The Computational Complexity of Training ReLU(s).
arXiv: Computational Complexity(2018)
摘要
We consider the computational complexity of training depth-2 neural networks composed of rectified linear units (ReLUs). We show that, even for the case of a single ReLU, finding a set of weights that minimizes the squared error (even approximately) for a given training set is NP-hard. We also show that for a simple network consisting of two ReLUs, the error minimization problem is NP-hard, even in the realizable case. We complement these hardness results by showing that, when the weights and samples belong to the unit ball, one can (agnostically) properly and reliably learn depth-2 ReLUs with $k$ units and error at most $epsilon$ in time $2^{(k/epsilon)^{O(1)}}n^{O(1)}$; this extends upon a previous work of Goel, Kanade, Klivans and Thaler (2017) which provided efficient improper learning algorithms for ReLUs.
更多查看译文
AI 理解论文
溯源树
样例
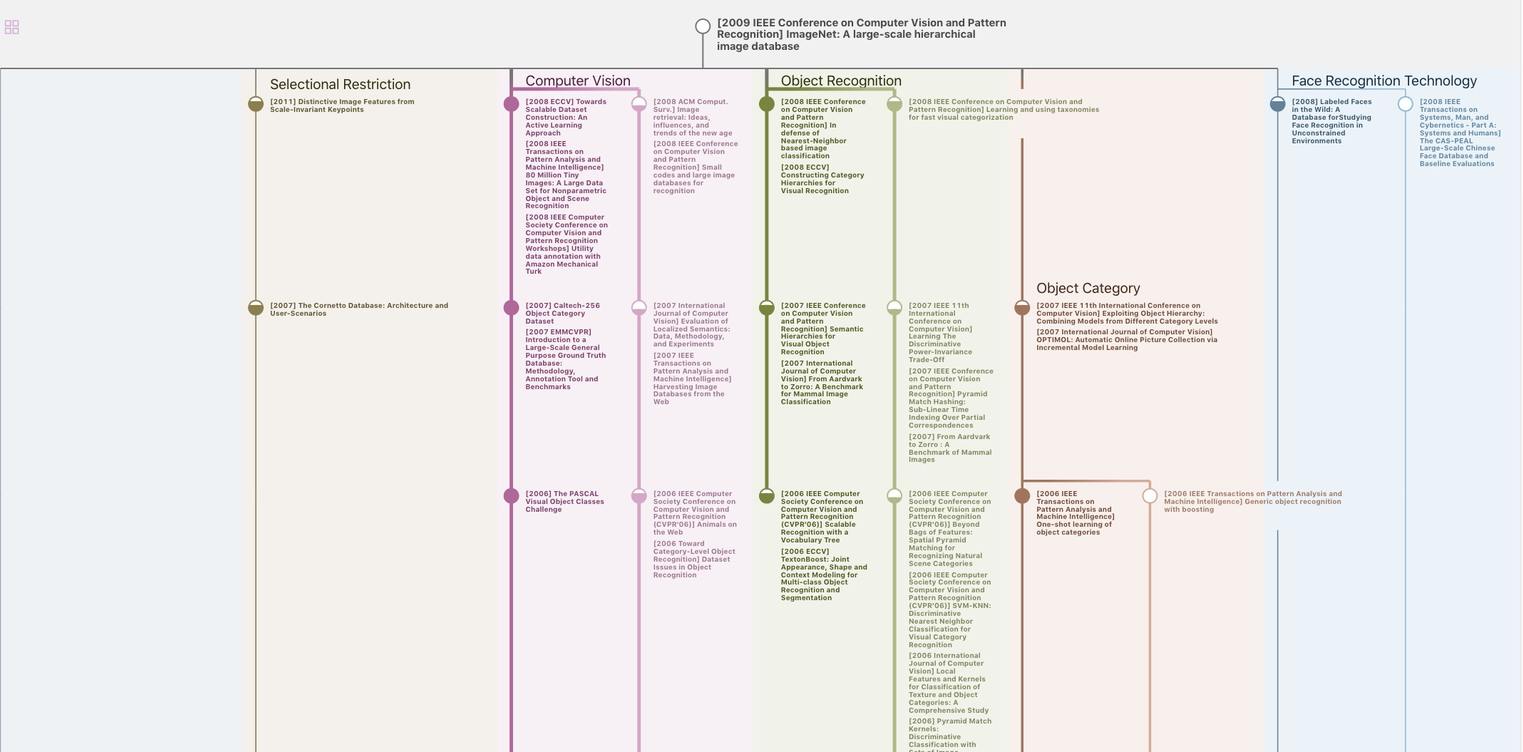
生成溯源树,研究论文发展脉络
Chat Paper
正在生成论文摘要