Deep Attentive Tracking Via Reciprocative Learning
ADVANCES IN NEURAL INFORMATION PROCESSING SYSTEMS 31 (NIPS 2018)(2018)
摘要
Visual attention, derived from cognitive neuroscience, facilitates human perception on the most pertinent subset of the sensory data. Recently, significant efforts have been made to exploit attention schemes to advance computer vision systems. For visual tracking, it is often challenging to track target objects undergoing large appearance changes. Attention maps facilitate visual tracking by selectively paying attention to temporal robust features. Existing tracking-by-detection approaches mainly use additional attention modules to generate feature weights as the classifiers are not equipped with such mechanisms. In this paper, we propose a reciprocative learning algorithm to exploit visual attention for training deep classifiers. The proposed algorithm consists of feed-forward and backward operations to generate attention maps, which serve as regularization terms coupled with the original classification loss function for training The deep classifier learns to attend to the regions of target objects robust to appearance changes. Extensive experiments on large-scale benchmark datasets show that the proposed attentive tracking method performs favorably against the state-of-the-art approaches.
更多查看译文
关键词
learning algorithm,visual tracking,human perception,cognitive neuroscience,visual attention,computer vision systems
AI 理解论文
溯源树
样例
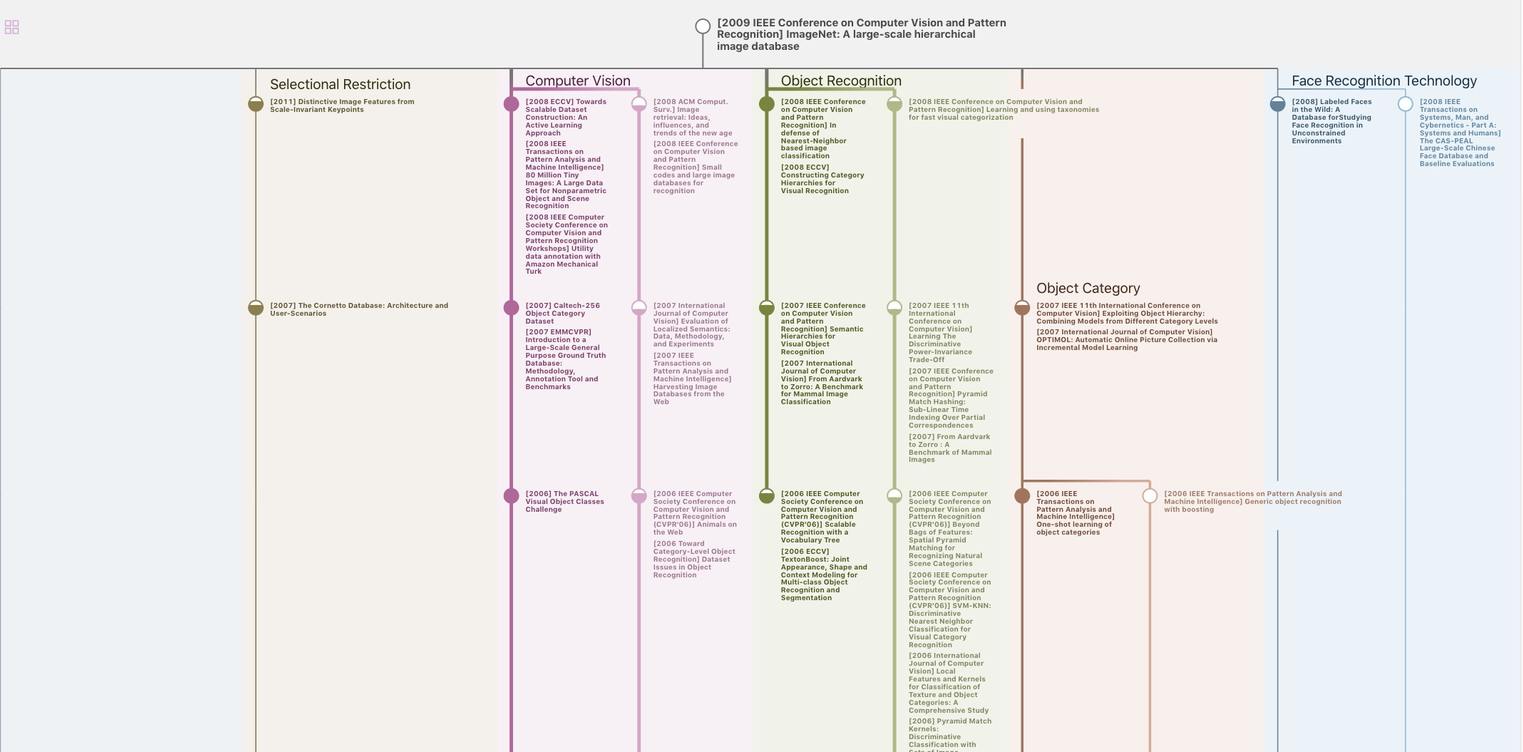
生成溯源树,研究论文发展脉络
Chat Paper
正在生成论文摘要