Improved robustness to adversarial examples using Lipschitz regularization of the loss.
arXiv: Learning(2018)
摘要
Adversarial training is an effective method for improving robustness to adversarial attacks. We show that adversarial training using the Fast Signed Gradient Method can be interpreted as a form of regularization. We implemented a more effective form of adversarial training, which in turn can be interpreted as regularization of the loss in the 2-norm, $|nabla_x ell(x)|_2$. We obtained further improvements to adversarial robustness, as well as provable robustness guarantees, by augmenting adversarial training with Lipschitz regularization.
更多查看译文
AI 理解论文
溯源树
样例
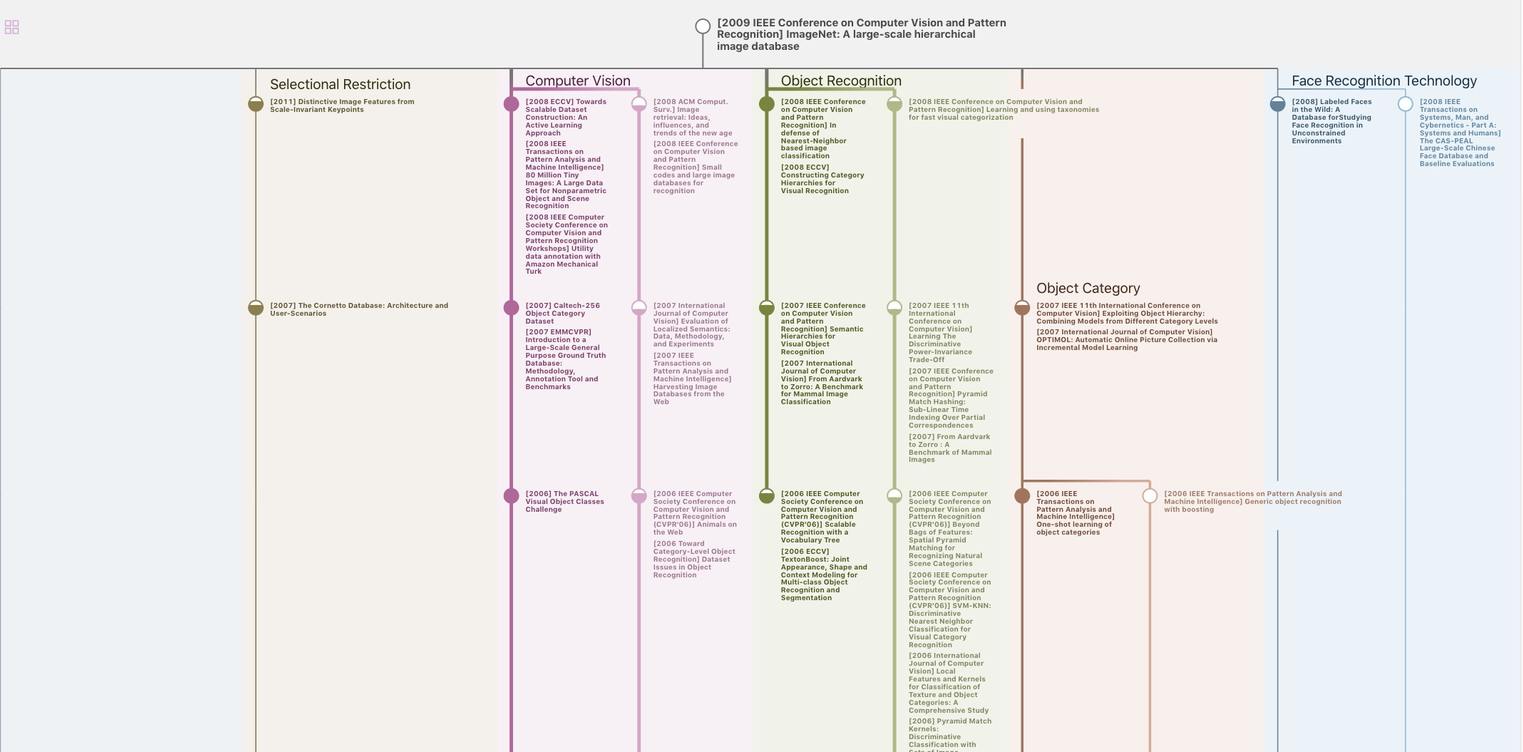
生成溯源树,研究论文发展脉络
Chat Paper
正在生成论文摘要