Covariate Gaussian Process Latent Variable Models.
arXiv (Cornell University)(2018)
摘要
Gaussian Process Regression (GPR) and Gaussian Process Latent Variable Models (GPLVM) offer a principled way of performing probabilistic non-linear regression and dimensionality reduction. In this paper we propose a hybrid between the two, the covariate-GPLVM (c-GPLVM), to perform dimensionality reduction in the presence of covariate information (e.g. continuous covariates, class labels, or censored survival times). This construction lets us adjust for covariate effects and reveals meaningful latent structure which is not revealed when using GPLVM. Furthermore, we introduce structured decomposable kernels which will let us interpret how the fixed and latent inputs contribute to feature-level variation, e.g. identify the presence of a non-linear interaction. We demonstrate the utility of this model on applications in disease progression modelling from high-dimensional gene expression data in the presence of additional phenotypes.
更多查看译文
关键词
models,process
AI 理解论文
溯源树
样例
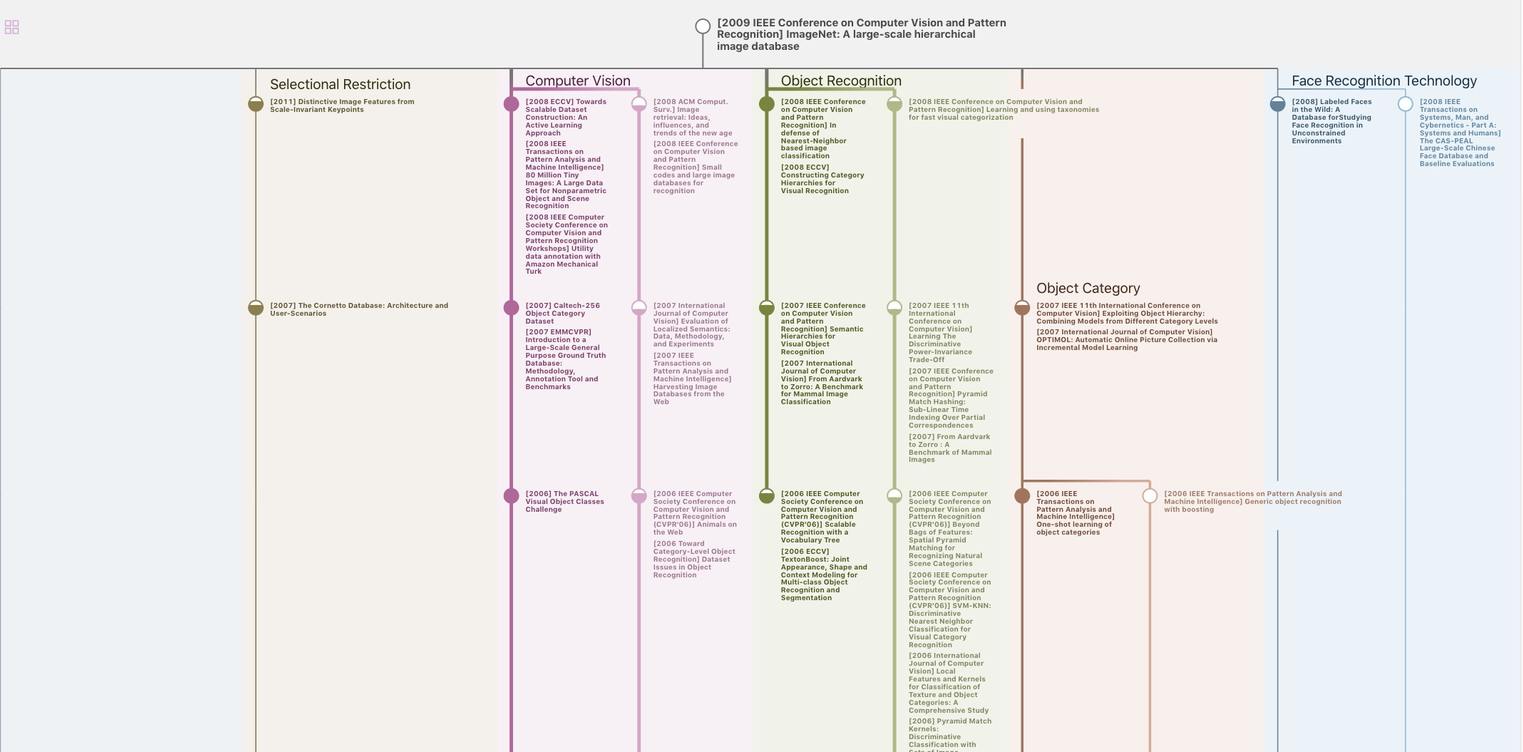
生成溯源树,研究论文发展脉络
Chat Paper
正在生成论文摘要