Deep Micro-Dictionary Learning and Coding Network.
2019 IEEE Winter Conference on Applications of Computer Vision (WACV)(2019)
摘要
In this paper, we propose a novel Deep Micro-Dictionary Learning and Coding Network (DDLCN). DDLCN has most of the standard deep learning layers (pooling, fully, connected, input/output, etc.) but the main difference is that the fundamental convolutional layers are replaced by novel compound dictionary learning and coding layers. The dictionary learning layer learns an over-complete dictionary for the input training data. At the deep coding layer, a locality constraint is added to guarantee that the activated dictionary bases are close to each other. Next, the activated dictionary atoms are assembled together and passed to the next compound dictionary learning and coding layers. In this way, the activated atoms in the first layer can be represented by the deeper atoms in the second dictionary. Intuitively, the second dictionary is designed to learn the fine-grained components which are shared among the input dictionary atoms. In this way, a more informative and discriminative low-level representation of the dictionary atoms can be obtained. We empirically compare the proposed DDLCN with several dictionary learning methods and deep learning architectures. The experimental results on four popular benchmark datasets demonstrate that the proposed DDLCN achieves competitive results compared with state-of-the-art approaches.
更多查看译文
关键词
Encoding,Dictionaries,Atomic layer deposition,Feature extraction,Deep learning,Compounds
AI 理解论文
溯源树
样例
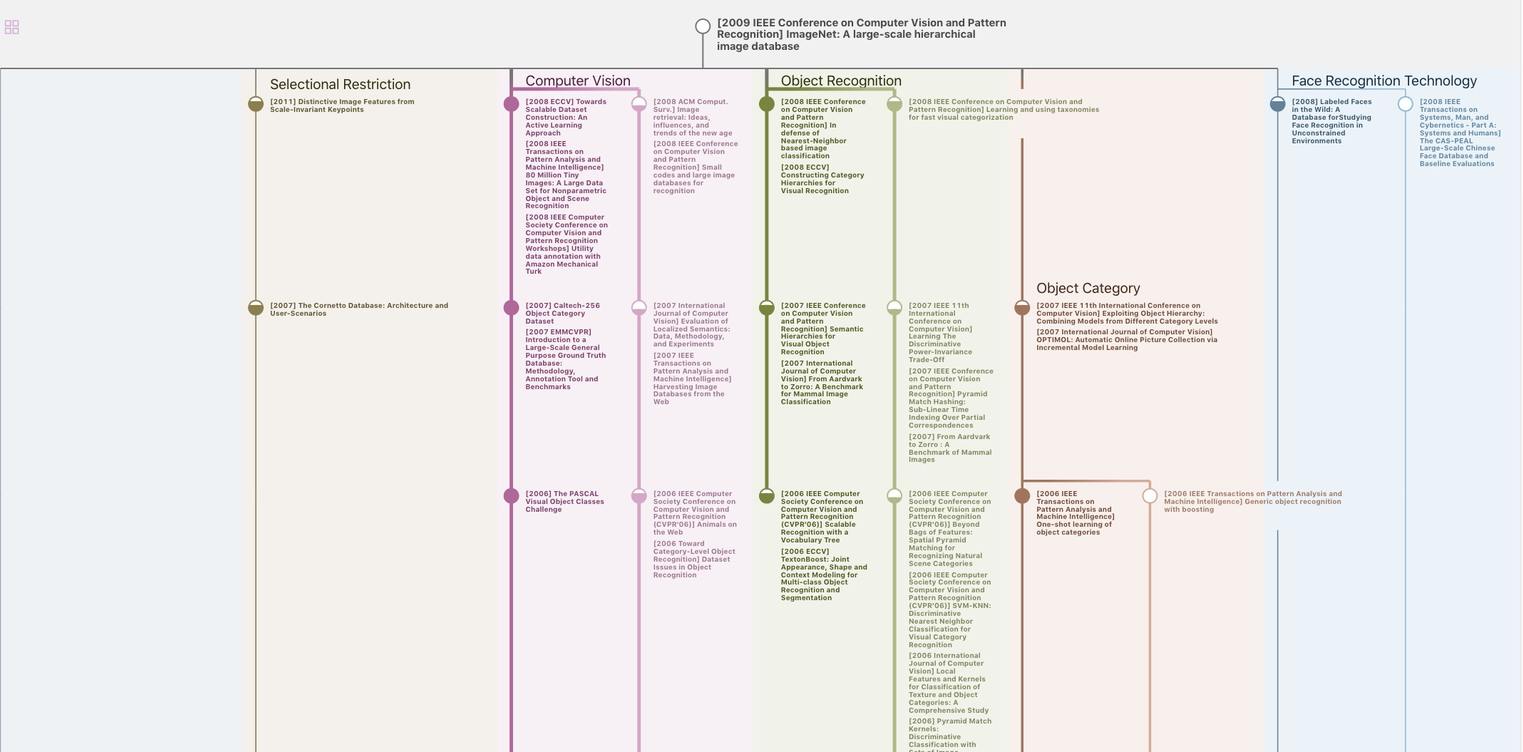
生成溯源树,研究论文发展脉络
Chat Paper
正在生成论文摘要