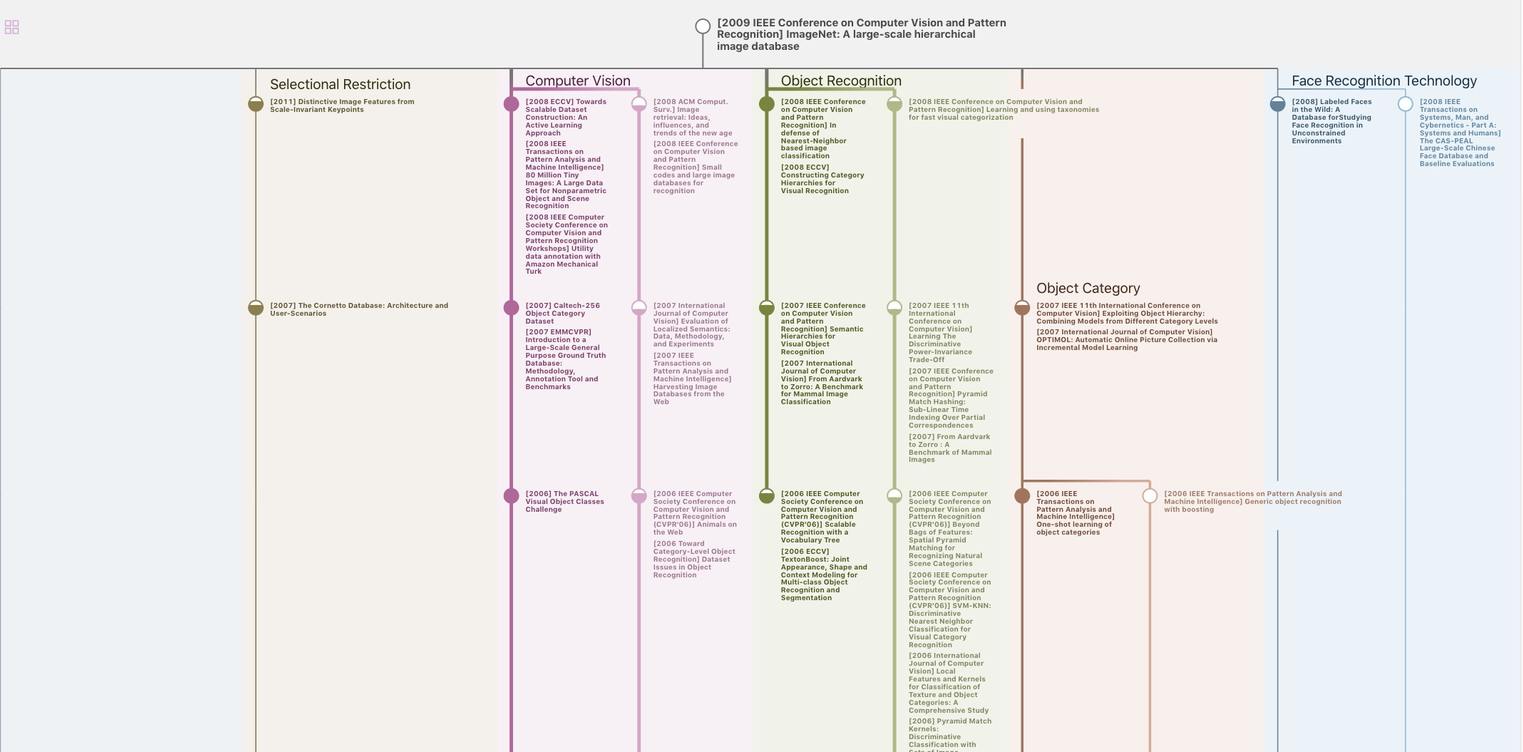
生成溯源树,研究论文发展脉络
Chat Paper
正在生成论文摘要